SCQ: Self-Supervised Cross-Modal Quantization for Unsupervised Large-Scale Retrieval
PROCEEDINGS OF 2022 ASIA-PACIFIC SIGNAL AND INFORMATION PROCESSING ASSOCIATION ANNUAL SUMMIT AND CONFERENCE (APSIPA ASC)(2022)
摘要
Cross-modal retrieval (e.g. retrieval of relevant images from the query text) from a large-scale database requires much retrieval time. Recently, fast retrieval methods without decreasing accuracy have been widely studied. If we can prepare labels that represent semantics of data, supervised deep quantization is effective for this aim. However, it is difficult to accurately annotate a large amount of data. This paper proposes an unsupervised deep quantization method for cross-modal retrieval, namely, Self-supervised Cross-modal Quantization (SCQ). SCQ enables fast retrieval without decreasing accuracy even if we cannot prepare any labels. SCQ aims to train the image quantizer and the text quantizer to quantize semantically similar (dissimilar) images and texts into the same feature vector (different feature vectors) regardless of modalities. To this end, we introduce a novel training scheme that jointly trains the image quantizer and the text quantizer with contrastive learning into SCQ. Specifically, we minimize the weighted sum of contrastive losses between the same and different modalities. This makes it possible to learn the common feature space shared by two modalities without any labels. Experimental results for two public datasets show that SCQ achieved 4.5% higher retrieval performance at most than the state-of-the-art performance.
更多查看译文
关键词
common feature space learning,contrastive learning,cross-modal retrieval,feature vector,image quantizer,large-scale database,query text,SCQ,self-supervised cross-modal quantization,text quantizer,training scheme,unsupervised deep quantization method,unsupervised large-scale retrieval
AI 理解论文
溯源树
样例
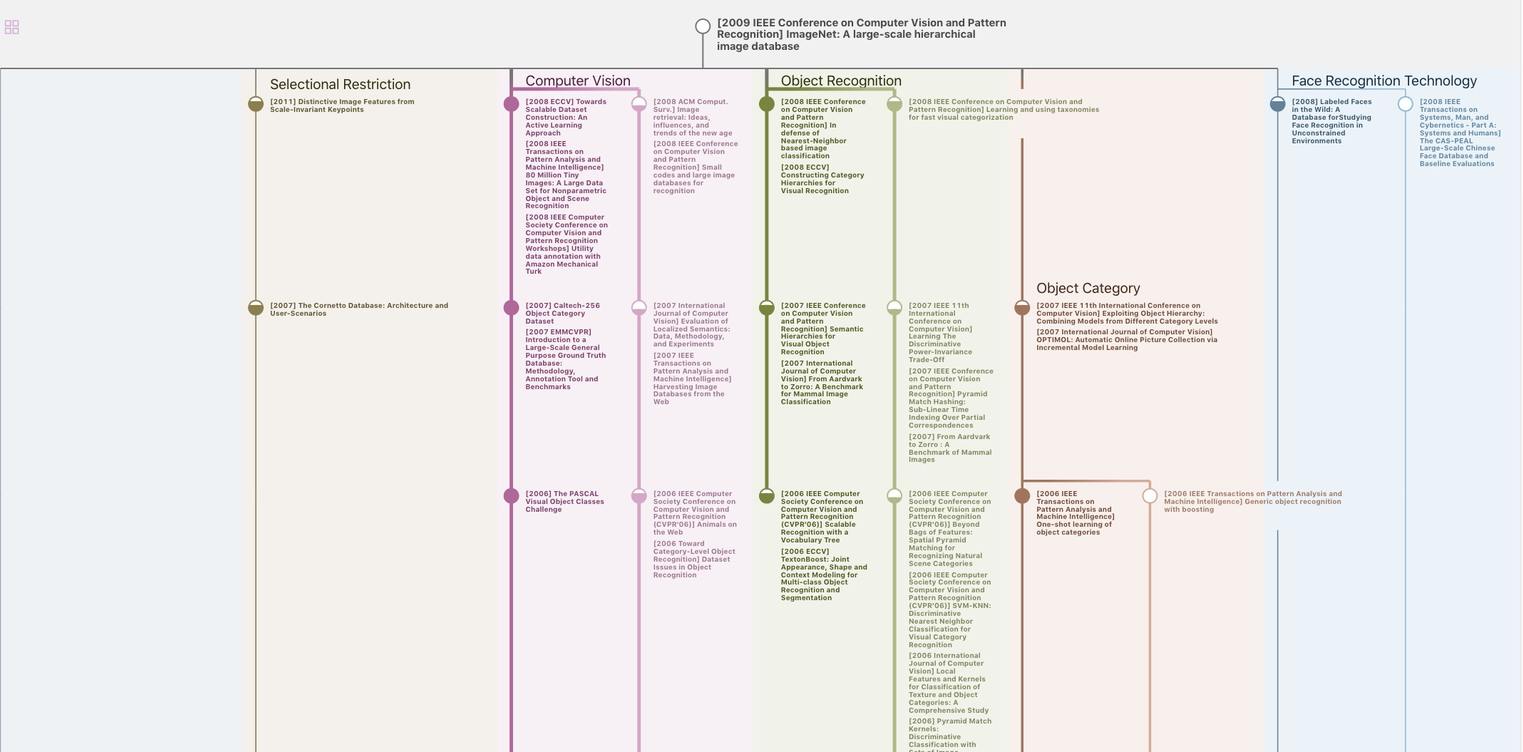
生成溯源树,研究论文发展脉络
Chat Paper
正在生成论文摘要