Hybrid Neural Network - Variational Data Assimilation algorithm to infer river discharges from SWOT-like data
Computational Geosciences(2023)
摘要
Estimating discharges Q ( x , t ) from altimetric measurements only, for ungauged rivers (in particular, those with unknown bathymetry b(x)), is an ill-posed inverse problem. We develop here an algorithm to estimate Q ( x , t ) without prior flow information other than global open datasets. Additionally, the ill-posedness feature of this inverse problem is re-investigated. Inversions based on a Variational Data Assimilation (VDA) approach enable accurate estimation of spatio-temporal variations of the discharge, but with a bias scaling the overall estimate. This key issue, which was already highlighted in our previous studies, is partly solved by considering additional hydrological information (the drainage area, A (km^2) ) combined with a Machine Learning (ML) technique. Purely data-driven estimations obtained from an Artificial Neural Network (ANN) provide a reasonably good estimation at a large scale ( ≈ 10^3 m). This first estimation is then employed to define the first guess of an iterative VDA algorithm. The latter relies on the Saint-Venant flow model and aims to compute the complete unknowns (discharge Q ( x , t ), bathymetry b ( x ), friction coefficient K ( x , t )) at a fine scale (approximately 10^2 m). The resulting complete inversion algorithm is called the H2iVDI algorithm for "Hybrid Hierarchical Variational Discharge Inference". Numerical experiments have been analyzed for 29 heterogeneous worldwide river portions. The obtained estimations present an overall bias (less than 30
更多查看译文
关键词
Data assimilation,Neural network,Inference,Rivers,Discharge,Bathymetry,Altimetry,Datasets,SWOT mission.
AI 理解论文
溯源树
样例
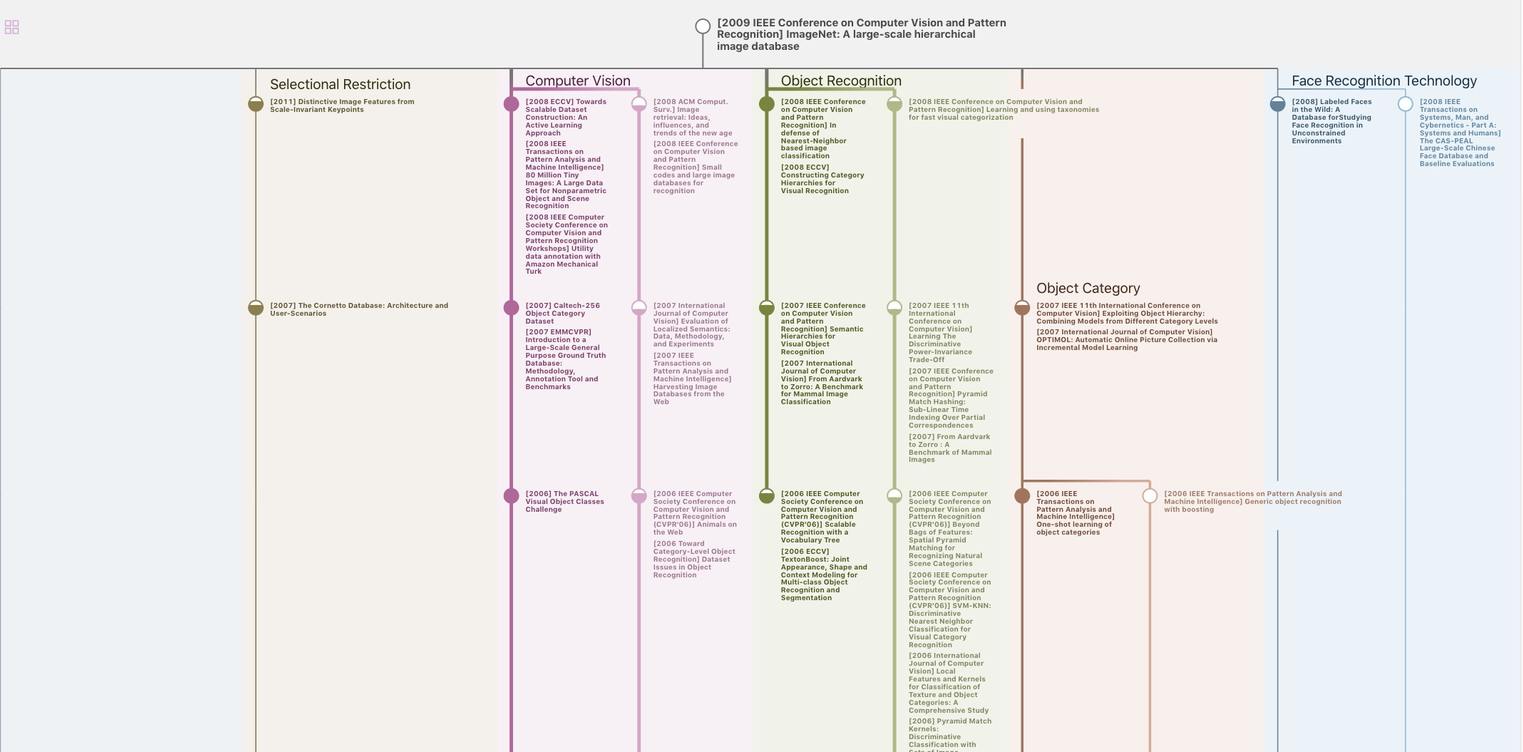
生成溯源树,研究论文发展脉络
Chat Paper
正在生成论文摘要