GSA4FDA: Deep Geometric and Statistic Alignment for Fewer Labeled Domain Adaptation
NEURAL PROCESSING LETTERS(2023)
摘要
Domain adaptation (DA) improves the generalization ability of models across source and target domains with different distributions. Current methods aim to reduce domain distribution divergence to learn transferable features. However, in most real cases, the number of available labeled samples is limited, and annotating labels requires significant time and labor costs, making it difficult to achieve high accuracy. To address this problem, we propose a novel method called Deep Geometric and Statistic Alignment for Fewer labeled Domain Adaptation (GSA4FDA). This method achieves the target task with fewer labeled source samples by combining manifold learning and leveraging the local geometric structure of sufficient unlabeled source samples. For domain alignment, we employ a joint geometric-statistical alignment and embed it into a specific layer of a deep convolutional neural network (CNN) to obtain high-level semantic information, taking into account the complementary nature of the two aspects. Specifically, we use the Nyström method and Maximum Mean Discrepancy (MMD) to compensate for the geometrical and statistical shift between domains. The experimental results on several datasets demonstrate the superiority of our method, particularly when there are limited labeled source samples available.
更多查看译文
关键词
Domain adaptation,Geometric adaptation,Nyström method,Statistical adaptation
AI 理解论文
溯源树
样例
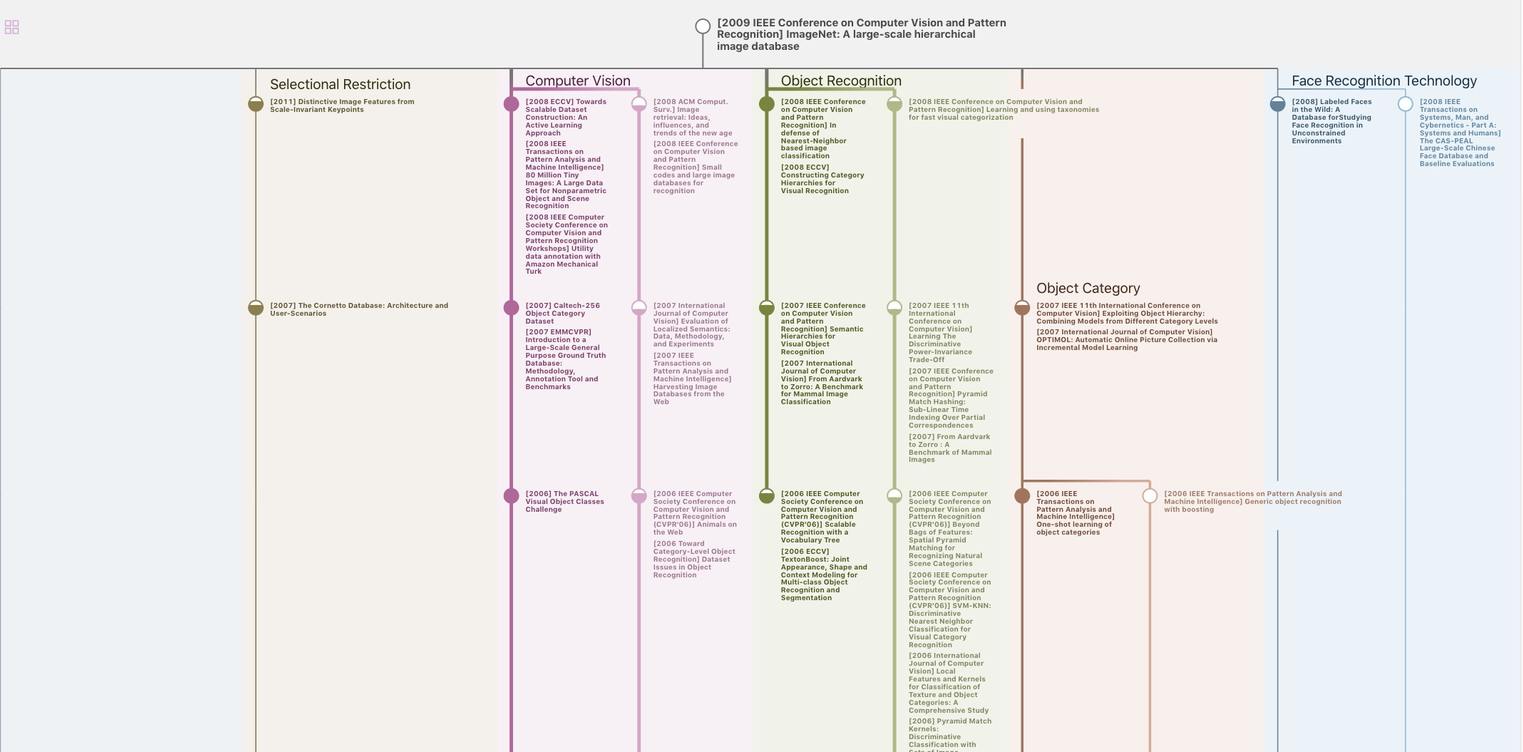
生成溯源树,研究论文发展脉络
Chat Paper
正在生成论文摘要