Fault Diagnosis of Tenessee Eastman Process with Detection Quality Using IMVOA with Hybrid DL Technique in IIOT
SN Comput. Sci.(2023)
摘要
This work presents a deep learning (DL) based approach to defect identification in continuous systems. The Tennessee Eastman Procedure was chosen as a use case for this study because it involves a dispersed network of sensors throughout a manufacturing facility. To improve detection quality for fault diagnosis, a hybrid DL approach is presented to choose the most representative sensors. Using an enhanced version of the Multi-Verse Optimization technique, the learning velocity of a Recurrent Neural Network is maximised (IMVOA). An exhaustive design space of solutions varying in sensing and detected quality has been made available by the suggested technique. It is an alternative to the standard Industry 4.0 setup, in which a plethora of decentralised sensor networks report their findings to a single cloud repository. In contrast, the suggested method takes a decentralised approach, which means that processing may occur closer to the sensors that create the data, or even at the advantage of the Internet of Things. Bandwidth, privacy, and latency issues, which often plague centralised methods, are circumvented here. Experimental results demonstrate that the suggested technique delivers fault finding solutions for the Tennessee Eastman Procedure at state-of-the-art detection quality levels. Solution times are 35 times faster than the application with the best detection quality, while feature counts are reduced by an average of 1.99 times. It is important to note that the framework’s scalability creates a design space from which the best possible implementation may be selected based on the requirements of the application.
更多查看译文
关键词
Tennessee Eastman process,Fault diagnosis,Deep learning,Improved multi-verse optimization algorithm,Recurrent Neural Network
AI 理解论文
溯源树
样例
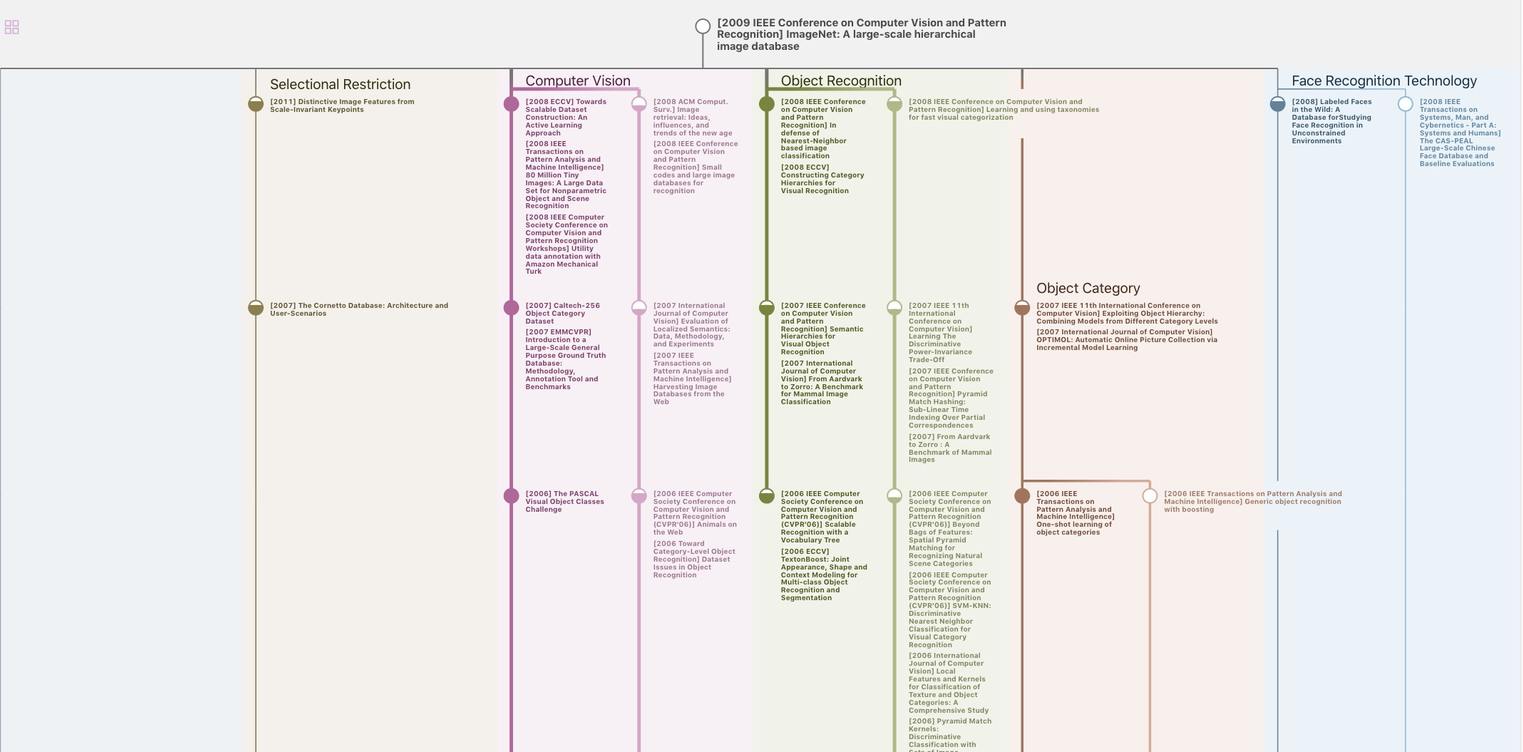
生成溯源树,研究论文发展脉络
Chat Paper
正在生成论文摘要