SLIM: A transparent structurized self-learning interpolation method for super-resolution images
VISUAL COMPUTER(2023)
摘要
Image super-resolution (SR) is a classic problem of image processing. This paper proposes a self-learning interpolation method (SLIM) based on a single image by combining grid feature mapping with binary decision tree, which is not only transparent as the interpolation-based methods, but also achieves comparable performance as the learning-based methods. Firstly, it downsamples the given image I^LR to obtain its low–low-resolution image I^LLR , which is used to obtain sample data for the self-learning interpolation algorithm for enlarging I^LLR to get I^LR . Secondly, it provides a structural feature classification method to divide all of the samples into several groups, such that each class of I^LLR is mapped to a matrix of coefficients for calculating the values of the pixels of I^LR . The image I^LR is approximated by executing the decision tree to refine the corresponding mapping matrix. Finally, the resulting high-resolution image I^HR is obtained from the given image I^LR by using the mapping matrixes. Experimental results show that SLIM achieves more smooth edges and better details on subjective vision than prevailing SR methods, and it is a transparent one but achieves comparable performances on PSNR and SSIM with the learning-based methods, while it outperforms the interpolation-based methods. It means that SLIM is both transparent and efficient and has much better subjective vision than other SR methods.
更多查看译文
关键词
Image interpolation,Self-learning,Classification,Structure features,Decision tree
AI 理解论文
溯源树
样例
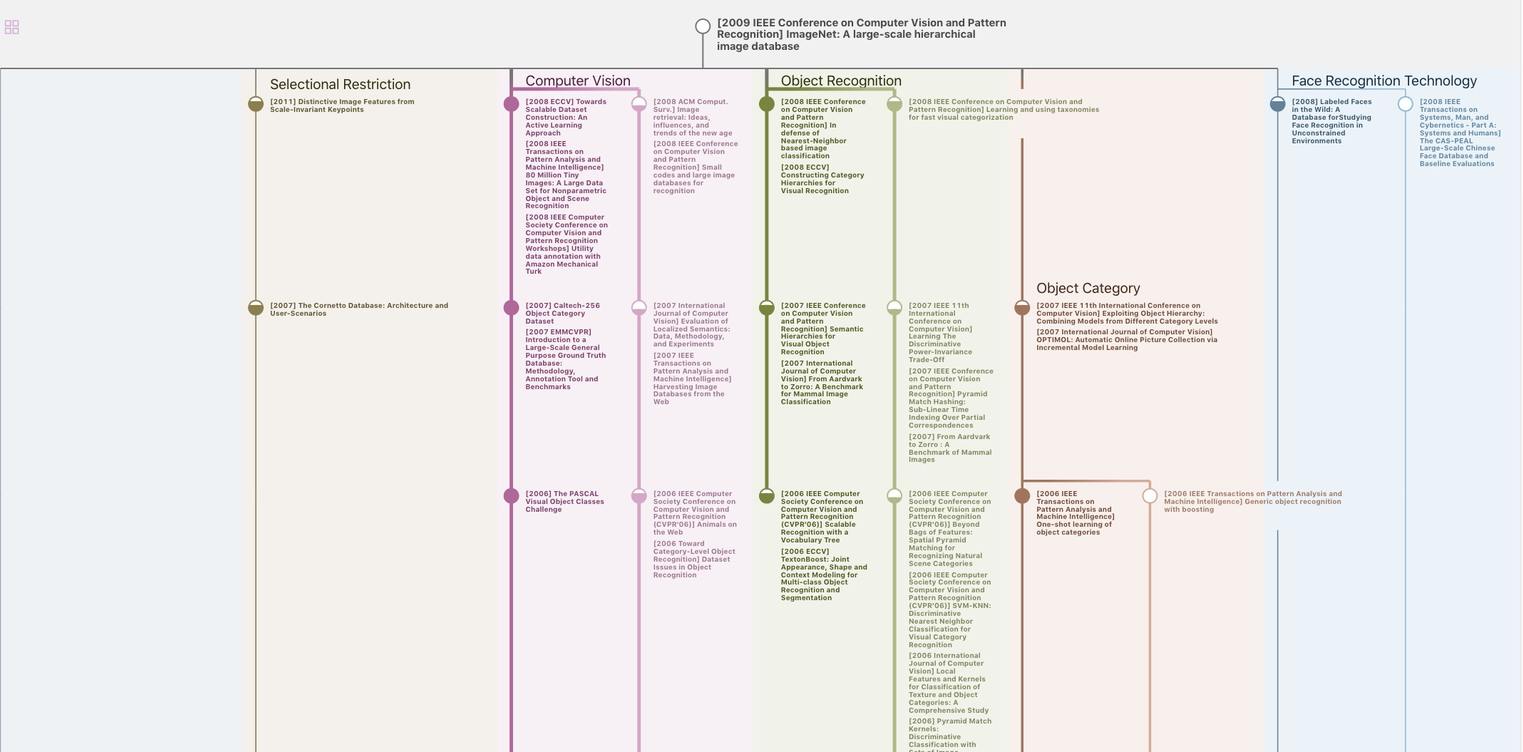
生成溯源树,研究论文发展脉络
Chat Paper
正在生成论文摘要