Detection of knee osteoarthritis based on recurrence quantification analysis, fuzzy entropy and shallow classifiers
Multimedia Tools and Applications(2024)
摘要
Knee osteoarthritis (OA) is the most common joint disorder which results in mobility impairment and altered gait patterns. For the purpose of developing an automatic and highly accurate diagnosis system for knee OA, this study investigated the classification capability of different dynamical features extracted from gait kinematic signals when evaluating their impact on different classification models. A general feature extraction framework was proposed and various dynamical features, such as recurrence rate, determinism and entropy from the recurrence quantification analysis (RQA), Fuzzy entropy and statistical analysis, were included. Different shallow classifiers, including support vector machine (SVM) classifier, K-nearest neighbor (KNN), naive Bayes (NB) classifier, decision tree (DT) and ensemble learning based Adaboost (ELA) classifier, derived for discriminant analysis of multiple dynamical gait features were evaluated on the classification accuracy for a comparative study. The effectiveness of this strategy was verified using a dataset of tibiofemoral joint angle and translation waveforms from 26 patients with knee OA and 26 age-matched asymptomatic healthy controls (HCs). When evaluated with two-fold and leave-one-subject-out cross-validation methods, the highest classification accuracy for discriminating between groups of patients with knee OA and HCs was reported to be 92.31% and 100% , respectively, by using the SVM classifier. Compared with other state-of-the-art methods, the results demonstrate superior performance and support the validity of the proposed method.
更多查看译文
关键词
Knee osteoarthritis (OA),Dynamical features,Gait classification,Recurrence quantification analysis (RQA),Fuzzy entropy,Machine learning
AI 理解论文
溯源树
样例
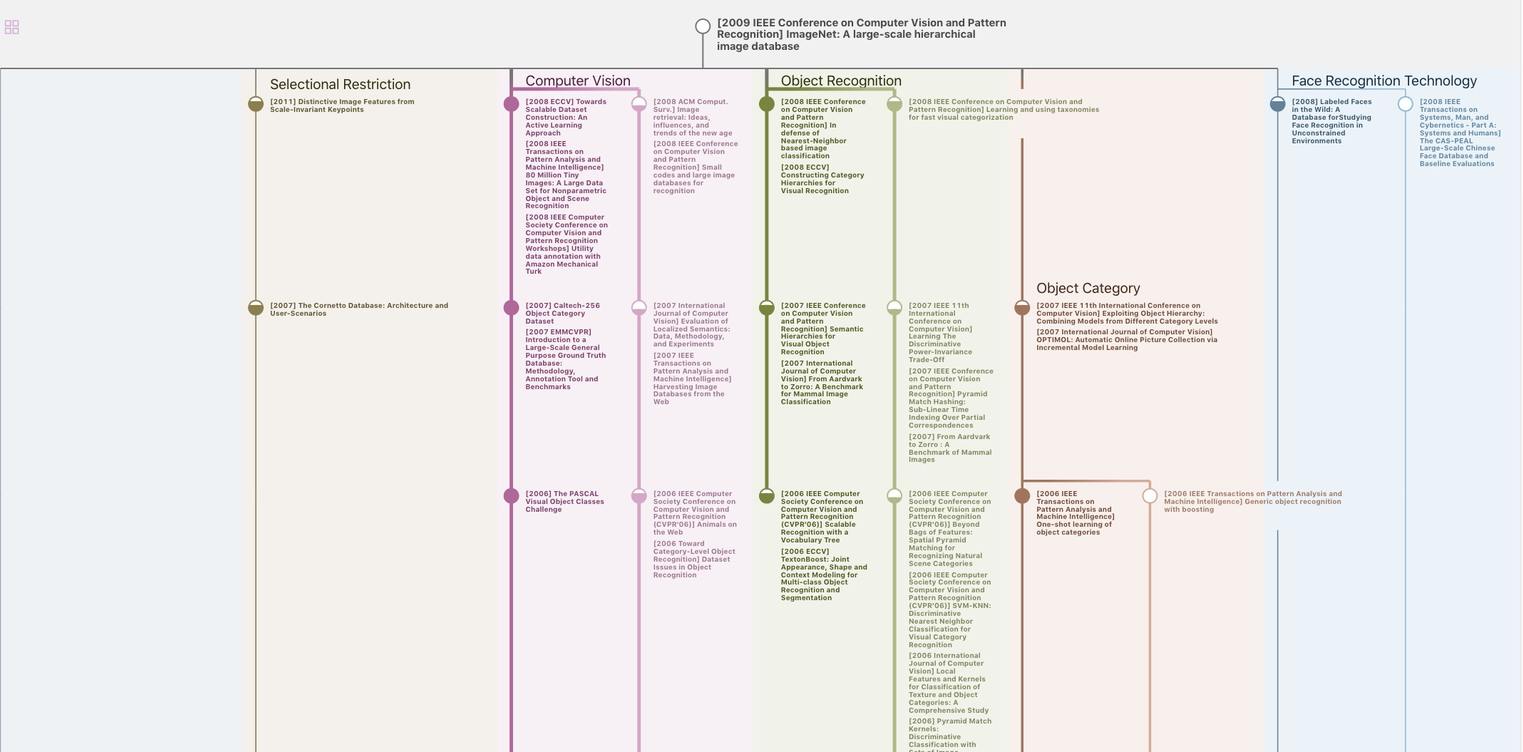
生成溯源树,研究论文发展脉络
Chat Paper
正在生成论文摘要