MaizeNetome: A multi-omics network database for functional genomics in maize
Molecular plant(2023)
摘要
Eukaryotic life is a complex system (Trewavas, 2006Trewavas A. A brief history of systems biology Every object that biology studies is a system of systems." Francois Jacob.Plant Cell. 2006; 18: 2420-2430https://doi.org/10.1105/tpc.106.042267Crossref PubMed Scopus (117) Google Scholar). Networks offer a reasonable means of describing complex life systems. Networks constructed for animals and plants have significantly furthered our understanding of complex life systems and functional genomics (Consortium, 2011Arabidopsis Interactome Mapping ConsortiumEvidence for network evolution in an Arabidopsis interactome map.Science. 2011; 333: 601-607https://doi.org/10.1126/science.1203877Crossref PubMed Google Scholar; Walley et al., 2016Walley J.W. Sartor R.C. Shen Z. Schmitz R.J. Wu K.J. Urich M.A. Smith L.G. Schnable J.C. Ecker J.R. Briggs S.P. et al.Integration of omic networks in a developmental atlas of maize.Science. 2016; 353: 814-818Crossref PubMed Scopus (270) Google Scholar; Altmann et al., 2020Altmann M. Altmann S. Rodriguez P.A. Weller B. Elorduy Vergara L. Palme J. Marín-de la Rosa N. Sauer M. Wenig M. Villaécija-Aguilar J.A. et al.Extensive signal integration by the phytohormone protein network.Nature. 2020; 583: 271-276https://doi.org/10.1038/s41586-020-2460-0Crossref PubMed Scopus (67) Google Scholar; Luck et al., 2020Luck K. Kim D.K. Lambourne L. Spirohn K. Begg B.E. Bian W. Brignall R. Cafarelli T. Campos-Laborie F.J. Charloteaux B. et al.A reference map of the human binary protein interactome.Nature. 2020; 580: 402-408https://doi.org/10.1038/s41586-020-2188-xCrossref PubMed Scopus (472) Google Scholar; McWhite et al., 2020McWhite C.D. Papoulas O. Drew K. Cox R.M. June V. Dong O.X. Kwon T. Wan C. Salmi M.L. Roux S.J. et al.A pan-plant protein complex map reveals deep conservation and novel assemblies.Cell. 2020; 181: 460-474.e14https://doi.org/10.1016/j.cell.2020.02.049Abstract Full Text Full Text PDF PubMed Scopus (97) Google Scholar; Zander et al., 2020Zander M. Lewsey M.G. Clark N.M. Yin L. Bartlett A. Saldierna Guzmán J.P. Hann E. Langford A.E. Jow B. Wise A. et al.Integrated multi-omics framework of the plant response to jasmonic acid.Nat. Plants. 2020; 6: 290-302https://doi.org/10.1038/s41477-020-0605-7Crossref PubMed Scopus (109) Google Scholar). However, integrative networks spanning different layers of genetic information are lacking. In previous research, we constructed MaizeNetome, a comprehensive Maize Network database (Figure 1A) containing over 15 million edges for more than 1000 functional modules. This database covers the flow of genetic information from genome to transcriptome (co-expression network from RNA sequencing [RNA-seq]), to translatome (co-translational network from ribosome profiling sequencing [Ribo-seq]), and to interactome (Han et al., 2023Han L. Zhong W. Qian J. Jin M. Tian P. Zhu W. Zhang H. Sun Y. Feng J.-W. Liu X. et al.A multi-omics integrative network map of maize.Nat. Genet. 2023; 55: 144-153https://doi.org/10.1038/s41588-022-01262-1Crossref PubMed Scopus (11) Google Scholar). We curated 10 networks derived from five different omics (genome, transcriptome, translatome, interactome, and integrative omics) at two confidence levels (high and low) and integrated them into our MaizeNetome database (Figure 1B). This integrative netome makes not only evolutionary sense but also biological sense, and it can be used for systematic functional genetics dissection (Han et al., 2023Han L. Zhong W. Qian J. Jin M. Tian P. Zhu W. Zhang H. Sun Y. Feng J.-W. Liu X. et al.A multi-omics integrative network map of maize.Nat. Genet. 2023; 55: 144-153https://doi.org/10.1038/s41588-022-01262-1Crossref PubMed Scopus (11) Google Scholar). The MaizeNetome database is not only the largest gene-to-gene network to our knowledge but also includes several user-friendly tools to enable its use in functional genomics: BLAST, Gbrowse, Module Search, Element Search, Element Fetch, Network Creation, Module Hit, and Network Comparison tools, plus QTG (quantitative trait gene) Interaction Score and Pathway Mapping analyses (Figure 1C). To efficiently explore this large network database, we designed MaizeNetome in three layers. The whole network is classified into hub networks, which show the network architecture of different omics; directly connected networks, which show direct relationships among specific nodes (genes); and module networks, which are a subset of coherent functional genes. Accordingly, two strategies—reverse genetics (starting from a gene/node; Figure 1D) and forward genetics (starting from a complex trait; Figure 1E)—were devised for systematic dissection of functional genomic relationships. For reverse genetics analyses, the Element Search tool allows users to query functional annotations, expression information across multiple tissues, module networks, and directly connected networks. We recommend a further Gene Ontology enrichment analysis using the gene identifiers (IDs) in these networks to hypothesize functions of query elements. Functional comparison of different genes using the Network Comparison tool is also available. Taking Zm00001d042282 as an example, users can obtain the Zm00001d042282 ID by sequence alignment, genomic location, or B73 Version 4 ID. Element Search delivers a series of annotation information on expression at the transcription and translation levels, module networks, and directly connected networks for each omics. When dissecting the functional relationship of Zm00001d042282 with Zm00001d017601, Network Comparison displays the similarities and differences between two nodes/genes at the omics levels (Figure 1D). Most agronomic traits are quantitative traits conferred by several genes and their complicated interactions. Forward genetics is effective for dissecting the molecular mechanisms of agronomic traits. The MaizeNetome database has several forward genetics tools based on our network big data, such as QTG Interaction Score and Pathway Mapping (Figure 1E). Although MaizeNetome is large, it is not saturated. We devised Pathway Mapping to infer potential interactions and predict new networks according to the shortest distance in different omics networks. Pathway Mapping can help users understand the molecular pathways underlying complex traits. For example, a network relationship constructed by Pathway Mapping using 19 genes related to plant height can further our understanding of plant development in maize (Supplemental Table 1; Figure 1F). Users can not only identify interactions with plant height-related genes under different omics but also search for previously uncharacterized interactions. MaizeNetome illustrates functional relationships among genes from the network structure (Han et al., 2023Han L. Zhong W. Qian J. Jin M. Tian P. Zhu W. Zhang H. Sun Y. Feng J.-W. Liu X. et al.A multi-omics integrative network map of maize.Nat. Genet. 2023; 55: 144-153https://doi.org/10.1038/s41588-022-01262-1Crossref PubMed Scopus (11) Google Scholar). We used the MaizeNetome map to develop QTG Interaction Score analysis to prioritize the candidate gene for a quantitative trait locus (QTL) based on preliminary QTL mapping. Fine mapping and cloning QTLs spanning a large genomic region is time consuming and costly (Wu et al., 2021Wu L. Han L. Li Q. Wang G. Zhang H. Li L. Using interactome big data to crack genetic mysteries and enhance future crop breeding.Mol. Plant. 2021; 14: 77-94https://doi.org/10.1016/j.molp.2020.12.012Abstract Full Text Full Text PDF PubMed Scopus (28) Google Scholar). QTG Interaction Score quickly narrows down the target genes associated with a QTL. To test the robustness of the QTG Interaction Score, we treated 36 well-known flowering time genes and 19 plant height genes (Supplemental Table 2) as positive QTGs of QTLs with 1- to 2-Mb genomic regions. QTG Interaction Score predicted potential genes located in each QTL and sorted candidate genes by their potential scores. The higher the score, the more likely the gene is the QTG. The accuracy of prediction in the top 30% of genes was up to 49% using Co-translation Network and Slim Co-expression Network (Figure 1G, Supplemental Table 3). Taking qPH7 located at chromosome 7:128 190 000–138 120 000 (B73V4) and differentially expressed genes identified in a previous study (Zhang et al., 2019Zhang H. Wang X. Pan Q. Li P. Liu Y. Lu X. Zhong W. Li M. Han L. Li J. et al.QTG-Seq accelerates QTL fine mapping through QTL partitioning and whole-genome sequencing of bulked segregant samples.Mol. Plant. 2019; 12: 426-437https://doi.org/10.1016/j.molp.2018.12.018Abstract Full Text Full Text PDF PubMed Scopus (67) Google Scholar) as an example, QTG Interaction Score ranked the causal gene fourth out of 209 in the QTL range (Co-translation Network) (Supplemental Table 4). When the region was narrowed down to only 2 Mb (7:134 000 000–136 000 000), the target gene was ranked first out of 52 genes using Co-expression Network (Supplemental Table 4). Users can quickly identify the causal QTG by observing whether genes are differentially expressed and whether mutations occur in the QTL. In summary, the MaizeNetome database is a comprehensive, big-data, molecular network that we believe is the first and largest multi-omics network database in eukaryotes. With rapidly advancing network detection techniques, we plan to update the maize interactome database regularly. However, the real internal environment is very complex, and it remains a huge challenge to estimate biological processes in vivo. MaizeNetome is based on the maize B73 version 4 genome reference, limiting its applicability to other maize genotypes and other species, especially where the functions of homologous genes are different. MaizeNetome is a robust big-data mining platform that will aid forward and reverse genetics research and functional genomics studies in plants. The MaizeNetome database is freely available at http://minteractome.ncpgr.cn/. This research was supported by the National Natural Science Foundation of China (32272158, 32270712, 92035302) and Outstanding Youth Team Project of Center Universities (2662023PY007). We thank the high-performance computing platform at the National Key Laboratory of Crop Genetic Improvement in Huazhong Agricultural University.
更多查看译文
关键词
functional genomics,maizenetome,multi-omics
AI 理解论文
溯源树
样例
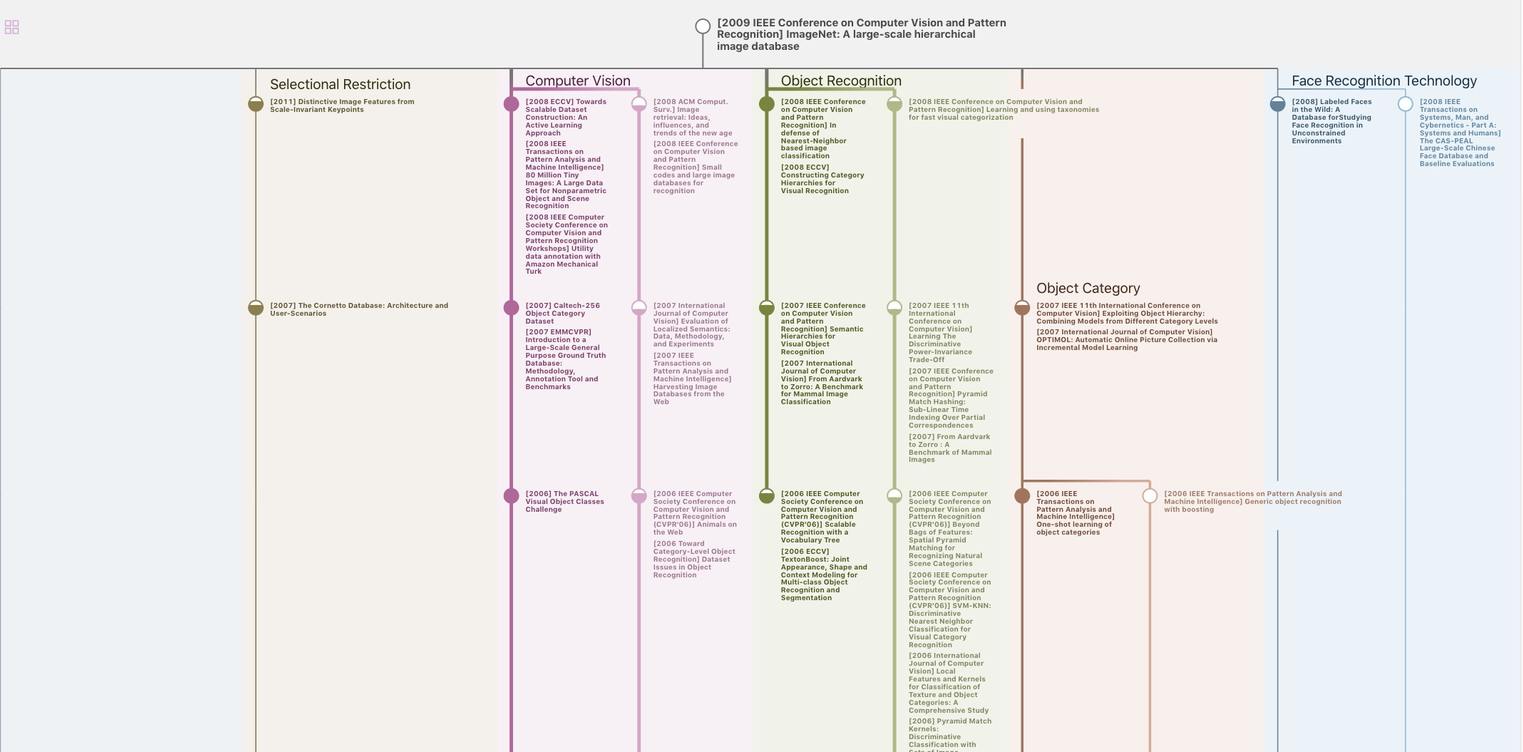
生成溯源树,研究论文发展脉络
Chat Paper
正在生成论文摘要