Deep transition network with gating mechanism for multivariate time series forecasting
APPLIED INTELLIGENCE(2023)
摘要
As an essential task in the machine learning community, multivariate time series forecasting has many real-world applications, such as PM2.5 forecasting, electricity price forecasting, and traffic flow forecasting. In multivariate time series data, one feature is chosen as the target feature and the other is seen as auxiliary information. These features have very complex spatial dependencies, and most of the existing models put all features together for spatial feature extraction, which is an undifferentiated feature extraction process. It can not distinguish the importance of different features to the target feature. Thus, the spatial feature extraction of data is insufficient. To solve the problems mentioned above, based on the Gated Recurrent Neural Network, we design a new architecture named Auxiliary Information-GRU (AI-GRU) to extract deep temporal and spatial features and combined it into Auxiliary Information-deep Transition Network (Al-DTN). AI-GRU added three gating units, auxiliary information gate controls the amount of auxiliary information flowing into AI-DTN, fusion information gate controls the degree of fusion of target feature and auxiliary information, linear transformation gate isolates the target features from the auxiliary information. Experimental results show that our proposed model outperforms the representative baseline models on the two benchmark datasets.
更多查看译文
关键词
Multivariate time series forecasting,Spatial feature extraction,Gated recurrent unit,Rurrent neural network
AI 理解论文
溯源树
样例
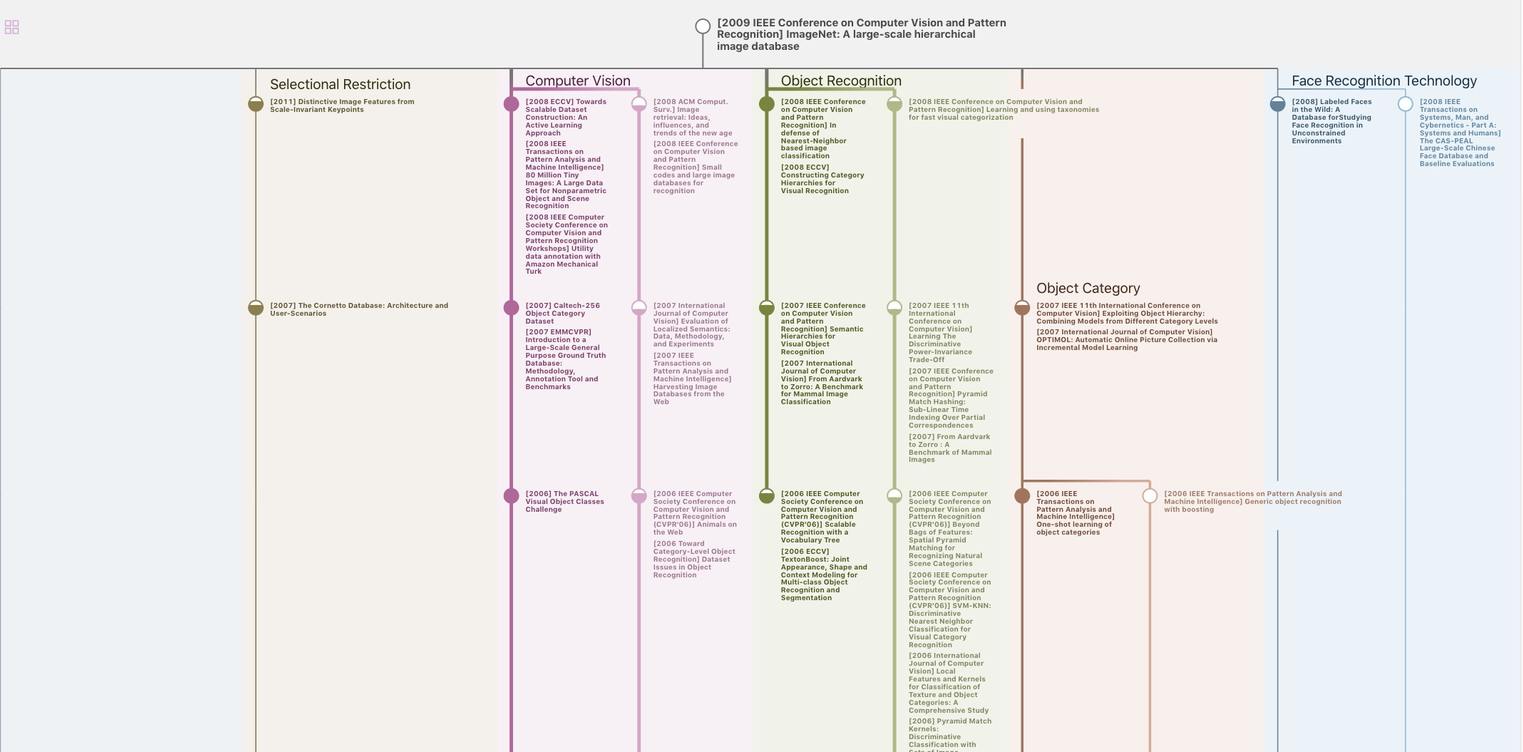
生成溯源树,研究论文发展脉络
Chat Paper
正在生成论文摘要