Hybridizing K-means clustering algorithm with harmony search and artificial bee colony optimizers for intelligence mineral prospectivity mapping
Earth Sci. Informatics(2023)
摘要
Unsupervised clustering methods are used as data-driven models for mapping of mineral prospectivity (MPM). One of the challenging problems for modeling high potential mineral prospects using these algorithms is to select proper cluster centroids in order to locate the same geospatial objects into reasonable clusters. To overcome this kind of problems arise from traditional clustering algorithms (e.g. K-means), the combination of harmony search (HS) and artificial bee colony (ABC) metaheuristic optimization algorithms with K-means have been firstly developed in this study which can be used for selection of optimum cluster centroids for highlighting favorable targets in prospecting stage of mineral explorations. This stage is critically important for exploration of undiscovered mineral deposits, because the time and cost are two substantial factors in regional-scale exploration programs. This study stated that the applying hybrid data-driven clustering models (e.g., HS-KM and ABC-KM) are operational tools for identifying high-favorable areas linked to porphyry Cu systems in prospecting stage. Moreover, the results demonstrated that the properly selection of geospatial exploration features has strong impact on the modeling of mineral prospectivity in Kerman porphyry belt, Iran and cause significant decrease in systemic uncertainty linked to MPM.
更多查看译文
关键词
K-means,Harmony search,Artificial bee colony,Optimization,MPM
AI 理解论文
溯源树
样例
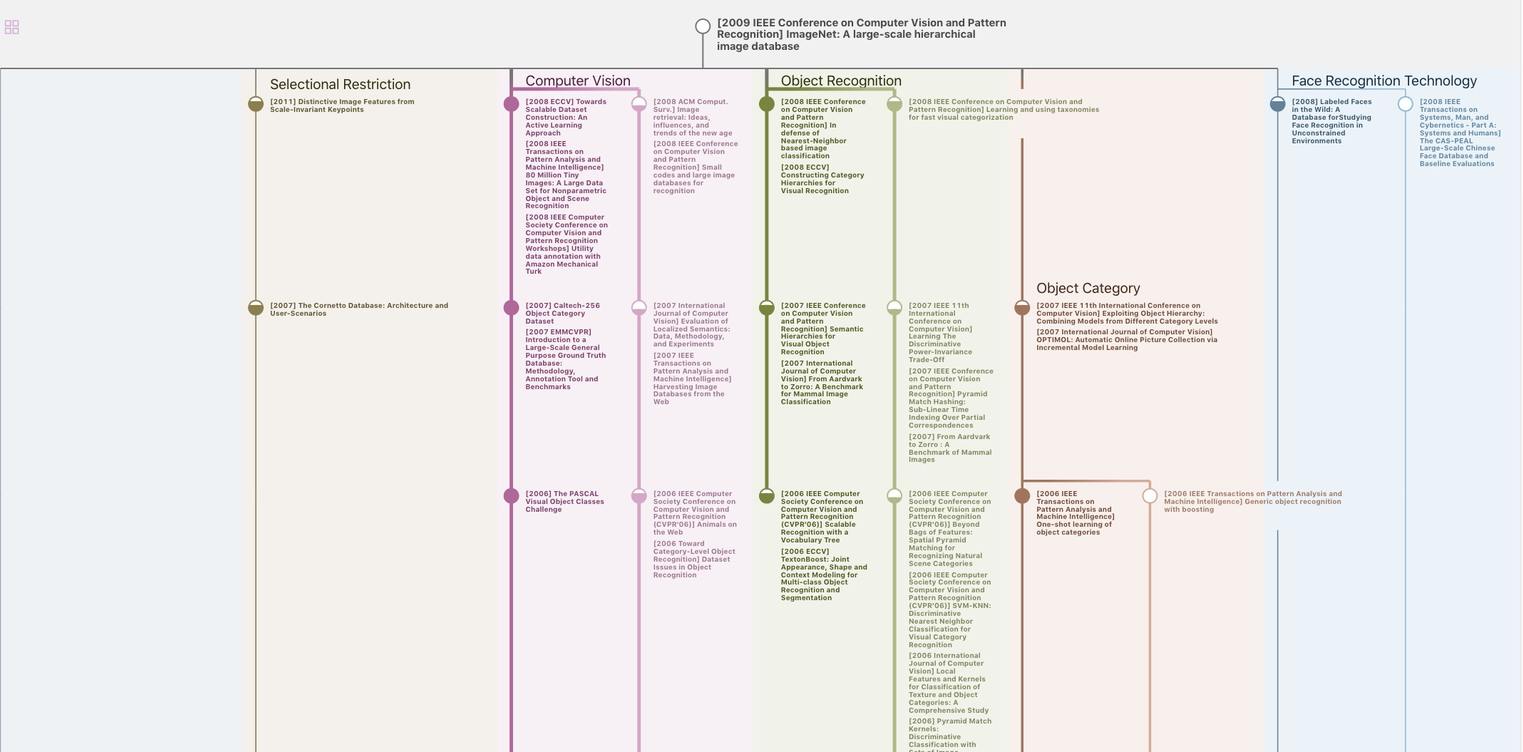
生成溯源树,研究论文发展脉络
Chat Paper
正在生成论文摘要