Node-of-Influence Network Analysis for Targeted Condition Sequencing in Plasma Chemical Reaction Networks
PLASMA CHEMISTRY AND PLASMA PROCESSING(2023)
摘要
It was demonstrated that a plasma chemical reaction system can be represented as a directed bipartite variable-relationship (VR) graph to accurately represent node influence, and that targeted node-influence analysis of this graph can determine improved condition sequencing for a target outcome. A novel graph algorithm (OCARINA) was adapted for VR-graphs to give a measure of the net-influence of incremental increase (NIII) of one variable on another at any depth in the graph. Additionally, two conventional node-influence measures, the Eigenvector Centrality Index (ECI) and Katz Centrality Index (KCI), were also trialled on the VR-graph. The electron energy (ε) node influence was evaluated on a “baseline” continuous sequence of 10 ns 1 eV pulses in a 0D chemical-kinetic simulation using ECI, KCI and OCARINA NIII at three depths. KCI appeared to give meaningful values for ε influence in the whole graph but not on specific nodes, ECI gave no meaningful results. OCARINA (O 3 targeted) suggested each successive ε pulse had diminishing influence on O 3 formation, though analysis differed for each NIII depth. O 3 concentrations in simulations with different numbers of ε pulses decreased with each additional pulse, correlating with the OCARINA analysis. O 3 , NO and O Species production in simulations of two ε pulses with one or both pulses incrementally changed by 10% from the baseline also largely agreed with the OCARINA results for each species on a baseline simulation of two consecutive 1 eV electron energy pulses. Additionally, it was found the NIII at antecedent depths corresponded to effects in subsequent phases in simulations.
更多查看译文
关键词
Plasma chemistry,Graph theory,Optimization,Targeting,Chemical engineering
AI 理解论文
溯源树
样例
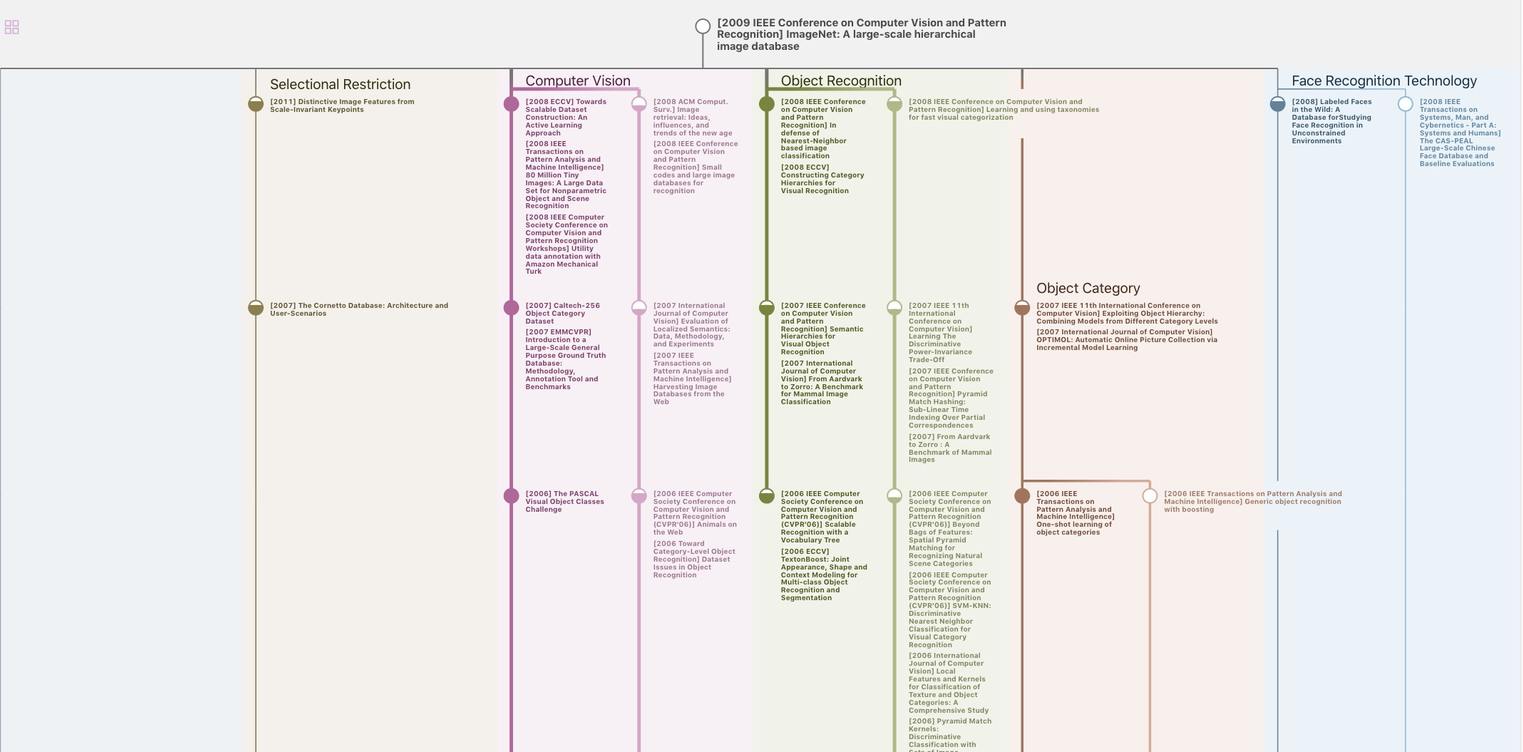
生成溯源树,研究论文发展脉络
Chat Paper
正在生成论文摘要