Deep Learning-Based Clinical Wound Image Analysis Using a Mask R-CNN Architecture
Journal of Medical and Biological Engineering(2023)
摘要
Purpose The purpose of this study is to address the lack of research on utilizing deep learning for the detection of peripheral artery disease (PAD) wounds. The main objective is to present a novel solution for automatic wound segmentation and detection using the Mask R-CNN framework. Methods The study utilizes a dataset of 3329 clinical wound images, which includes wounds in patients with PAD as well as general trauma. The Mask R-CNN framework is implemented to detect and differentiate wounds. Results The results of our approach are remarkable, achieving an Intersection over Union score of 0.69, recall of 0.72, precision of 0.77, F1 score of 0.75, and average precision of 0.71. These metrics demonstrate the accuracy and effectiveness of our proposed framework for wound segmentation and diagnosis. Conclusion Our innovative approach utilizing the Mask R-CNN framework provides an accurate and efficient solution for the detection and classification of wounds, specifically in patients with PAD. The results highlight the practical applicability of our framework and its potential to improve the clinical healthcare of patients dealing with chronic wounds. This study represents a significant step forward in addressing the challenges of PAD and chronic wounds, opening up exciting possibilities for future research in this field.
更多查看译文
关键词
clinical wound image analysis,learning-based,r-cnn
AI 理解论文
溯源树
样例
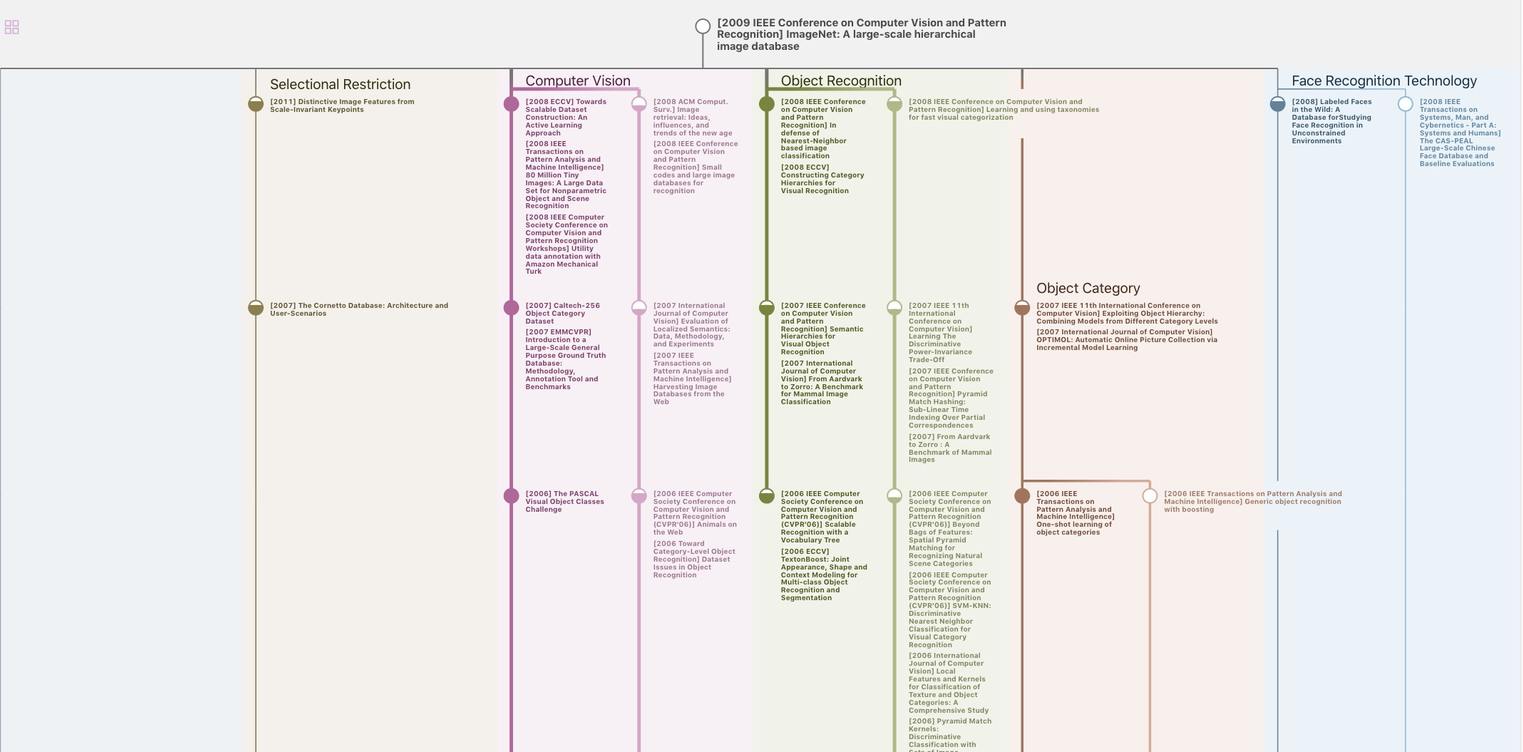
生成溯源树,研究论文发展脉络
Chat Paper
正在生成论文摘要