Non-invasive tumor microenvironment evaluation and treatment response prediction in gastric cancer using deep learning radiomics.
Cell reports. Medicine(2023)
摘要
The tumor microenvironment (TME) plays a critical role in disease progression and is a key determinant of therapeutic response in cancer patients. Here, we propose a noninvasive approach to predict the TME status from radiological images by combining radiomics and deep learning analyses. Using multi-institution cohorts of 2,686 patients with gastric cancer, we show that the radiological model accurately predicted the TME status and is an independent prognostic factor beyond clinicopathologic variables. The model further predicts the benefit from adjuvant chemotherapy for patients with localized disease. In patients treated with checkpoint blockade immunotherapy, the model predicts clinical response and further improves predictive accuracy when combined with existing biomarkers. Our approach enables noninvasive assessment of the TME, which opens the door for longitudinal monitoring and tracking response to cancer therapy. Given the routine use of radiologic imaging in oncology, our approach can be extended to many other solid tumor types.
更多查看译文
关键词
tumor microenvironment,immunotherapy,deep learning,CT image,gastric cancer,radiomics,treatment response
AI 理解论文
溯源树
样例
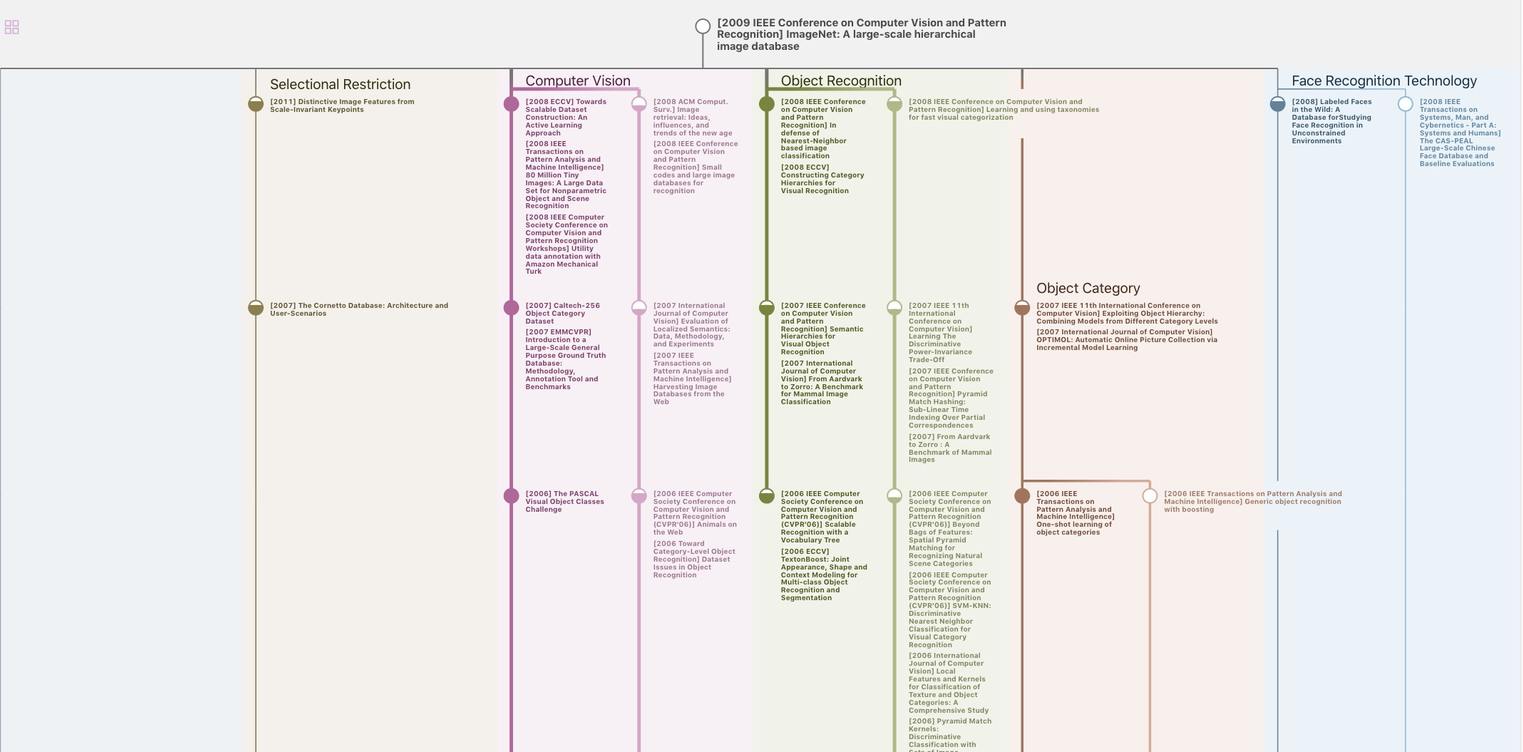
生成溯源树,研究论文发展脉络
Chat Paper
正在生成论文摘要