Semantic Segmentation of Satellite Images for Crop Type Identification in Smallholder Farms
The Journal of Supercomputing(2023)
摘要
Accurate and reliable crop type identification from satellite images provides a foundation for crop yield predictions which paves the way to help ensure food security. Most of the work done in the field of crop type mapping using remote sensing is restricted to the developed countries having large field parcels, while a little effort has been directed towards doing so for developing countries, where this task becomes more challenging due to the small size of field parcels, irregular shapes of the fields, and an acute shortage of labelled datasets for training supervised machine learning models. In this research, we try to fill this gap in the literature by exploring the feasibility of performing the semantic segmentation of agricultural fields from satellite images by proposing an encoder–decoder-based semantic segmentation architecture, CropNet, with a ResNet network as the encoder backbone and the use of attention modules in the decoder to allow the model to focus on more important portions of the feature maps and the feature fusion to concatenate the feature maps from all the decoder nodes getting a more precise prediction by bringing the spatial location information from the previous layers. The architecture outperformed the state of the art by 0.51
更多查看译文
关键词
Semantic segmentation,Remote sensing,Encoder–decoder architecture,Sentinel-2,Crop type mapping,Smallholder farms
AI 理解论文
溯源树
样例
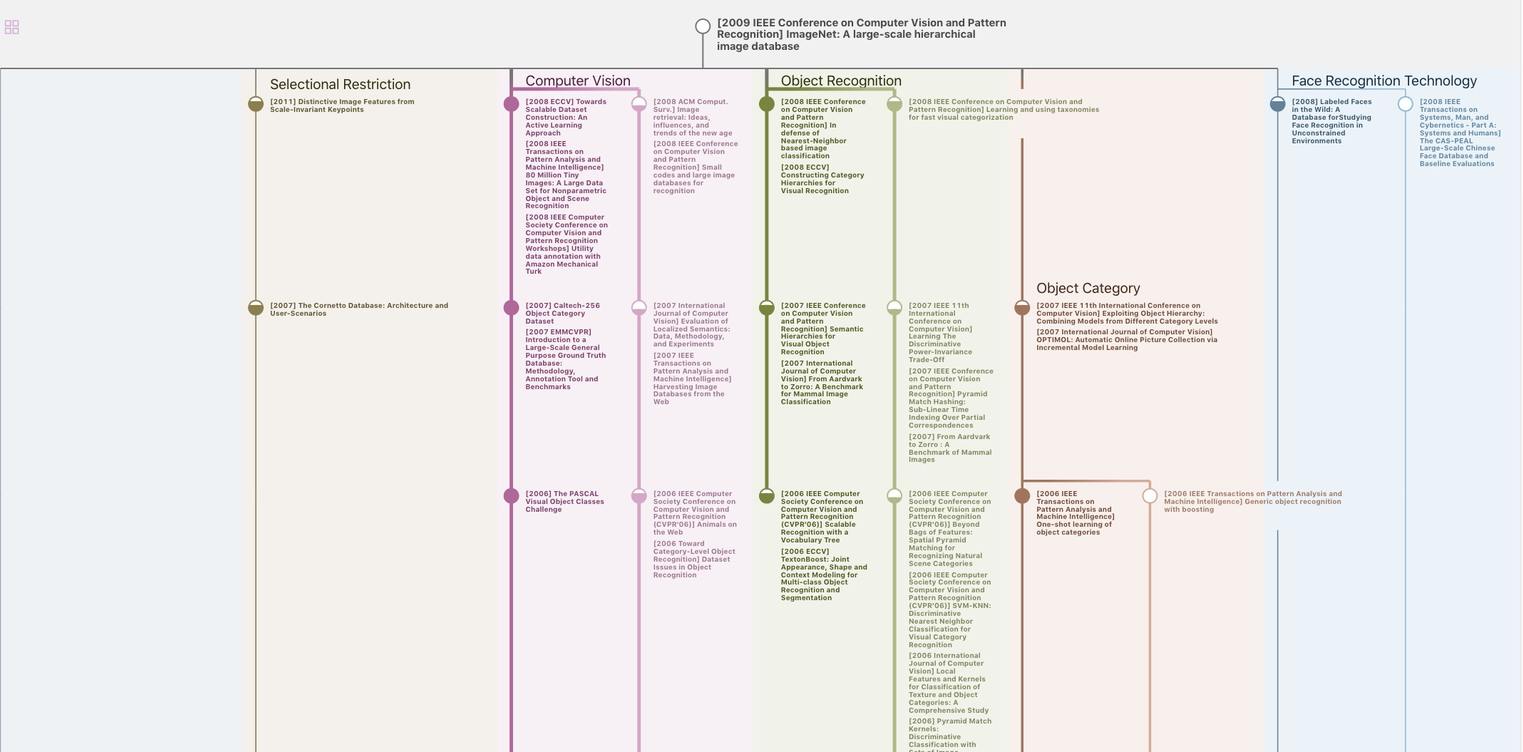
生成溯源树,研究论文发展脉络
Chat Paper
正在生成论文摘要