Parallel EM optimization using improved pole-residue-based neuro-TF surrogate and isomorphic orthogonal DOE sampling for microwave components design
INTERNATIONAL JOURNAL OF NUMERICAL MODELLING-ELECTRONIC NETWORKS DEVICES AND FIELDS(2024)
摘要
Direct electromagnetic (EM) optimization for microwave components design is usually a time-consuming process. To improve the optimization efficiency, this paper proposes a novel parallel EM optimization technique exploiting improved pole-residue-based neuro-transfer function (neuro-TF) surrogate and isomorphic orthogonal design of experiment (DOE) sampling strategy. We propose a new sampling method combining isomorphic orthogonal DOE and parallel EM simulations to generate training data for developing the neuro-TF surrogate. This proposed sampling method can ensure the scattered distribution of data samples in the overall optimization process, thus effectively improving the surrogate accuracy and increasing the optimization speed. We also propose a new pole-residue tracking technique for order-changing to solve the discontinuity problem of pole/residues during the neuro-TF surrogate development. Different from the fixed split ratio in existing pole-residue tracking technique, the split ratio of poles and residues in the proposed technique is adaptive and determined according to the information of neighboring samples. Therefore, the continuity and smoothness of pole/residues after the splitting are improved, so as the neuro-TF surrogate accuracy. In addition, the trust region algorithm is exploited during EM optimization to improve the convergence speed. In this way, the proposed EM optimization technique obtains the optimal solution in a shorter time with fewer iterations than the existing techniques. Two examples of EM optimizations of microwave components are used to illustrate the proposed technique.
更多查看译文
关键词
electromagnetic (EM) optimization,isomorphic orthogonal DOE,microwave components,neuro-transfer function (neuro-TF),order-changing,parallel computation
AI 理解论文
溯源树
样例
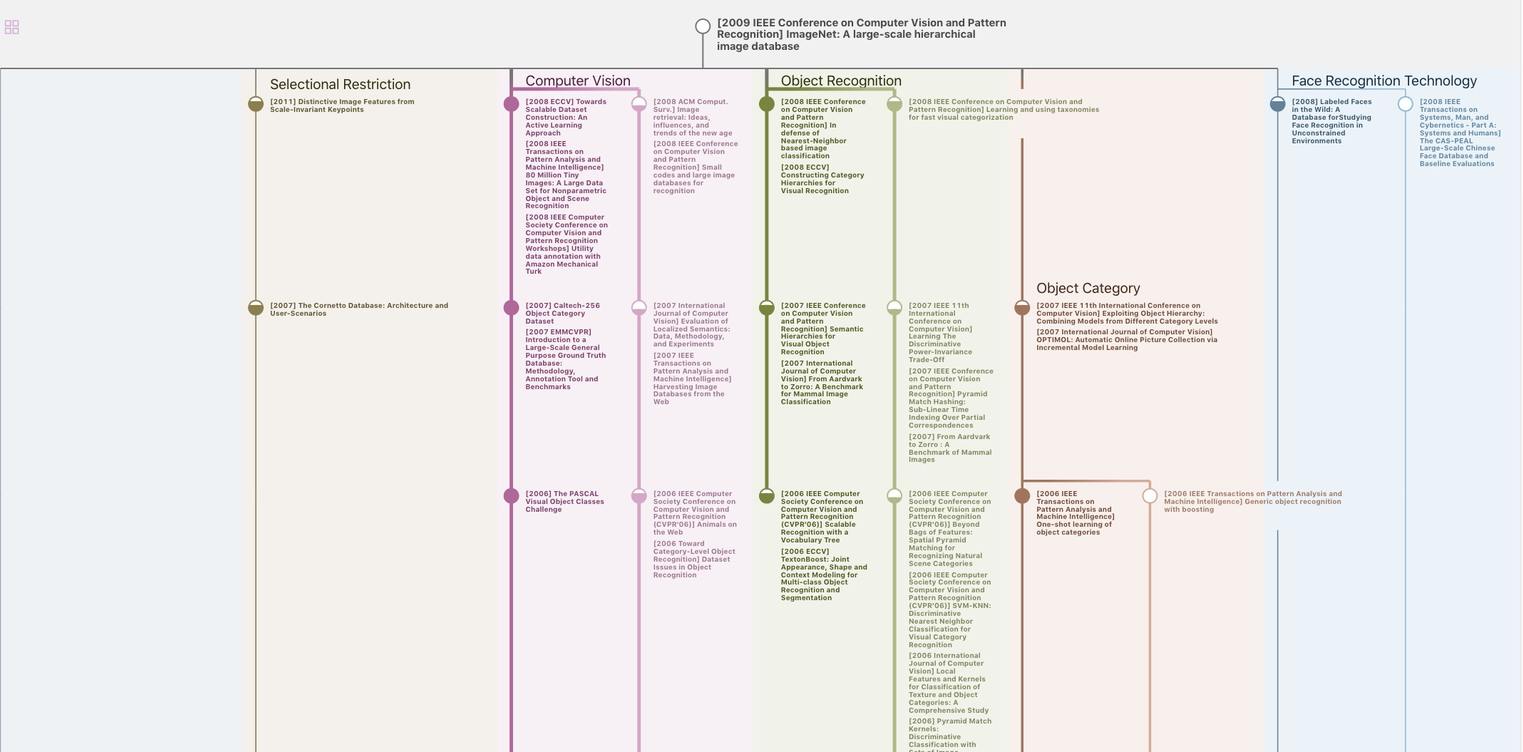
生成溯源树,研究论文发展脉络
Chat Paper
正在生成论文摘要