Structure recovery and trend estimation for dynamic network analysis
STAT(2023)
摘要
Low-dimensional parametric models for network dynamics have been successful as inferentially efficient and interpretable tools for modelling network evolution but have difficulty in settings with strong time inhomogeneity (particularly when sharp variation in parameters is possible and covariates are limited). Here, we propose to address this problem via a novel family of block-structured dynamic exponential-family random graph models (ERGMs), where the time domain is divided into consecutive blocks and the network parameters are assumed to evolve smoothly within each block. In particular, we let the latent ERGM parameters follow a piecewise polynomial model with an unknown block structure (e.g., change points). We propose an iterative estimation procedure that involves estimating the block structure using trend filtering and fitting ERGMs for networks belonging to the same time block. We demonstrate the utility of the proposed approach using simulation studies and applications to interbank transaction networks and citations among political blogs over the course of an electoral cycle.
更多查看译文
关键词
change point, exponential-family random graph model (ERGM), financial network, piecewise polynomial, structure recovery, trend filtering
AI 理解论文
溯源树
样例
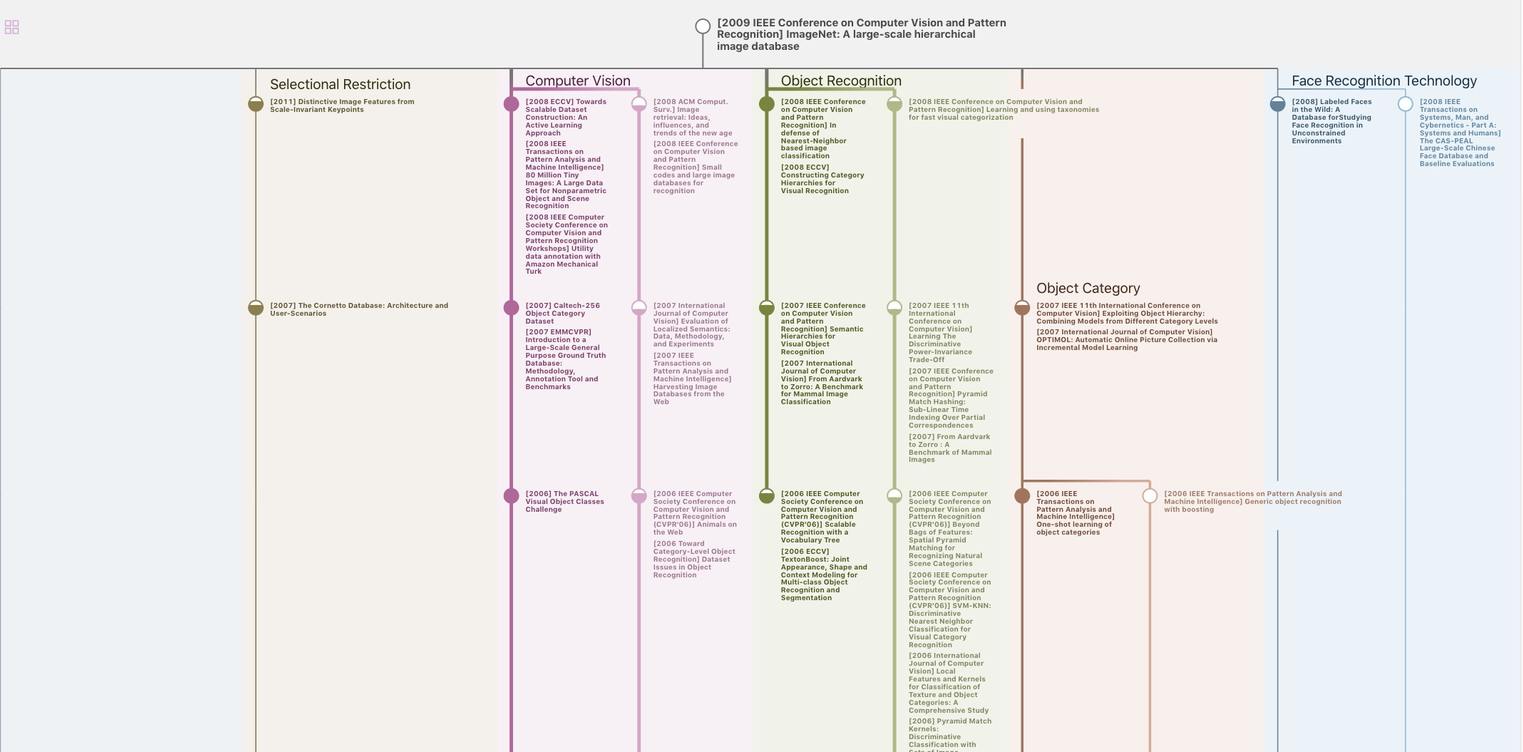
生成溯源树,研究论文发展脉络
Chat Paper
正在生成论文摘要