Intra- and Inter-Reasoning Graph Convolutional Network for Saliency Prediction on 360 degrees Images
IEEE TRANSACTIONS ON CIRCUITS AND SYSTEMS FOR VIDEO TECHNOLOGY(2022)
摘要
projection can be utilized to divide 360 degrees images into multiple rectilinear images, with little distortion. However, the existing saliency prediction models fail to integrate semantic information of these images. In this paper, we address this by proposing an intra-and inter-reasoning graph convolutional network for saliency prediction on 360 degrees images (SalReGCN360). The whole framework contains six subnetworks, each of which contains two branches. In the training phase, after utilizing Multiple Cubic Projection (MCP), six rectilinear images are simultaneously put into corresponding sub-networks. In one of the branches, the global features of a single rectilinear image are extracted by the intra-graph inference module to finely predict local saliency of 360 degrees images. In the other branch, the contextual features are extracted by the inter-graph inference module to effectively integrate semantic information of six rectilinear images. Finally, the feature maps are generated by the two branches fusion, and six corresponding rectilinear saliency maps are predicted. Extensive experiments on two popular saliency datasets illustrate the superiority of the proposed model, especially the improvement in KLD metric.
更多查看译文
关键词
Feature extraction, Semantics, Convolution, Predictive models, Image edge detection, Distortion, Data mining, Virtual reality, 360 degrees image, saliency prediction, graph convolutional network
AI 理解论文
溯源树
样例
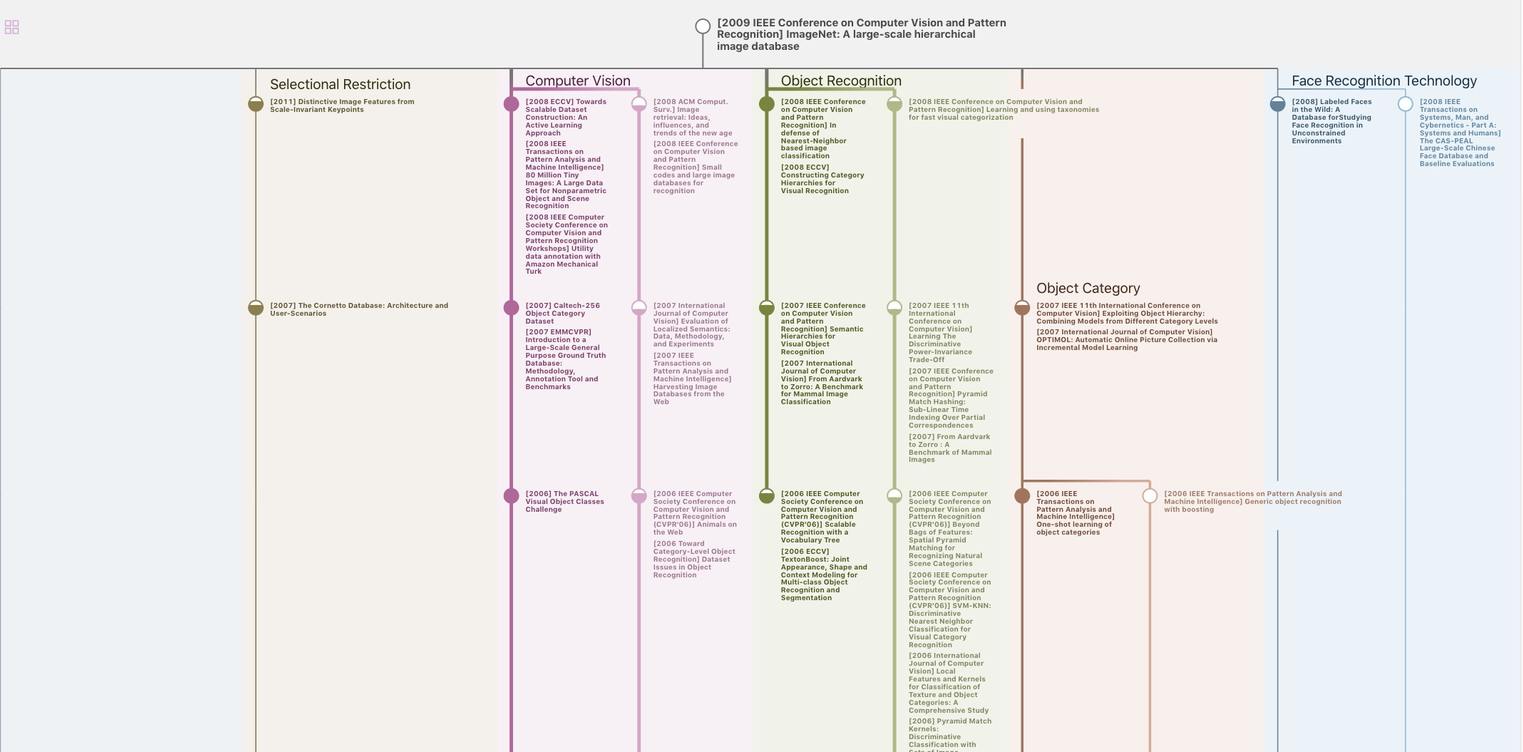
生成溯源树,研究论文发展脉络
Chat Paper
正在生成论文摘要