Using random forest and multiple-regression models to predict changes in surface runoff and soil erosion after prescribed fire
MODELING EARTH SYSTEMS AND ENVIRONMENT(2023)
摘要
Prescribed fire is a viable practice to reduce the wildfire risk in forests, but its application may lead to increased surface runoff and soil erosion. Several hydrological and erosive models have been proposed and evaluated to predict the changes in soil hydrology and erosion after prescribed fire. However, the prediction capacity of machine learning and Multiple-Regression models has scarcely been studied in sites treated with prescribed fire, despite of the usefulness of these tools for landscape planners. This study aims to evaluate how a Random Forest, RF, algorithm and Multiple-Regression, MR, and Partial Least Square Regression, PLS-R, equations can predict changes in surface runoff and soil erosion after prescribed fire. This prediction capacity has been quantified through the application of the models to 35 case studies reported in 18 academic papers selected from the international scientific literature. The model predictions have been evaluated using common statistics and indexes (e.g., the coefficient of Nash and Sutcliffe, NSE). The results show poor performance of the RF and PLS-R models in predicting runoff (NSE < 0 and < 0.31). However, the models' capacity to predict soil erosion was acceptable (NSE = 0.47 and 0.69, respectively). The predictions by the MR equation were also acceptable for runoff (NSE < 0.69) and good for erosion (NSE = 0.80). Furthermore, the MR equation offers a large applicability, since this simple model has been tested using a database of hydrological observations in environments with different characteristics. The performance of MR equations is encouraging when its broader use in runoff and erosion predictions in soils treated with prescribed fire.
更多查看译文
关键词
Soil hydrology,Wildfire risk,Machine learning,Regression analysis,Hydrological modelling
AI 理解论文
溯源树
样例
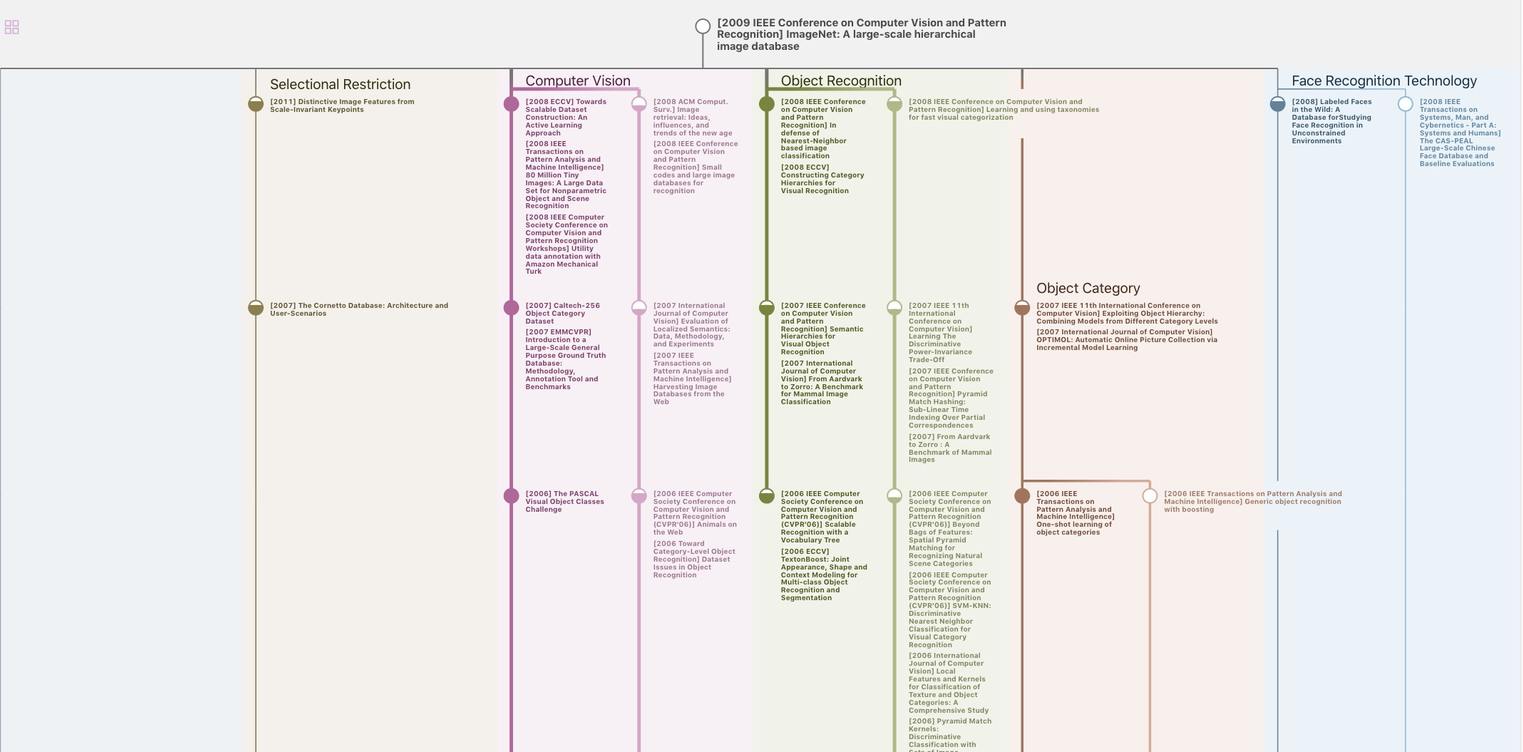
生成溯源树,研究论文发展脉络
Chat Paper
正在生成论文摘要