Trade-off Background Joint Learning for Unsupervised Vehicle Re-Identification
The visual computer/The visual computer(2023)
摘要
Existing vehicle re-identification (Re-ID) methods either extract valuable background information to enhance the robustness of the vehicle model or segment background interference information to learn vehicle fine-grained information. However, these methods do not consider the background information as a trade-off attribute to unite valuable background and background interference. This work proposes the trade-off background joint learning method for unsupervised vehicle Re-ID, which consists of two branches, to exploit the ambivalence of background information. In the global branch, a background focus of the pyramid global branch module is proposed to optimize the sample feature space. The designed pyramid background-aware attention extracts background-related features from the global image and constructs a two-fold confidence metric based on background-related and identity-related confidence scores to obtain robust clustering results during the clustering. In the local branch, a background filtering of the local branch module is proposed to alleviate the background interference. First, the background of each local region is segmented and weakened. Then, a background adaptive local label smoothing is designed to reduce noise in every local region. Comprehensive experiments on VeRi-776 and VeRi-Wild are conducted to validate the performance of the proposed balanced background information method. Experimental results show that the proposed method outperforms the state-of-the-art.
更多查看译文
关键词
Vehicle re-identification,Label smoothing,Pyramid background-aware attention,Joint learning
AI 理解论文
溯源树
样例
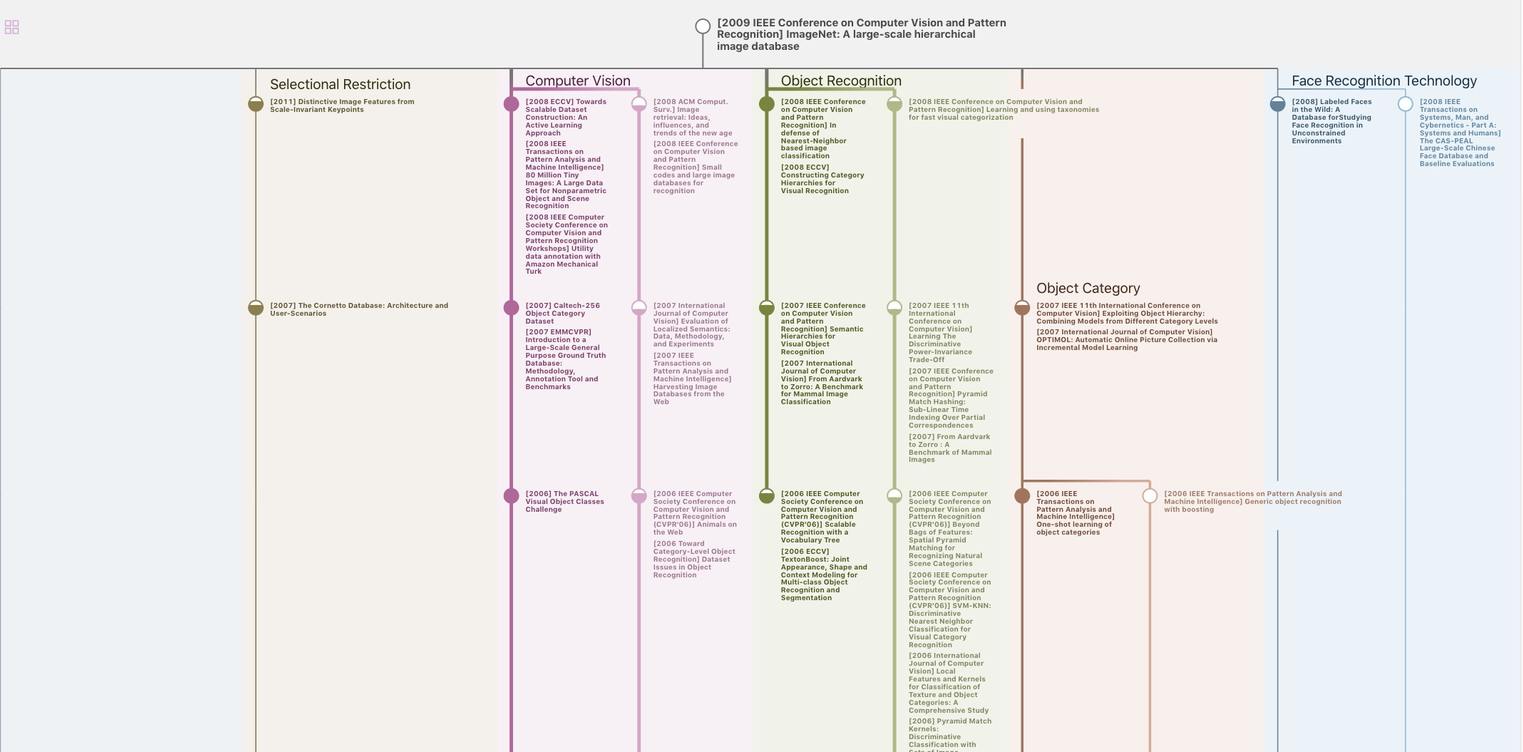
生成溯源树,研究论文发展脉络
Chat Paper
正在生成论文摘要