Bayesian learning via neural Schrödinger–Föllmer flows
Statistics and Computing(2022)
摘要
In this work we explore a new framework for approximate Bayesian inference in large datasets based on stochastic control. We advocate stochastic control as a finite time and low variance alternative to popular steady-state methods such as stochastic gradient Langevin dynamics. Furthermore, we discuss and adapt the existing theoretical guarantees of this framework and establish connections to already existing VI routines in SDE-based models.
更多查看译文
关键词
Schrödinger bridge problem,Föllmer drift,Stochastic control,Bayesian inference,Bayesian deep learning
AI 理解论文
溯源树
样例
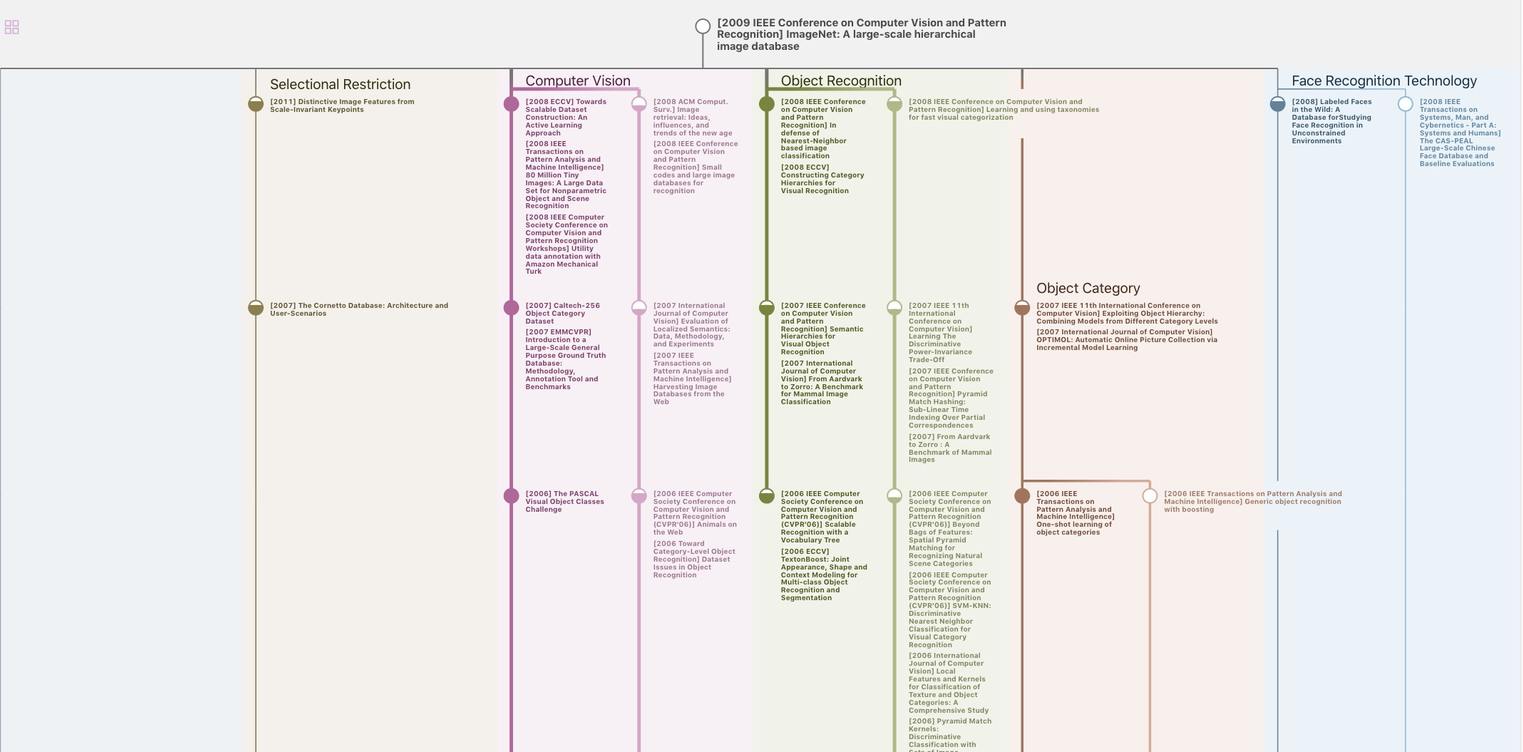
生成溯源树,研究论文发展脉络
Chat Paper
正在生成论文摘要