Trajectories of fasting glucose and glycated haemoglobin in obese and non-obese incident diabetes: Results from two large cohort studies
STATISTICS IN MEDICINE(2023)
摘要
Alzheimer's disease (AD) is a severe public health issue in the world. Magnetic Resonance Imaging (MRI) offers a way to study brain differences between AD patients and healthy individuals through feature extraction and comparison. However, in most previous works, the extracted features were not aimed to be causal, hindering biological understanding and interpretation. In order to extract causal features, we propose using instrumental variable (IV) regression with genetic variants as IVs. Specifically, we propose Deep Feature Extraction via Instrumental Variable Regression (DeepFEIVR), which uses a nonlinear neural network to extract causal features from three-dimensional neuroimages to predict an outcome (eg, AD status in our application) while maintaining a linear relationship between the extracted features and IVs. DeepFEIVR not only can handle high dimensional individual-level data for model building, but also is applicable to GWAS summary data to test associations of the extracted features with the outcome in subsequent analysis. In addition, we propose an extension of DeepFEIVR, called DeepFEIVR-CA, for covariate adjustment (CA). We apply DeepFEIVR and DeepFEIVR-CA to the Alzheimer's Disease Neuroimaging Initiative (ADNI) individual-level data as training data for model building, then apply to the UK Biobank neuroimaging and the International Genomics of Alzheimer's Project (IGAP) AD GWAS summary data, showcasing how the extracted causal features are related to AD and various brain endophenotypes.
更多查看译文
关键词
Alzheimer's disease, causal inference, instrumental variable, MRI, neural networks
AI 理解论文
溯源树
样例
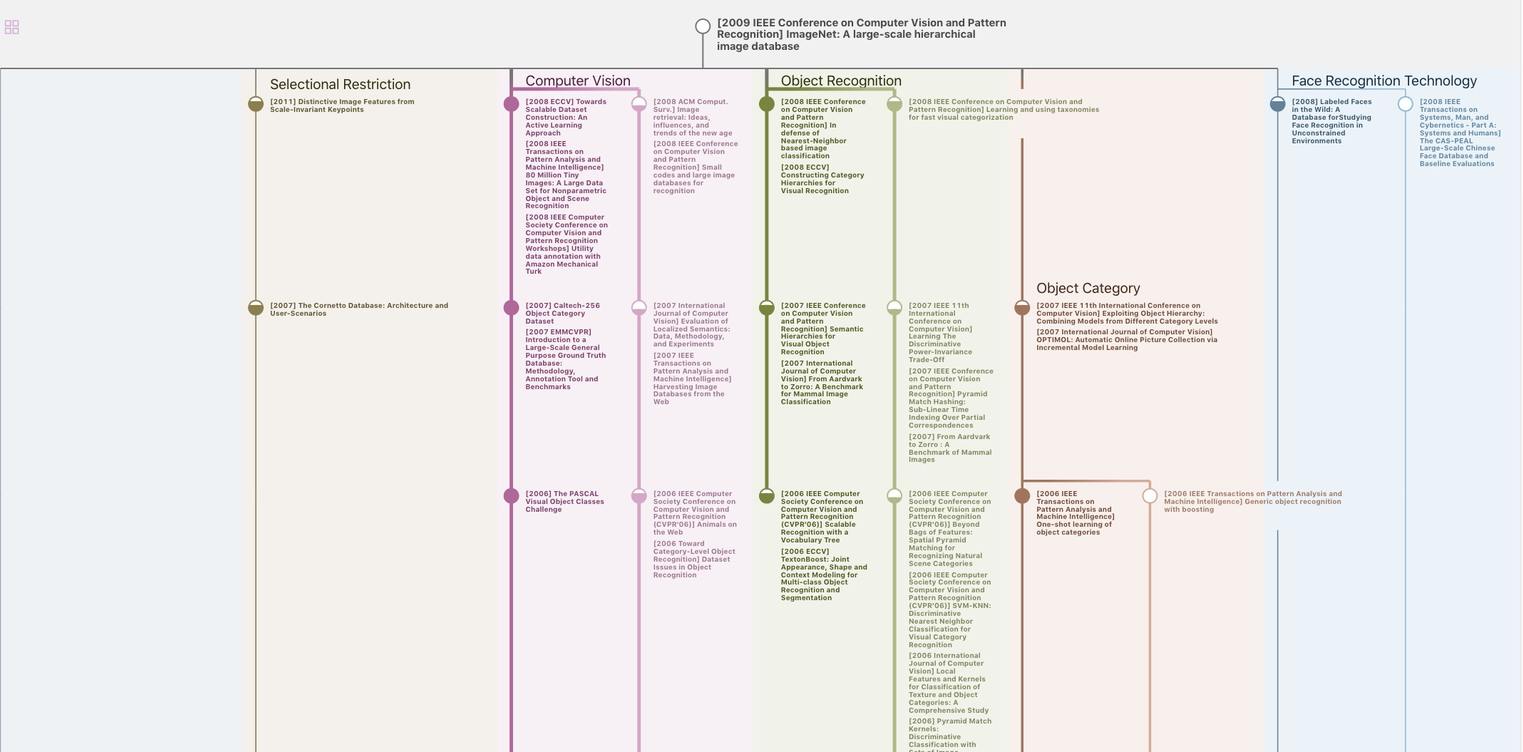
生成溯源树,研究论文发展脉络
Chat Paper
正在生成论文摘要