Enhancing the performance of tunnel water inflow prediction using Random Forest optimized by Grey Wolf Optimizer
Earth Sci. Informatics(2023)
摘要
In this research, a groundbreaking intelligent model named the GWO-RF model is introduced for the prediction of water inflow (WI) during tunnel construction. WI is a prevalent and intricate occurrence that significantly jeopardizes the safety of workers and equipment. The proposed model aims to address this issue by leveraging the capabilities of Grey Wolf Optimization (GWO) combined with the Random Forest (RF) algorithm. Through this innovative approach, the study strives to enhance the accuracy and effectiveness of WI prediction, ultimately contributing to improved safety measures in tunnel construction projects. The GWO-RF model utilizes 600 sets of data comprising tunnel depth (H), groundwater level (h), rock quality designation (RQD), and water yield property (W) as input independent variables. To evaluate the predictive performance of the GWO-RF model, seven ensemble regression models, namely RF, Bagging, AdaBoost, HistGradientBoosting (HGBoosting), GradientBoostingRegressorTree (GBRT), Voting, and Stacking are compared. Four prediction accuracy criteria were used to evaluate the performance of the developed models. The significance of variables and their contribution to WI prediction are analyzed using the Shapley Additive Explanations (SHAP) approach. The evaluation results demonstrate the superior accuracy of the proposed GWO-RF model over other conventional ensemble models in both the training and testing phases. With R 2 values of 0.9483 and 0.9289, MAE values of 4.6042 and 8.3862, MSE values of 68.5806 and 144.1149, and RMSE values of 8.2813 and 12.0047, respectively, the GWO-RF model outperforms the others. As a result, the proposed GWO-RF model proves highly effective in predicting WI and determining the corresponding pumping requirements, making it invaluable for tunneling projects.
更多查看译文
关键词
Water inflow into tunnel,Random forest,Grey Wolf optimizer,Shapley Additive Explanation
AI 理解论文
溯源树
样例
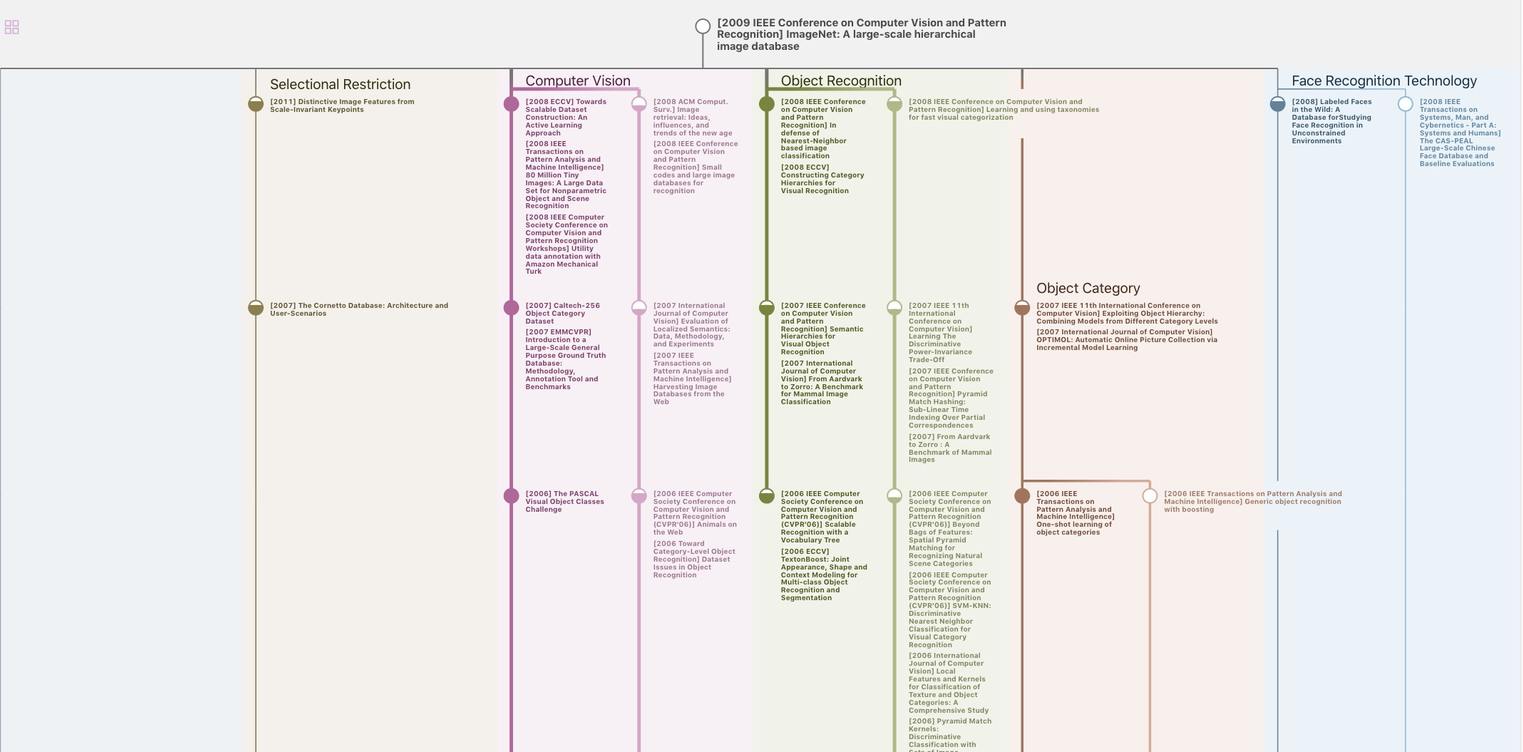
生成溯源树,研究论文发展脉络
Chat Paper
正在生成论文摘要