Multi-objective gradient-based intelligent optimization of ultra-high-strength galvanized TRIP steels
INTERNATIONAL JOURNAL OF ADVANCED MANUFACTURING TECHNOLOGY(2023)
摘要
In this paper, a novel gradient-based algorithm named Kernel-based hybrid multi-objective optimization (KHMO) is implemented and coupled with a support vector regression (SVR) model to efficiently optimize the production of a cold rolled hot-dip galvanized TRIP steel. For this purpose, several heat treatments using an isothermal bainitic transformation (IBT) temperature compatible with continuous hot-dip galvanizing were performed. The most significant processing parameters (cooling rate after intercritical austenitizing ( CR_1 ), isothermal holding time at the galvanizing temperature in the bainitic region t_2 , and last cooling rate to room temperature ( CR_2 )) were thus optimized to achieve the required mechanical properties values. In general, SVR model fits in a satisfactory manner the highly non-linear relationship between experimental parameters and resulting mechanical properties; hence, it is used as objective function. Besides, KHMO algorithm reveals an outstanding performance since it found a dense and spread Pareto front. Moreover, the processing window to manufacture TRIP-aided martensitic steels is suggested in a range of 57–63 ^∘ C/s, 33–37 s, and 1–2 ^∘ C/s for CR_1 , t_2 , and CR_2 , respectively. The developed computational methodology for modeling and optimization of operating parameters is successfully applied for the first time in the experimental processing of advanced TRIP steels.
更多查看译文
关键词
Support vector regression,Kernel-based gradient approximation,TRIP-aided martensitic steels,Hot-dip galvanization,KHMO
AI 理解论文
溯源树
样例
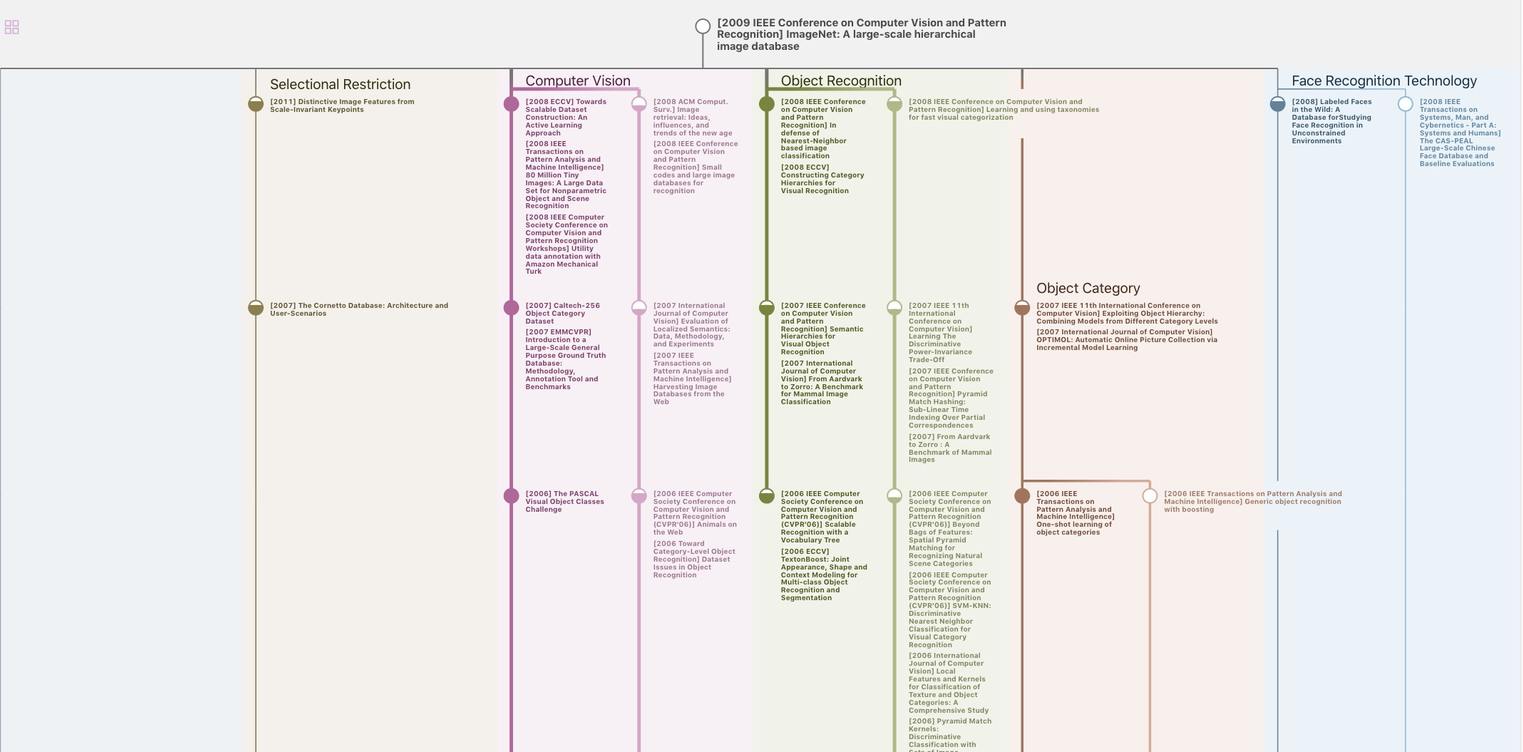
生成溯源树,研究论文发展脉络
Chat Paper
正在生成论文摘要