Combination of the electronic nose with microbiology as a tool for rapid detection of Salmonella.
Journal of microbiological methods(2023)
摘要
Salmonella is one of the most important foodborne pathogens and its analysis in raw and processed products is mandatory in the food industry. Although microbiological analysis is the standard practice for Salmonella determination, these assays are commonly laborious and time-consuming, thus, alternative techniques based on easy operation, few manipulation steps, low cost, and reduced time are desirable. In this paper, we demonstrate the use of an e-nose based on ionogel composites (ionic liquid + gelatine + FeO particles) as a complementary tool for the conventional microbiological detection of Salmonella. We used the proposed methodology for differentiating Salmonella from Escherichia coli, Pseudomonas fluorescens, Pseudomonas aeruginosa, and Staphylococcus aureus in nonselective medium: pre-enrichment in brain heart infusion (BHI) (incubation at 35 °C, 24 h) and enrichment in tryptone soy agar (TSA) (incubation at 35 °C, 24 h), whereas Salmonella differentiation from E. coli and P. fluorescens was also evaluated in selective media, bismuth sulfite agar (BSA), xylose lysine deoxycholate agar (XLD), and brilliant green agar (BGA) (incubation at 35 °C, 24 h). The obtained data were compared by principal component analysis (PCA) and different machine learning algorithms: multilayer perceptron (MLP), linear discriminant analysis (LDA), instance-based (IBk), and Logistic Model Trees (LMT). For the nonselective media, under optimized conditions, taking merged data of BHI + TSA (total incubation time of 48 h), an accuracy of 85% was obtained with MLP, LDA, and LMT, while five separated clusters were presented in PCA, each cluster corresponding to a bacterium. In addition, for evaluation of the e-nose for discrimination of Salmonella using selective media, considering the combination of BSA + XLD and total incubation of 72 h, the PCA showed three separated and well-defined clusters corresponding to Salmonella, E. coli, and P. fluorescens, and an accuracy of 100% was obtained for all classifiers.
更多查看译文
关键词
Salmonella,Microbiology,Electronic nose,Machine learning,Food safety
AI 理解论文
溯源树
样例
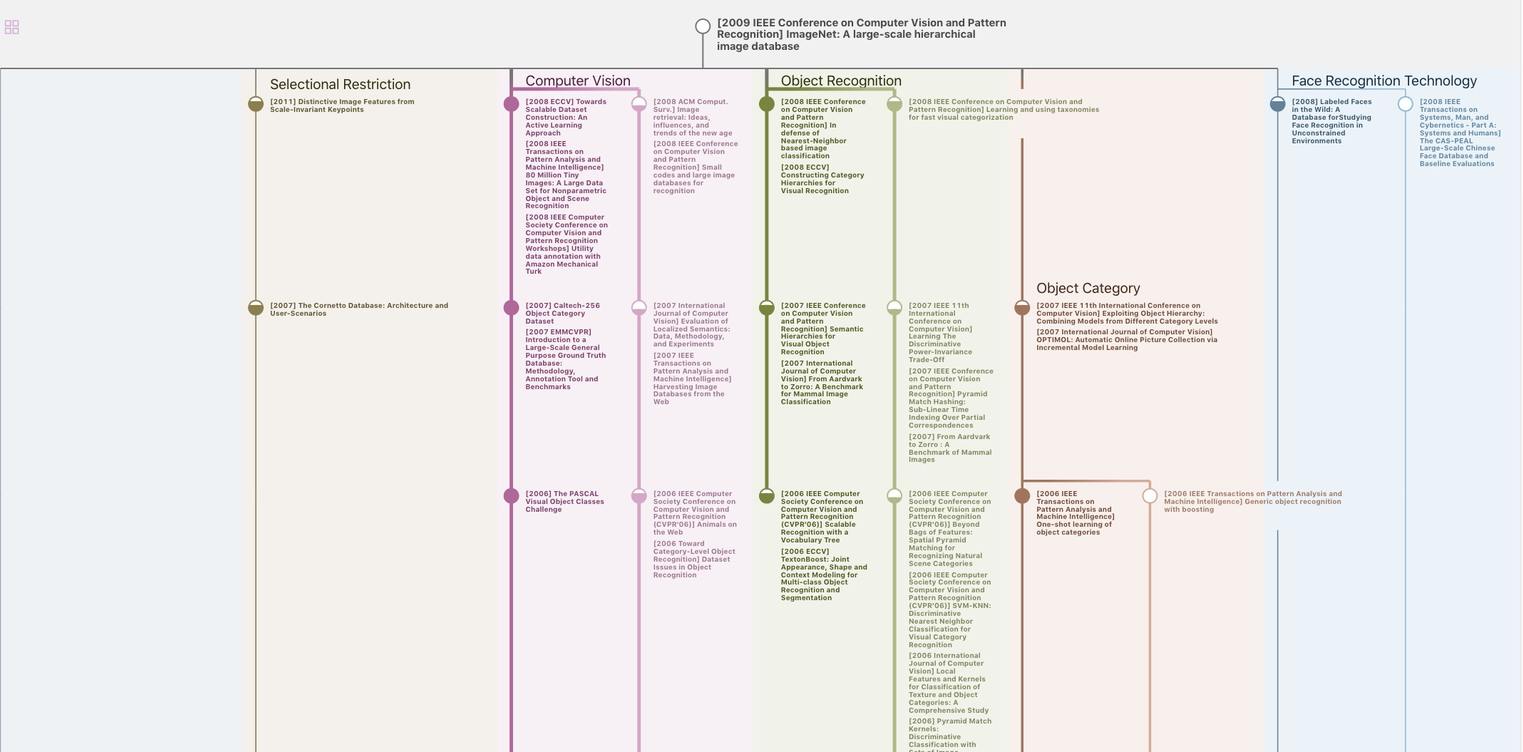
生成溯源树,研究论文发展脉络
Chat Paper
正在生成论文摘要