Comparative Analysis of Epileptic Seizure Prediction: Exploring Diverse Pre-Processing Techniques and Machine Learning Models
CoRR(2023)
摘要
Epilepsy is a prevalent neurological disorder characterized by recurrent and unpredictable seizures, necessitating accurate prediction for effective management and patient care. Application of machine learning (ML) on electroencephalogram (EEG) recordings, along with its ability to provide valuable insights into brain activity during seizures, is able to make accurate and robust seizure prediction an indispensable component in relevant studies. In this research, we present a comprehensive comparative analysis of five machine learning models - Random Forest (RF), Decision Tree (DT), Extra Trees (ET), Logistic Regression (LR), and Gradient Boosting (GB) - for the prediction of epileptic seizures using EEG data. The dataset underwent meticulous preprocessing, including cleaning, normalization, outlier handling, and oversampling, ensuring data quality and facilitating accurate model training. These preprocessing techniques played a crucial role in enhancing the models' performance. The results of our analysis demonstrate the performance of each model in terms of accuracy. The LR classifier achieved an accuracy of 56.95%, while GB and DT both attained 97.17% accuracy. RT achieved a higher accuracy of 98.99%, while the ET model exhibited the best performance with an accuracy of 99.29%. Our findings reveal that the ET model outperformed not only the other models in the comparative analysis but also surpassed the state-of-the-art results from previous research. The superior performance of the ET model makes it a compelling choice for accurate and robust epileptic seizure prediction using EEG data.
更多查看译文
关键词
epileptic seizure prediction,epileptic seizure,machine learning,pre-processing
AI 理解论文
溯源树
样例
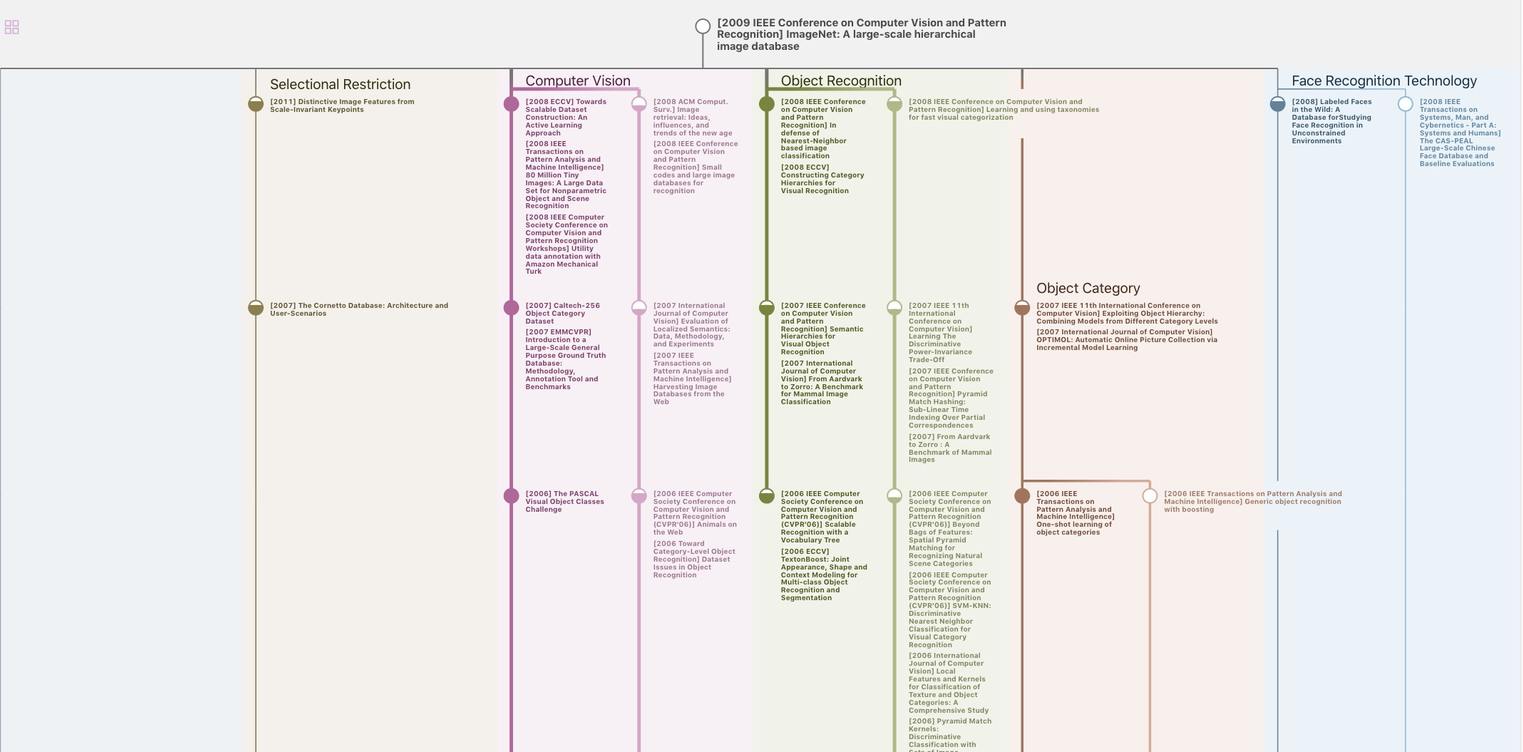
生成溯源树,研究论文发展脉络
Chat Paper
正在生成论文摘要