Deep-learning with context sensitive quantization and interpolation for underwater image compression and quality image restoration
International Journal of Information Technology(2022)
摘要
Reconstructed images from deep learning-based compressed images are not suitable for underwater image analysis. Hence, this paper proposes a compression framework by integrating deep learning and traditional techniques for underwater image compression to improve both the compression rate and quality of the restored image. Two asymmetric Convolutional Neural Networks (CNNs) are used in the proposed methodology namely Compact–CNN (C-CNN) and Residual Dense CNN (RD-CNN). C-CNN retains the structural information of input 128 × 128 × 3 data in 64 × 64 × 3 output image. Contrast Sensitivity Function (CSF) quantization-based JPEG applied on this 64 × 64 × 3 image, is used to increase the compression rate. At the receiver side, the decoded image is upsampled using bicubic interpolation and RD-CNN is used for image restoration. The proposed method provides better compression rates and reconstruction quality. An accuracy of 92.12% is obtained for classification of reconstructed fish images from the proposed model using transfer learning on Shufflenet.
更多查看译文
关键词
Underwater image compression,Convolutional Neural Networks,Contrast Sensitivity Function,JPEG,C-CNN,RD-CNN
AI 理解论文
溯源树
样例
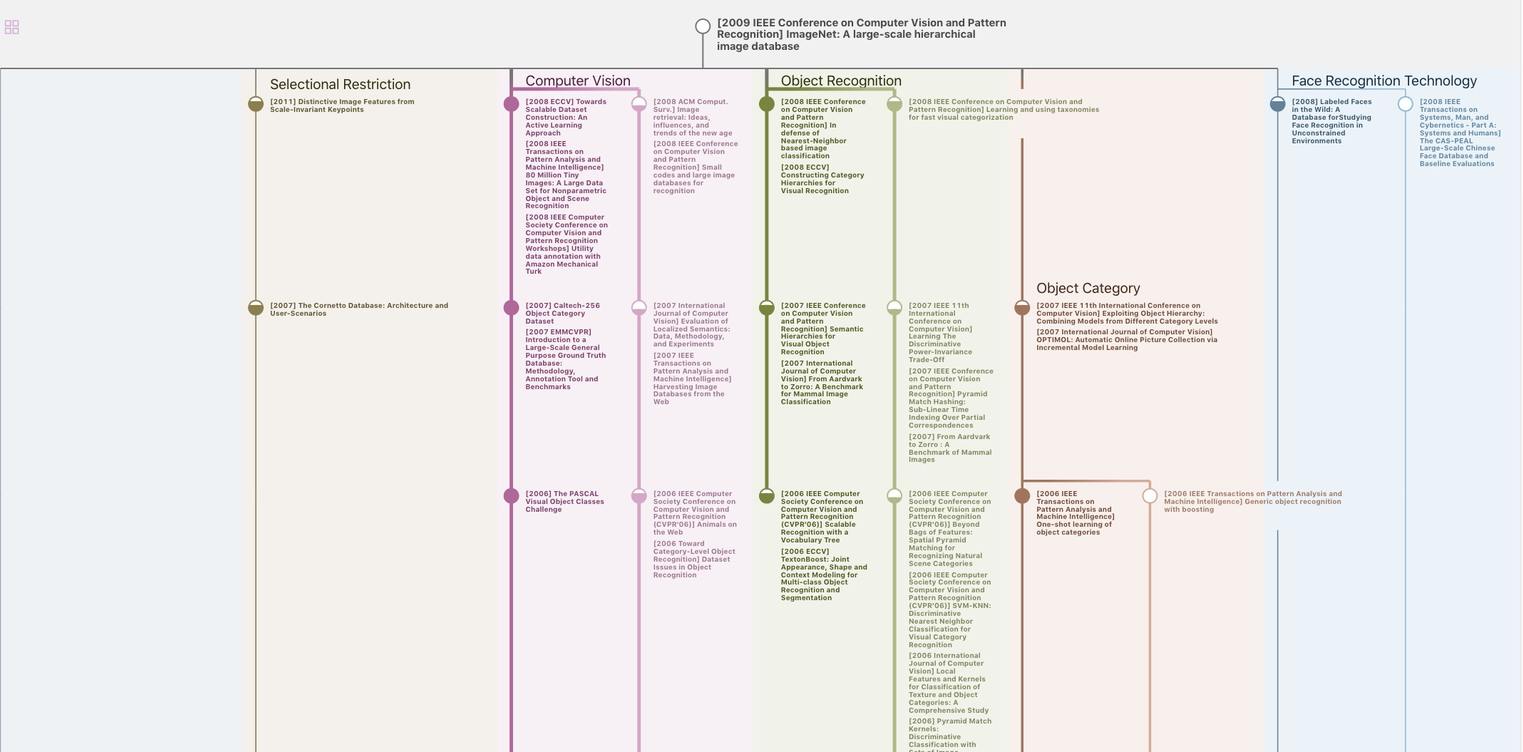
生成溯源树,研究论文发展脉络
Chat Paper
正在生成论文摘要