A novel adaptive two-stage selection strategy in local binary pattern for texture classification
Signal Image Video Process.(2023)
摘要
Local binary pattern (LBP) is widely used in texture classification fields because of its low computational cost and invariance to environmental changes. There are two essential steps in LBP: the texture feature extraction step and the texture feature classification step. However, in the texture feature extraction step, all existing LBP-based methods with fixed sampling radius R cannot obtain multi-scale texture features. Furthermore, at present, the texture feature classification step cannot efficiently use multi-scale texture features as well. To overcome these two main drawbacks, we propose a novel adaptive two-stage selection strategy in local binary pattern. There are totally three steps in our proposed adaptive two-stage selection (ATSS) strategy: the preprocessing step, the adaptive first-stage selection step and the second-stage selection step. In the preprocessing step, the ATSS strategy uses Gaussian kernel to obtain down-sampled multi-scale texture images. In the adaptive first-stage selection step, the ATSS strategy uses the low-complexity original LBP to off-line extract a small number of large-scale texture features from down-sampled texture images. The top T training images which have more similar large-scale texture features with the testing image are adaptively selected to go to the next step. In the second-stage selection step, the ATSS strategy uses the original LBP and LBP-based variants separately to off-line extract a large number of small-scale texture features from the original testing images and the selected top T original training images. Hence, the finally selected top 1 training image has most similar both small-scale and large-scale texture features with the testing image. Comparing with original LBP-based methods, after introducing our adaptive two-stage selection (ATSS) strategy, the training images with only similar small-scale texture structures but different large-scale texture structures can be excluded after the adaptive first-stage selection step. Hence, the classification accuracy of LBP-based methods can be significantly improved. Furthermore, it is worth noting that our proposed adaptive two-stage selection (ATSS) strategy can be straightforwardly utilized in any other LBP-based variants to enhance their classification performance. Extensive experiments are conducted on four standard texture databases, Outex, UIUC, CUReT and XU_HR. The experimental results of seven representative LBP-based methods, LBP, LTP, CLBP, BRINT, CLBC, LNDP and CMPE show that our proposed ATSS strategy can significantly improve their classification accuracy and robustness against noise corruption.
更多查看译文
关键词
Local binary pattern (LBP),Texture classification,Adaptive two-stage selection (ATSS) strategy,Multi-scale feature,Noise robustness
AI 理解论文
溯源树
样例
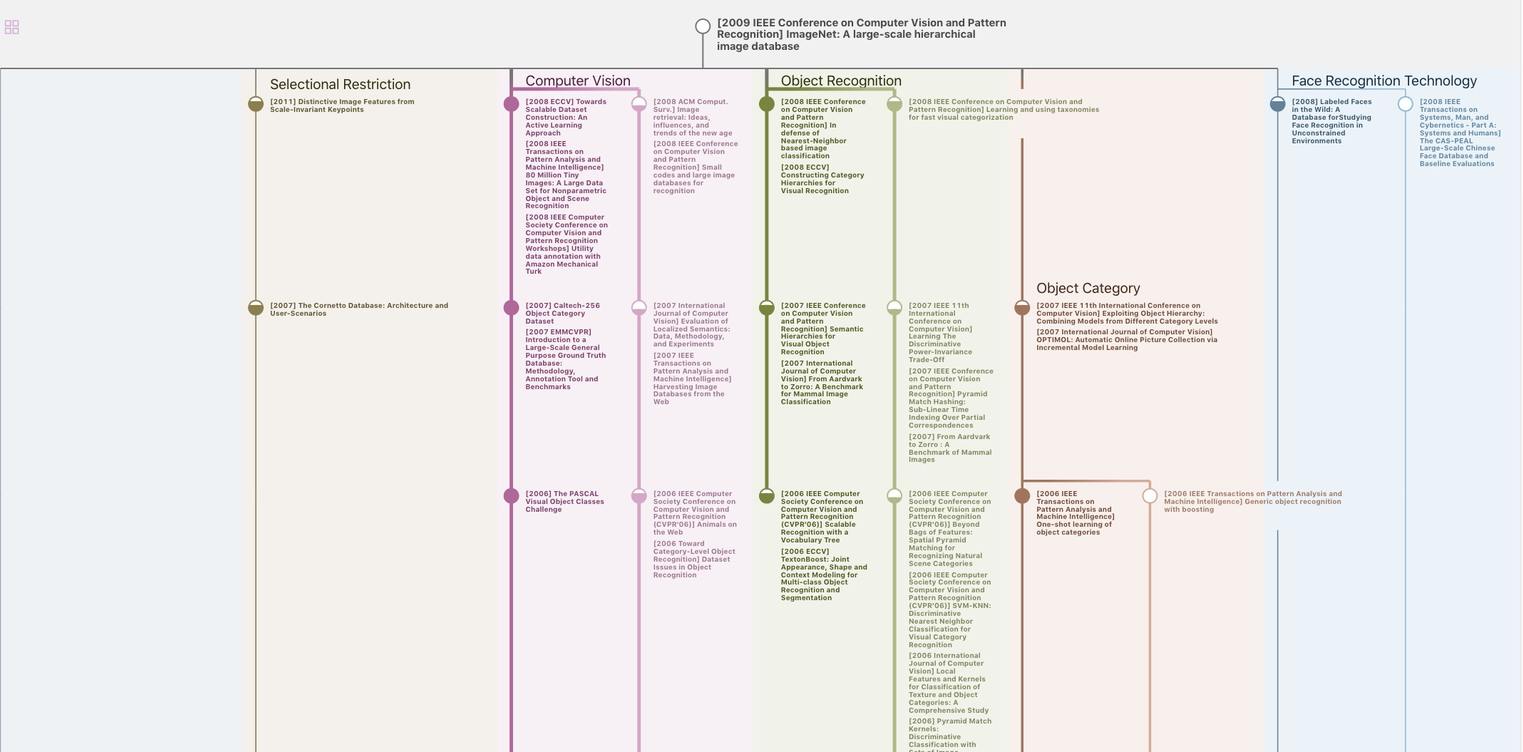
生成溯源树,研究论文发展脉络
Chat Paper
正在生成论文摘要