A weighted fuzzy belief factor-based D-S evidence theory of sensor data fusion method and its application to face recognition
Multimedia Tools and Applications(2024)
摘要
Automatic Face Recognition (AFR) plays an important role in wide range of applications such as, smart cities, consumer-level and organizational-level applications. AFR is one of the formidable tasks because the face images are susceptible to various degradations by illumination, noise and pose variations. Significant efforts have been manifested in biometric (single/multiple) fusion methods. Prior works on Dempster-Shafer (D-S) evidence theory based fusion show encouraging results in biometric systems. However, most of them fails to handle the inherent uncertainty appropriately. To redress this shortcoming, a new fuzzy belief factor weighted evidence theory-based decision fusion (FBFE ve ) method is presented in this paper. Three feature extraction methods such as, FG-2DFLD, G2DLDA, Modular-LBP are employed to generate feature vector corresponding to a face image which are fed into the neural network to obtain belief factor. These belief factors based on different feature vectors are fused together to calculate a merit for each class. We have introduced a vanishing-penalty in terms of fuzzy weight, imposed on the missing winning class which is absent in the output set of anyone of three methods. These vanishing-penalty fuzzy weights are used in decision fusion-based face recognition. The proposed method is evaluated by means of two different classifiers namely, radial basis function (RBF) neural network, and support vector machines (SVM). We have validated our method on four benchmark datasets such as, AR, AT&T, FERET, and UMIST. The empirical results show that our FBFE ve method attains superior recognition accuracy over some classical sensor-, evidence theory and other related fusion-based techniques.
更多查看译文
关键词
Decision-level fusion,Fuzzy belief factor,Face recognition,Evidence theory,Vanish penalty weight
AI 理解论文
溯源树
样例
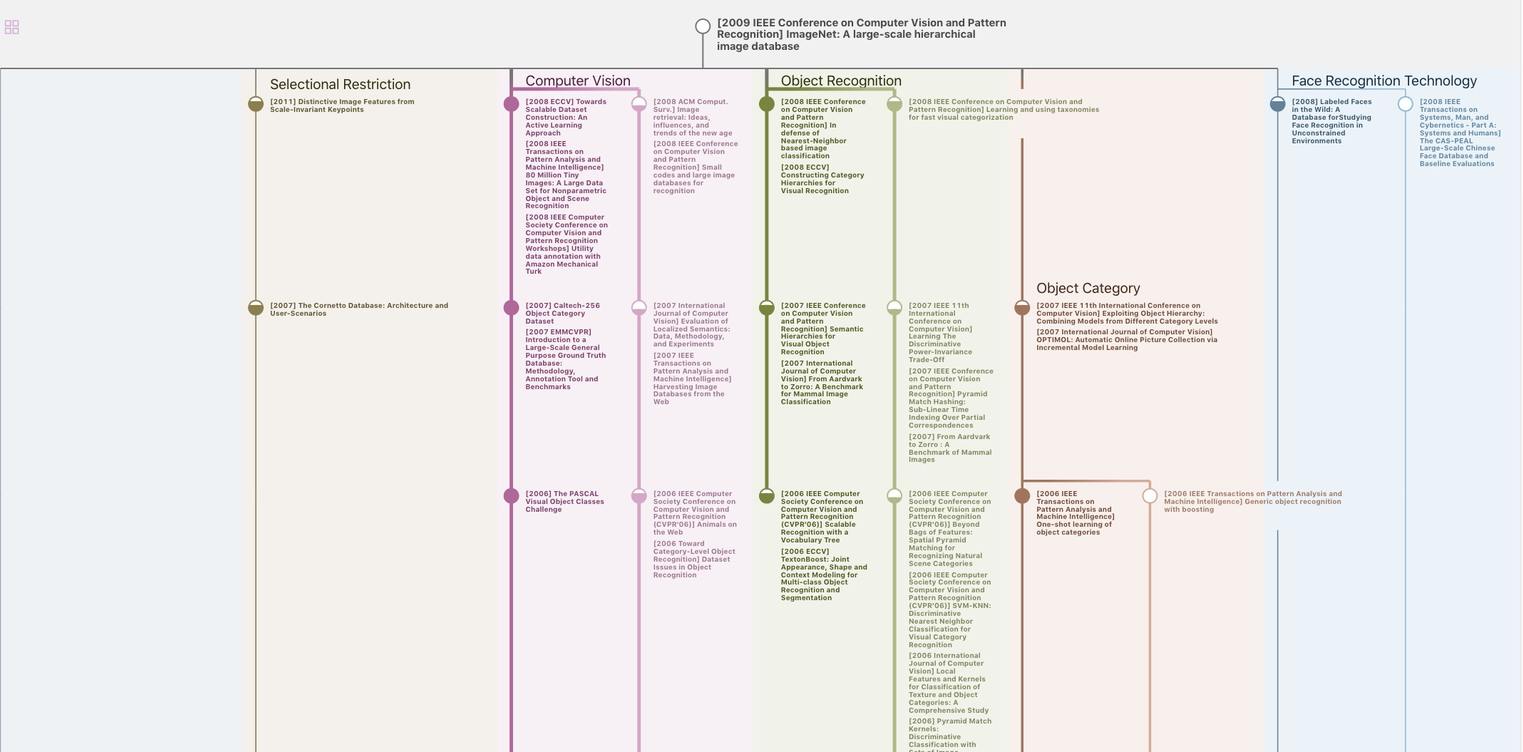
生成溯源树,研究论文发展脉络
Chat Paper
正在生成论文摘要