Accurate Dimension Prediction for Low-Dimensional Organic-Inorganic Halide Perovskites via a Self-Established Machine Learning Strategy.
The journal of physical chemistry letters(2023)
摘要
Low-dimensional perovskites (LDPs) have enormous potential for the development of advanced optoelectronic devices and tackling the stability issue for the commercial application of perovskites. However, quantified structural dimensionality prediction for LDPs is still an intractable issue. Herein, we develop a self-established machine learning (ML)-assisted approach to predict the dimensionality of LDPs based on 195 reported amines that are classified as two-dimensional, one-dimensional, and zero-dimensional. The optimal K-nearest neighbor model allows us to realize an accuracy rate of 92.3% for the test data set containing 39 reported amines. Two features, i.e., ATSC1pe and SlogP_VSA2, associated with polarity and electrostatic potential on the van der Waals surface of an organic spacer, are identified from >1800 descriptors as key controlling factors determining the structure dimensionality. This work develops a typical paradigm for the application of a multiple-classification strategy of ML with an extremely high accuracy rate, which would thereby motivate the development of new types of LDPs.
更多查看译文
关键词
organic–inorganic halide perovskites,machine learning,low-dimensional,self-established
AI 理解论文
溯源树
样例
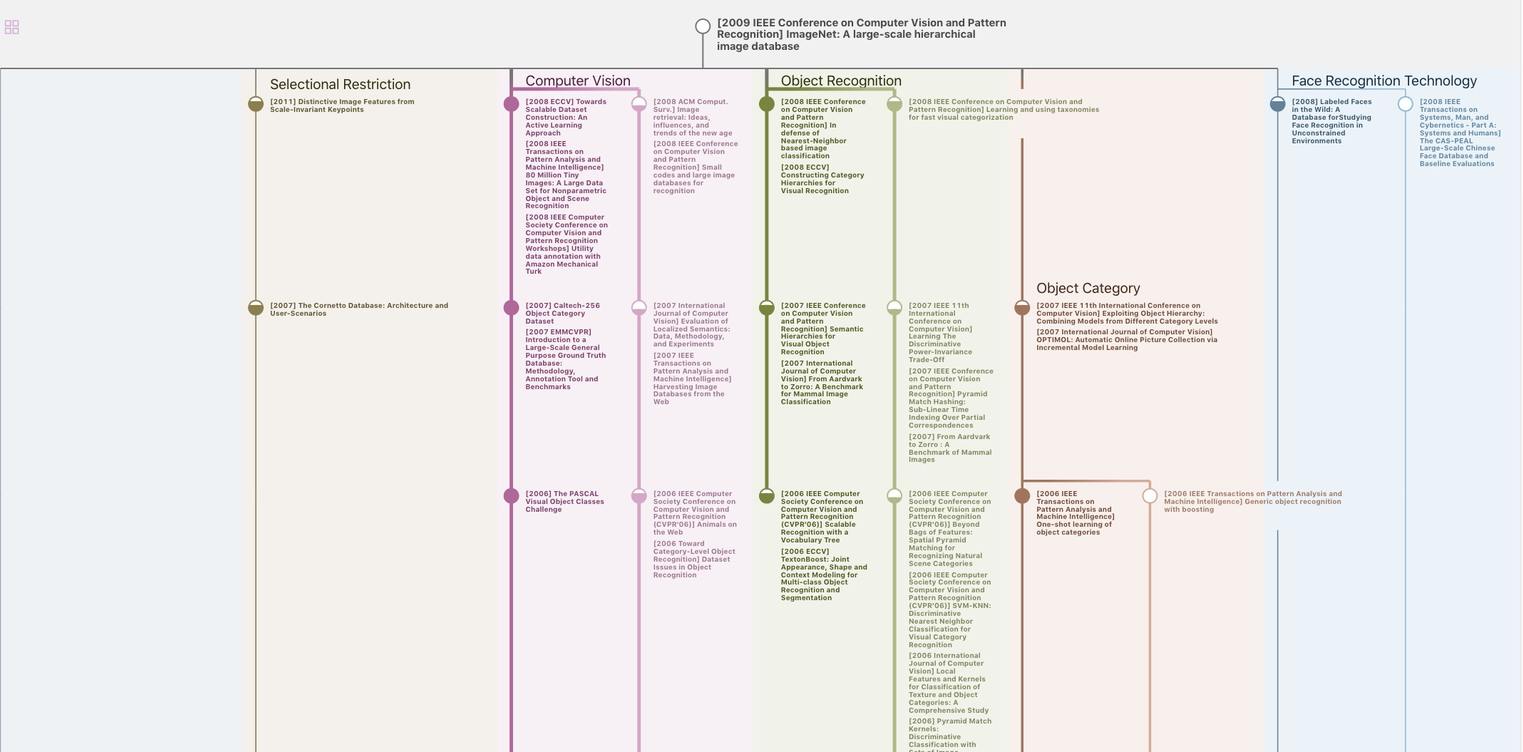
生成溯源树,研究论文发展脉络
Chat Paper
正在生成论文摘要