Underwater Image Enhancement via Triple-Branch Dense Block and Generative Adversarial Network
JOURNAL OF MARINE SCIENCE AND ENGINEERING(2023)
摘要
The complex underwater environment and light scattering effect lead to severe degradation problems in underwater images, such as color distortion, noise interference, and loss of details. However, the degradation problems of underwater images bring a significant challenge to underwater applications. To address the color distortion, noise interference, and loss of detail problems in underwater images, we propose a triple-branch dense block-based generative adversarial network (TDGAN) for the quality enhancement of underwater images. A residual triple-branch dense block is designed in the generator, which improves performance and feature extraction efficiency and retains more image details. A dual-branch discriminator network is also developed, which helps to capture more high-frequency information and guides the generator to use more global content and detailed features. Experimental results show that TDGAN is more competitive than many advanced methods from the perspective of visual perception and quantitative metrics. Many application tests illustrate that TDGAN can significantly improve the accuracy of underwater target detection, and it is also applicable in image segmentation and saliency detection.
更多查看译文
关键词
generative adversarial network (GAN),underwater image enhancement,multiscale dense,residual learning
AI 理解论文
溯源树
样例
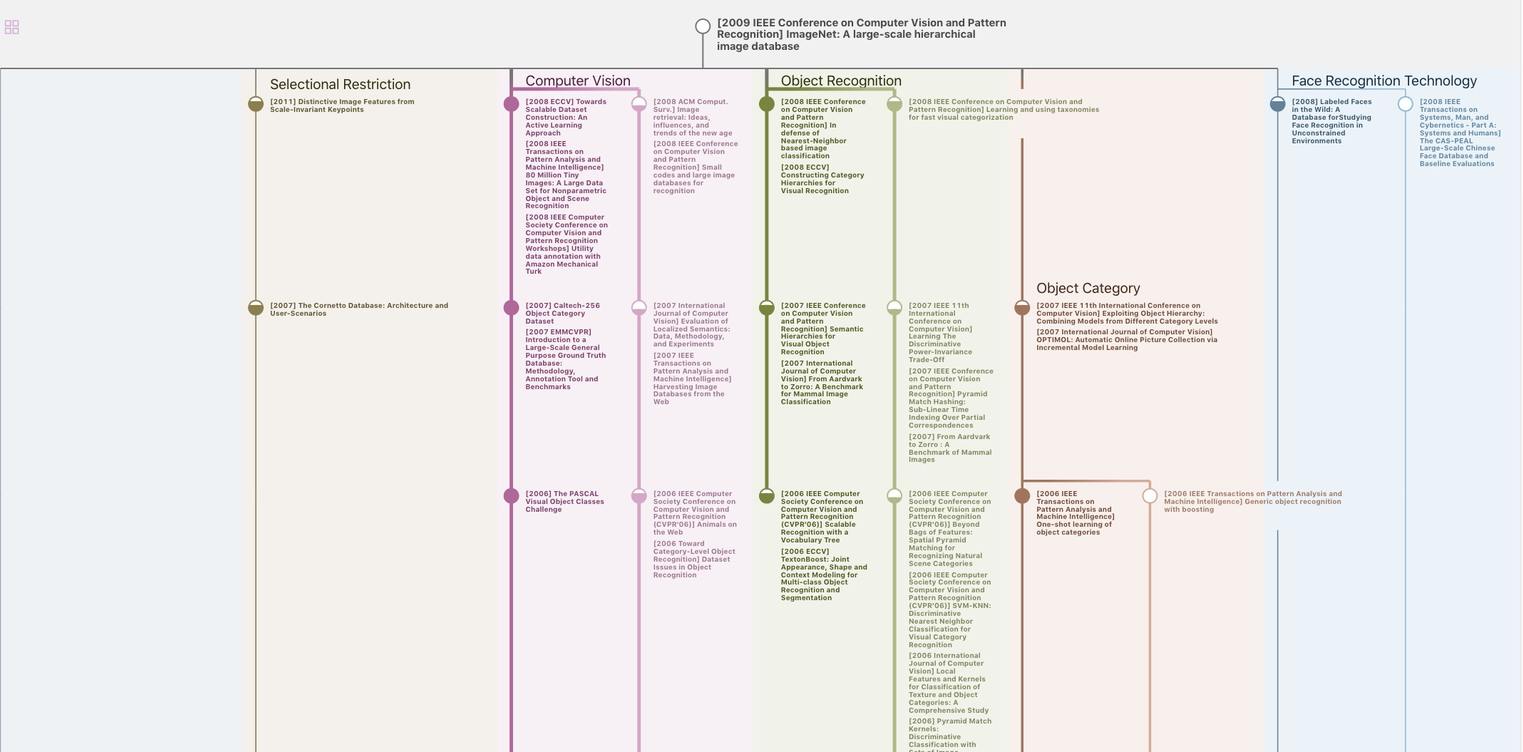
生成溯源树,研究论文发展脉络
Chat Paper
正在生成论文摘要