Data-driven and uncertainty-aware robust airstrip surface estimation
Neural Comput. Appl.(2023)
摘要
The performances of aircraft braking control systems are strongly influenced by the tire friction force experienced during the braking phase. The availability of an accurate estimate of the current airstrip characteristics is a recognized issue for developing optimized braking control schemes. The study presented in this paper is focused on the robust online estimation of the airstrip characteristics from sensory data usually available on an aircraft. In order to capture the nonlinear dependency of the current best slip on sequential slip-friction measurements acquired during the braking maneuver, multilayer perceptron (MLP) approximators have been proposed. The MLP training is based on a synthetic data set derived from a widely used tire–road friction model. In order to achieve robust predictions, MLP architectures based on the drop-out mechanism have been applied not only in the offline training phase but also during the braking. This allowed to online compute a confidence interval measure for best friction estimate that has been exploited to refine the estimation via Kalman Filtering. Open loop and closed loop simulation studies in 15 representative airstrip scenarios (with multiple surface transitions) have been performed to evaluate the performance of the proposed robust estimation method in terms of estimation error, aircraft braking distance, and time, together with a quantitative comparison with a state-of-the-art benchmark approach.
更多查看译文
关键词
Braking control,Surface estimation,Dropout neural networks,Kalman filtering
AI 理解论文
溯源树
样例
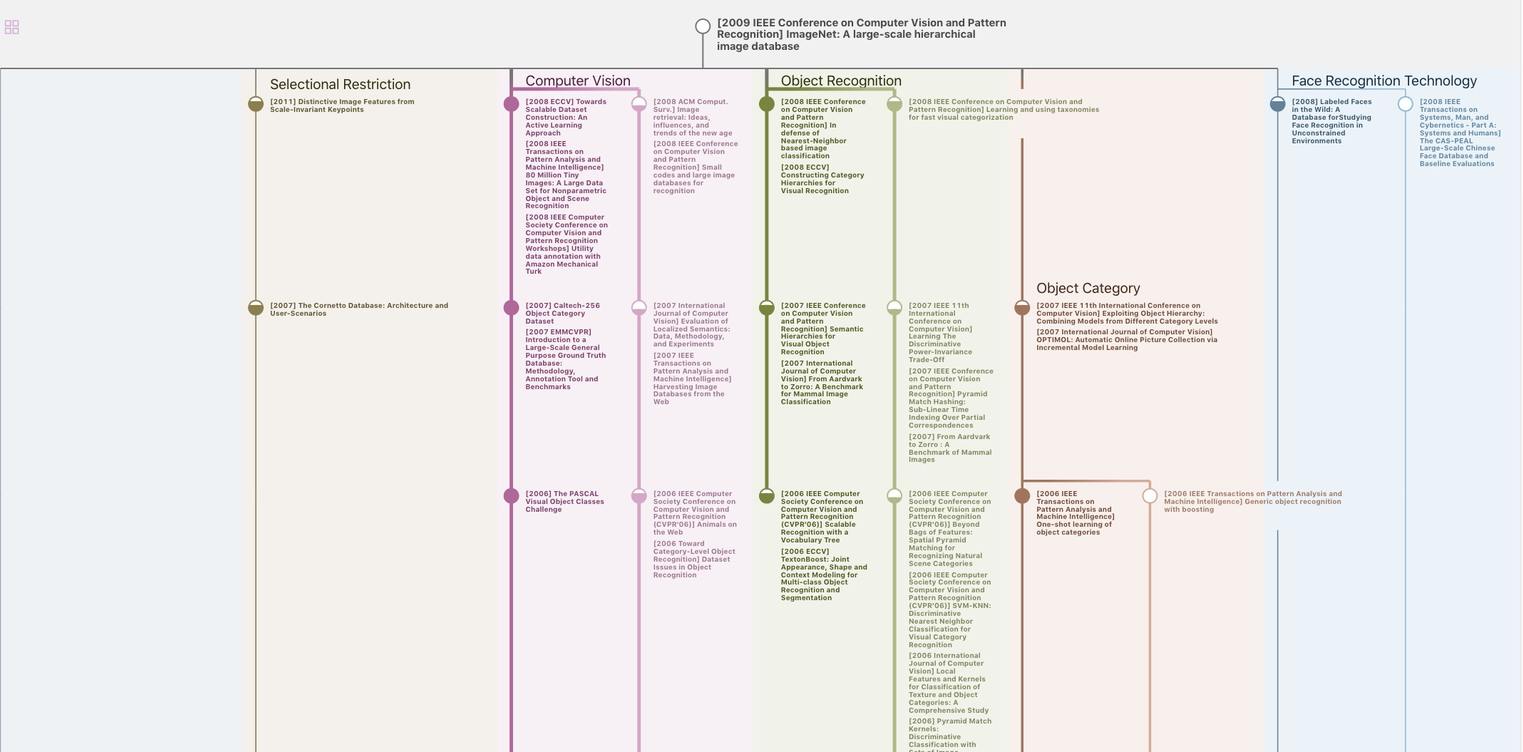
生成溯源树,研究论文发展脉络
Chat Paper
正在生成论文摘要