MIN: multi-dimensional interest network for click-through rate prediction
Knowl. Inf. Syst.(2023)
摘要
Click-through rate (CTR) prediction is a critical task in recommender systems and online advertising systems. The extensive collection of behavior data has become popular for building prediction models by capturing user interests from behavior sequences. There are two types of entities involved in behavior sequences, users and items, which form three kinds of relationships: user-to-user, user-to-item, and item-to-item. Most related work focuses on only one or two of these relationships, often ignoring the association between users, which also helps discover potential user interests. In this paper, we consider all three relationships useful and propose a Multi-dimensional Interest Network (MIN) to focus on their impact on CTR prediction simultaneously. It consists of three sub-networks that capture users’ preferences regarding group interests and individual interests. Specifically, the u-u sub-network models the relationship between the target user and those who have clicked on the target item. It takes user representations learned from behavior sequences via transformer as input. Two other sub-networks capture the individual interest of the target user. The u-i sub-network models the relationship between the target user and the target item. The i-i sub-network models the relationship between the target item and the items the target user has interacted with in the past time. Extensive evaluations on the real datasets show that our MIN model outperforms the state-of-the-art solutions in prediction accuracy ( + 5.0
更多查看译文
关键词
Click-through rate prediction,Sequential recommendation,Computational advertising,Interest network,Transformer
AI 理解论文
溯源树
样例
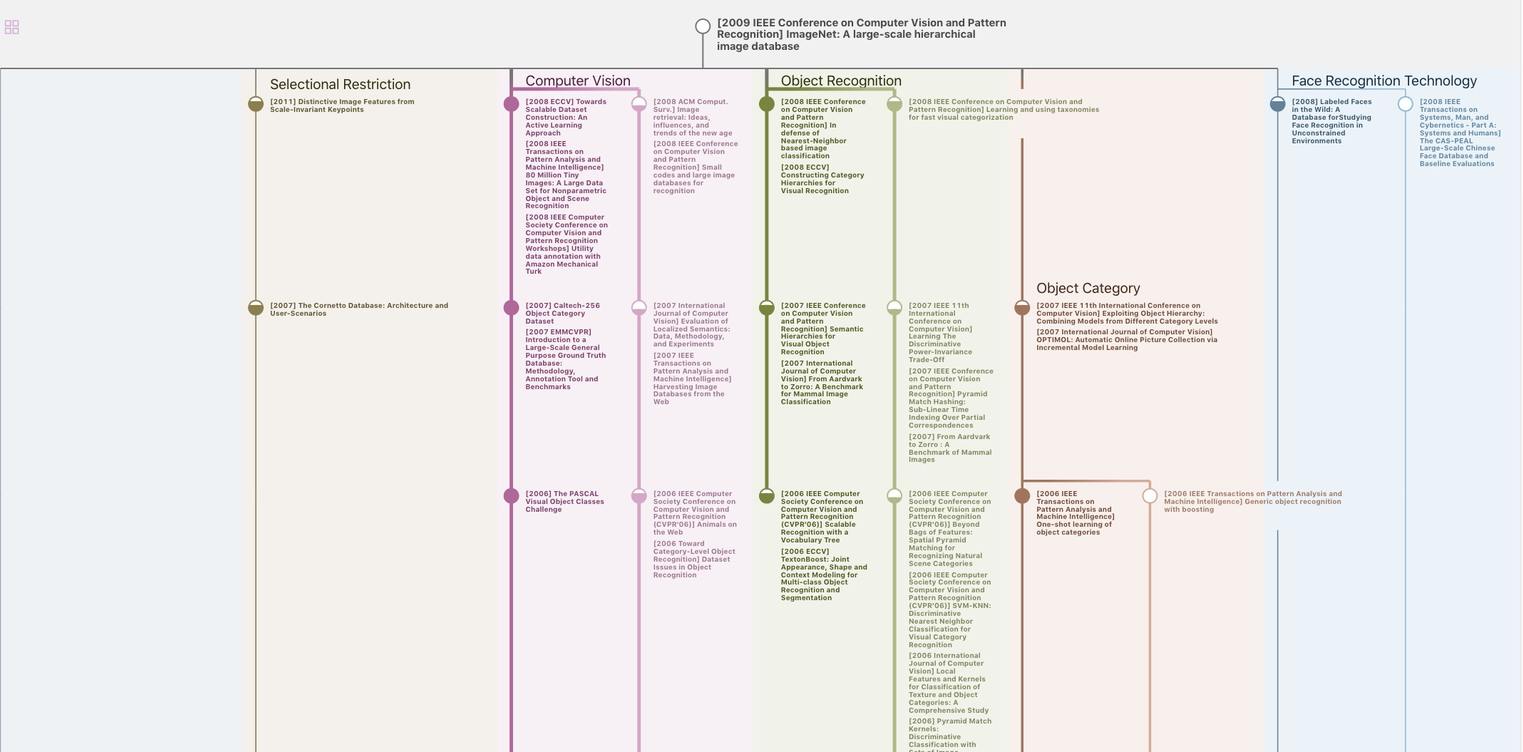
生成溯源树,研究论文发展脉络
Chat Paper
正在生成论文摘要