An Order-Invariant and Interpretable Dilated Convolution Neural Network for Chemical Process Fault Detection and Diagnosis
IEEE TRANSACTIONS ON AUTOMATION SCIENCE AND ENGINEERING(2023)
摘要
Although convolution neural network (CNN) has achieved certain success in fault detection and diagnosis (FDD) tasks in the chemical engineering industry, the performance and credibility of CNN-based FDD methods are greatly limited by two factors. First, CNN relies upon strong temporal/spatial correlation in data, which is very difficult to obtain in generic chemical tabular data. Second, most CNN methods have poor interpretability due to the encapsulation mechanism of feature extraction, and thus there is great difficulty in identifying the root-cause features from the results obtained using these methods. To address these difficulties, we propose an order-invariant and interpretable dilated convolution neural network (OIDLCNN), which is composed of feature clustering, dilated convolution, and a deep Shapley additive explanations (SHAP) explainer. Specifically, the feature clustering technique is adopted to reorder the features thus those with strong correlations are placed to be adjacent to each other. The large receptive field of dilated convolution can capture long-range correlations so then it can further recover the feature correlations and improve the classification performance. Last but not least, the proposed method provides interpretability by including the SHAP values to quantify feature contribution and identify the root-cause feature as the one with the highest contribution. Computational experiments are conducted on the Tennessee Eastman chemical process benchmark dataset. Compared with the other state-of-the-art methods, the proposed OIDLCNN-SHAP method achieves better performance in capturing feature correlations, detecting faults, and identifying the root-cause features. Note to Practitioners-Fault detection and diagnosis (FDD) is significant for reducing maintenance costs and improving safety in chemical processes. In this paper, we investigate the difficulty in detecting faults and identifying the root-cause features in chemical multivariate processes. This work was motivated by the fact that the existing CNN-based FDD algorithms are not designed for generic chemical tabular data and fail to capture vital information about feature correlations. In addition, the commonly used bayesian network-based root cause analysis methods are expensive since they require much prior knowledge and expert rules. We present a novel framework to capture the unique feature correlations and obtain the root-cause features without any expert knowledge. The proposed method provides an automatic and low-cost way for chemical process FDD tasks. It can be further integrated into the condition monitoring system of real chemical processes to analyze potential faults and identify the corresponding root causes in real time.
更多查看译文
关键词
Index Terms- Fault diagnosis,root-cause analysis,feature clustering,dilated convolution neural network,Shapley additive explanations
AI 理解论文
溯源树
样例
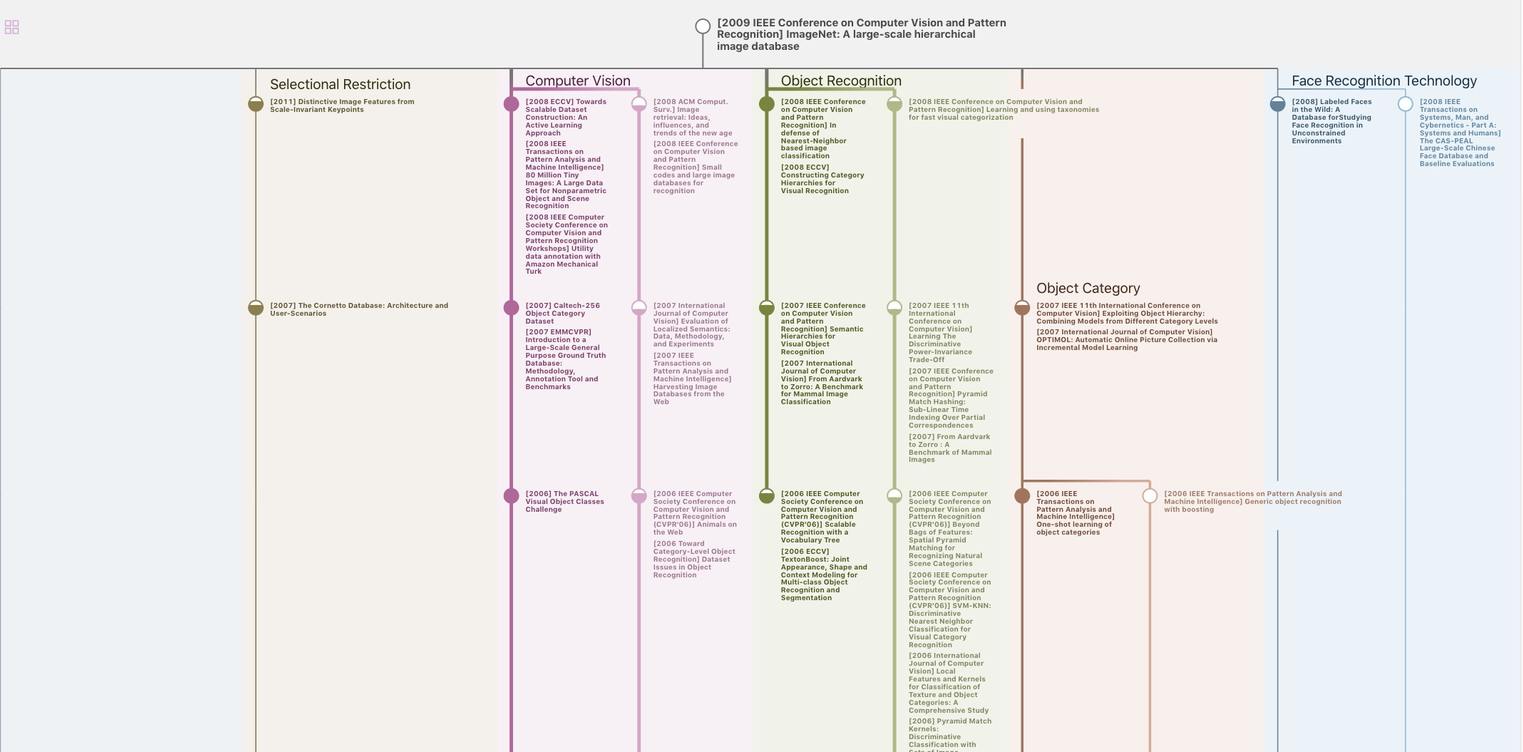
生成溯源树,研究论文发展脉络
Chat Paper
正在生成论文摘要