NC ^2 E: boosting few-shot learning with novel class center estimation
Neural Computing and Applications(2022)
摘要
urate class distribution estimation is expected to solve the problem of the poor generalization ability that exists in few-shot learning models due to data shortages. However, the reliability of class distributions estimates based on limited samples and knowledge is questionable, especially for similar classes. We find that the distribution calibration method is inaccurate in estimating similar classes due to limited knowledge being reused through double-validation experiments. To address this issue, we propose a novel class center estimation (NC ^2 E) method, which consists of a two-stage center estimation (TCE) algorithm and a class centroid estimation (CCE) algorithm. The class centers estimated by TCE in two stages are closer to the truth, and its superiority is demonstrated by error theory. CCE searches for the centroid of the base class iteratively and is used as the basis for the novel class calibration. Sufficient simulation samples are generated based on the estimated class distribution to augment the training data. The experimental results show that, compared with the distribution calibration method, the proposed method achieves an approximately 1
更多查看译文
关键词
Few-shot learning,Object recognition,Class distribution estimation,Similar class classification
AI 理解论文
溯源树
样例
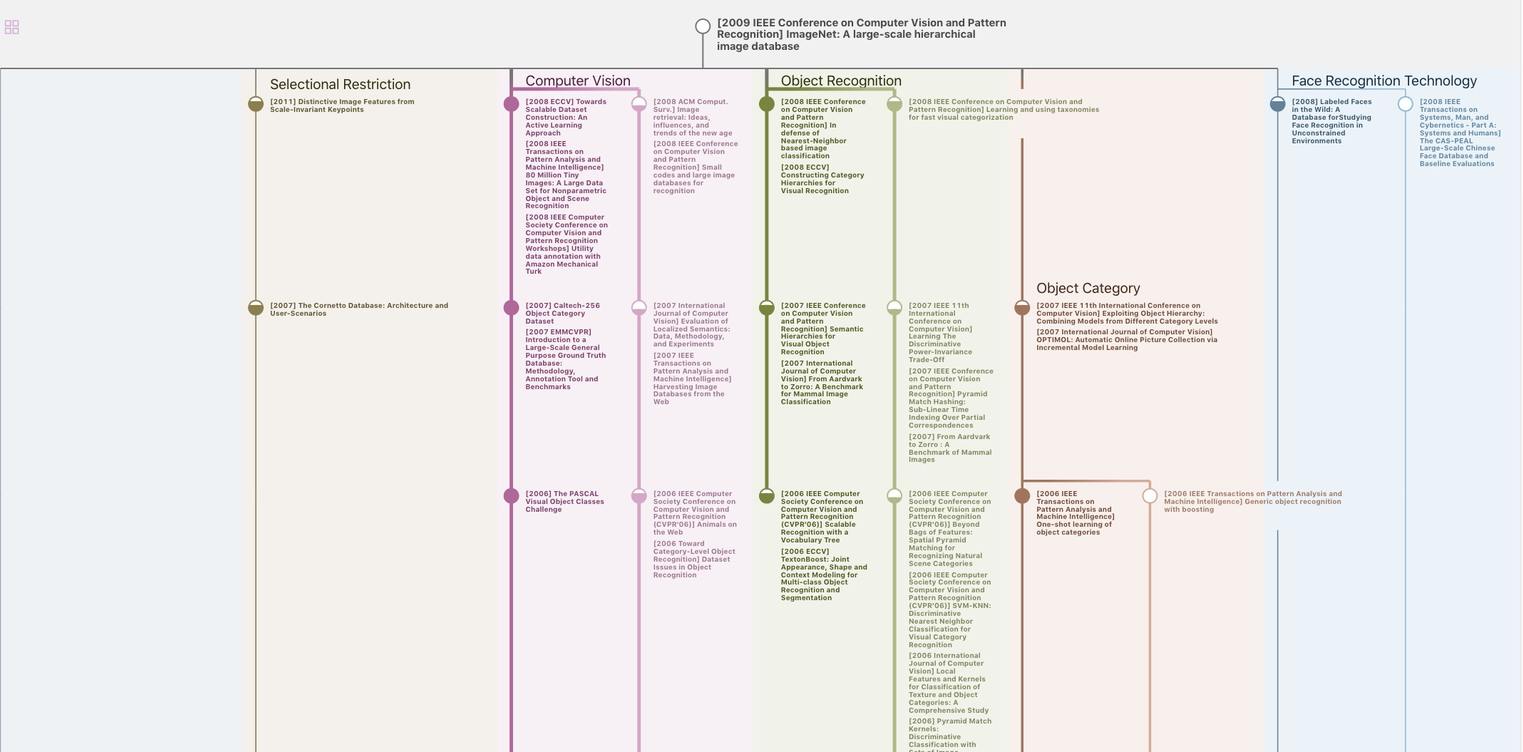
生成溯源树,研究论文发展脉络
Chat Paper
正在生成论文摘要