Gradient leakage attacks in federated learning
ARTIFICIAL INTELLIGENCE REVIEW(2023)
摘要
Federated Learning (FL) improves the privacy of local training data by exchanging model updates (e.g., local gradients or updated parameters). Gradients and weights of the model have been presumed to be safe for delivery. Nevertheless, some studies have shown that gradient leakage attacks can reconstruct the input images at the pixel level, which belong to deep leakage. In addition, well understanding gradient leakage attacks are beneficial to model inversion attacks. Furthermore, gradient leakage attacks can be performed in a covert way, which does not hamper the training performance. It is significant to study gradient leakage attacks deeply. In this paper, a systematic literature review on gradient leakage attacks and privacy protection strategies. Through carefully screening, existing works about gradient leakage attacks can be categorized into three groups: (i) bias attacks, (ii) optimization-based attacks, and (iii) linear equation solver attacks. We propose one privacy attack system, i.e., single-sample reconstruction attack system (SSRAS). Furthermore, rank analysis index (RA-I) can be introduced to provide an overall estimate of the security of the neural network. In addition, we propose an Improved R-GAP Algorithm, this improved algorithm can carry out image reconstruction regardless of whether the label can be determined. Finally, experimental results show the superiority of the attack system over some other state-of-the-art attack algorithms.
更多查看译文
关键词
Security and privacy,Federated Learning,Data reconstruction attack,Gradient leakage attack,Data privacy
AI 理解论文
溯源树
样例
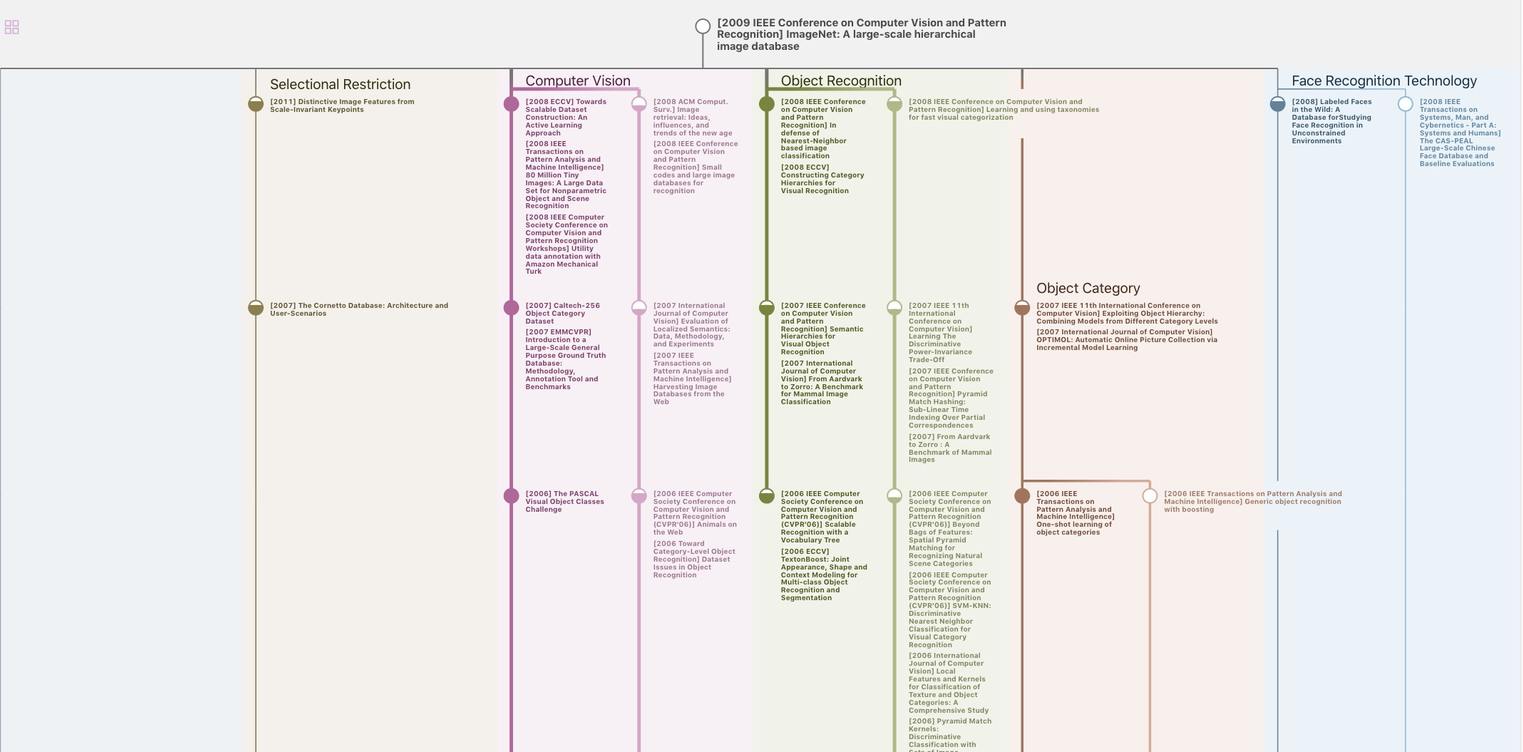
生成溯源树,研究论文发展脉络
Chat Paper
正在生成论文摘要