DegUIL: Degree-Aware Graph Neural Networks for Long-Tailed User Identity Linkage
MACHINE LEARNING AND KNOWLEDGE DISCOVERY IN DATABASES: APPLIED DATA SCIENCE AND DEMO TRACK, ECML PKDD 2023, PT VI(2023)
摘要
User identity linkage (UIL), matching accounts of a person on different social networks, is a fundamental task in cross-network data mining. Recent works have achieved promising results by exploiting graph neural networks (GNNs) to capture network structure. However, they rarely analyze the realistic node-level bottlenecks that hinder UIL's performance. First, node degrees in a graph vary widely and are long-tailed. A significant fraction of tail nodes with small degrees are underrepresented due to limited structural information, degrading linkage performance seriously. The second bottleneck usually overlooked is super head nodes. It is commonly accepted that head nodes perform well. However, we find that some of them with super high degrees also have difficulty aligning counterparts, due to noise introduced by the randomness of following friends in real-world social graphs. In pursuit of learning ideal representations for these two groups of nodes, this paper proposes a degree-aware model named DegUIL to narrow the degree gap. To this end, our model complements missing neighborhoods for tail nodes and discards redundant structural information for super head nodes in embeddings respectively. Specifically, the neighboring bias is predicted and corrected locally by two modules, which are trained using the knowledge from structurally adequate head nodes. As a result, ideal neighborhoods are obtained for meaningful aggregation in GNNs. Extensive experiments demonstrate the superiority of our model. Our data and code can be found at https://github.com/Longmeix/DegUIL.
更多查看译文
关键词
User identity linkage,Long-tailed graph representation learning,Graph neural networks
AI 理解论文
溯源树
样例
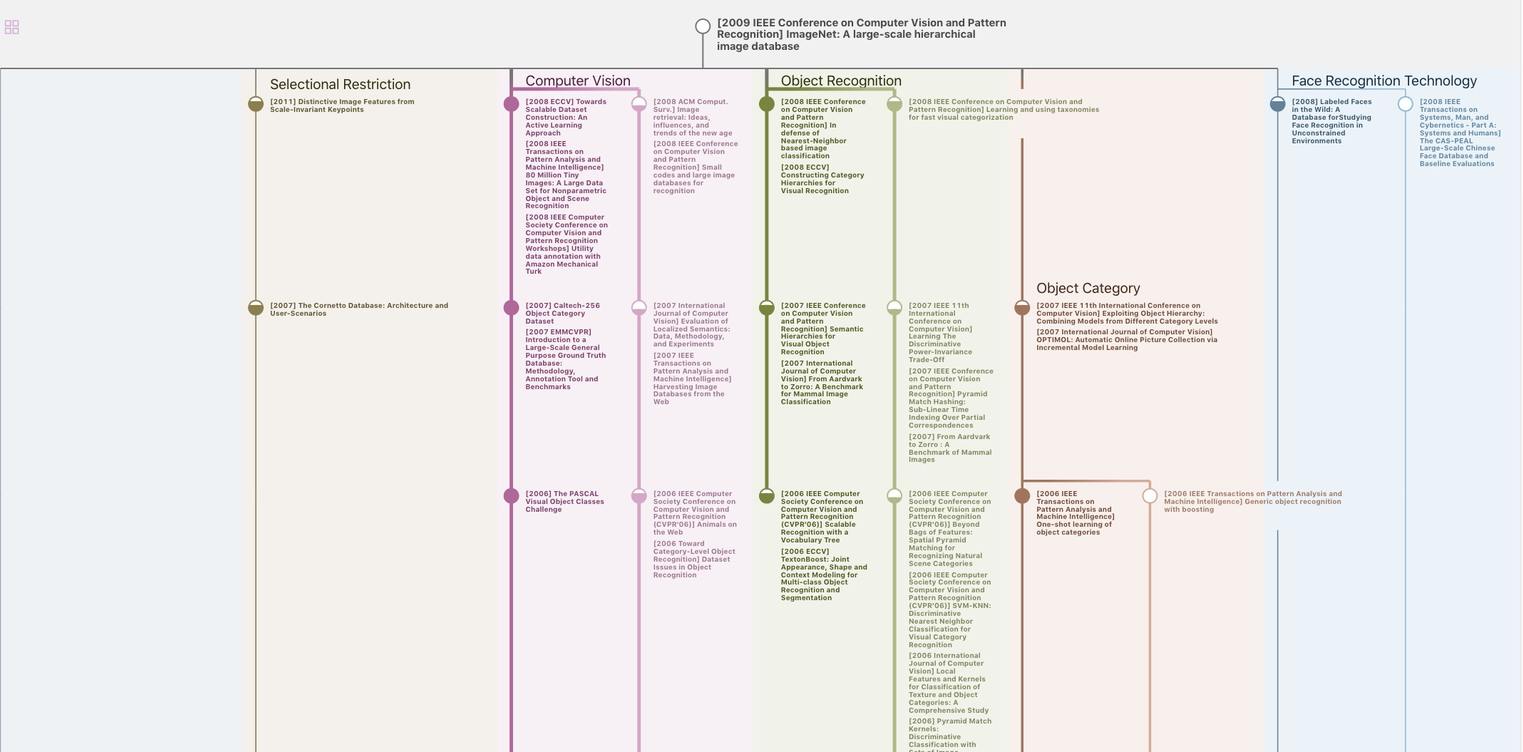
生成溯源树,研究论文发展脉络
Chat Paper
正在生成论文摘要