A new framework for intelligent fault diagnosis of spiral bevel gears with unbalanced data
Appl. Intell.(2023)
摘要
The special meshing motion and complex internal structure of spiral bevel gears make modeling the fault mechanism and extracting manual features challenging. Intelligent technology is expected to significantly improve the automation level of spiral bevel gear fault diagnosis. However, the lack of spiral bevel gear fault samples can result in degraded diagnostic performance. Existing solutions have often been developed at either the algorithm level or the data level, making it difficult to achieve high-quality feature extraction and high-precision pattern classification simultaneously. To overcome this deficiency, a novel framework is proposed for the intelligent fault diagnosis under imbalanced dataset. Firstly, an unsupervised learning neural network, deep convolutional auto-encoder (DCAE), is constructed to encode the data features, which modeled global and local information by combing global pooling and max pooling. Then during the fine-tuning stage of the classifier, we used an improved cross-entropy loss function to impose a greater misclassification cost on difficult and small samples, which can avoid overfitting of the features to healthy data features. By analyzing the experimental datasets of spiral bevel gears, it is demonstrated that the proposed framework has better performance in handing intelligent fault diagnosis tasks under the condition of unbalanced data than the reported frameworks.
更多查看译文
关键词
Spiral bevel gear,New fault diagnostic framework,Unbalanced data,Improved DCAE,Modified loss function
AI 理解论文
溯源树
样例
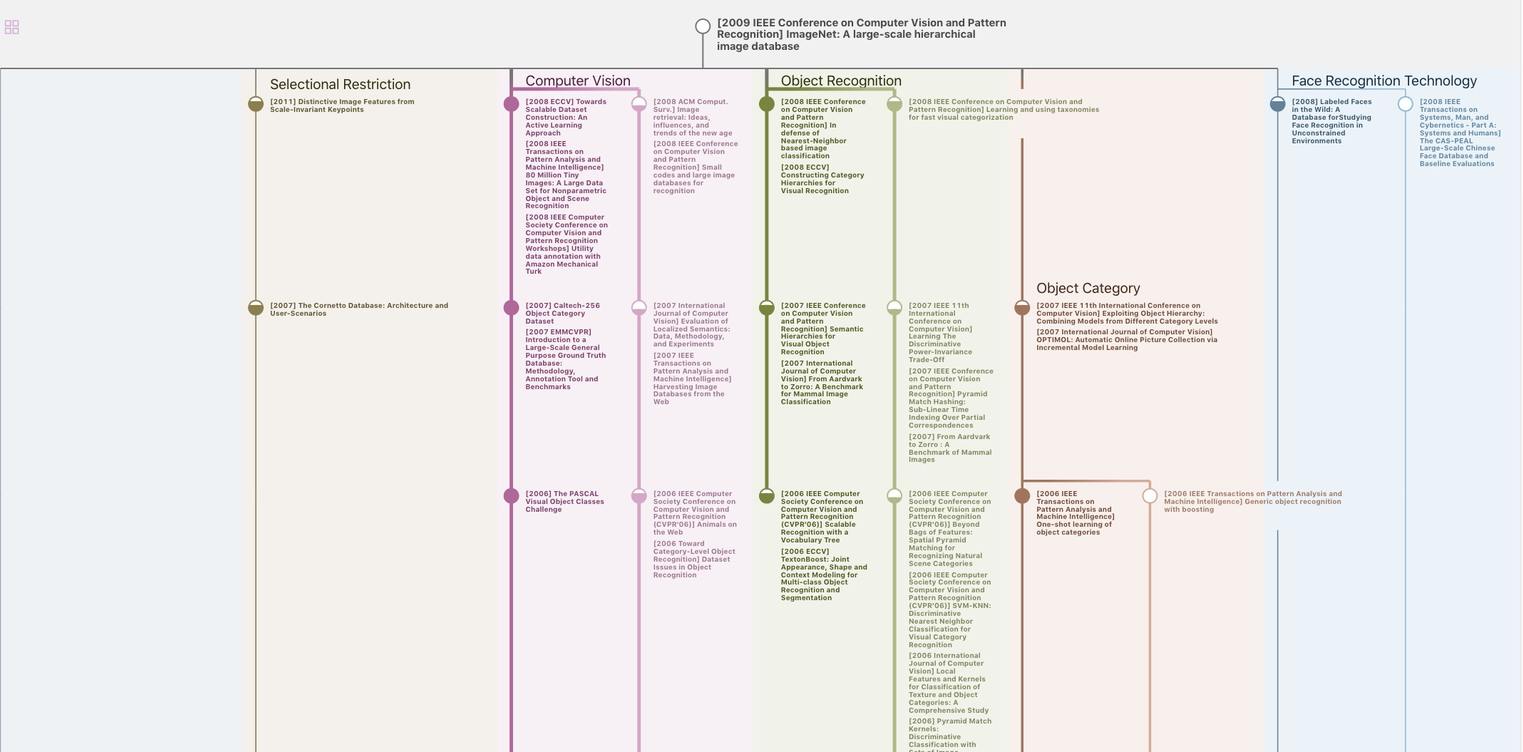
生成溯源树,研究论文发展脉络
Chat Paper
正在生成论文摘要