EABERT: An Event Annotation Enhanced BERT Framework for Event Extraction
MOBILE NETWORKS & APPLICATIONS(2023)
摘要
Event extraction(EE) is a challenging task of information extraction, which aims to extract structured event information from text. Existing methods usually achieve state-of-the-art performance based on pre-trained language models(PLMs) that exploit the semantic information of triggers and arguments. However, these methods struggle in extraction due to their lack of two intrinsic considerations: (a) the complexity of event structure; (b) the impact of exact event type on event extraction. In this paper, we propose an event annotation enhanced BERT framework, termed EABERT. Specifically,event annotations are predefined and can be considered as a complete event structure. We inject additional event knowledge into the model by incorporating event annotations into the model input. Furthermore, to incorporate appropriate event annotations into the model, we employ the bilateral-branch BERT network to train the event type classifier for better accuracy of event annotations. Experiments on the event extraction benchmark dataset (ACE 2005, MAVEN) significantly improved our proposed framework compared to previous approaches.
更多查看译文
关键词
Event extraction,Event annotation,Pre-trained language model
AI 理解论文
溯源树
样例
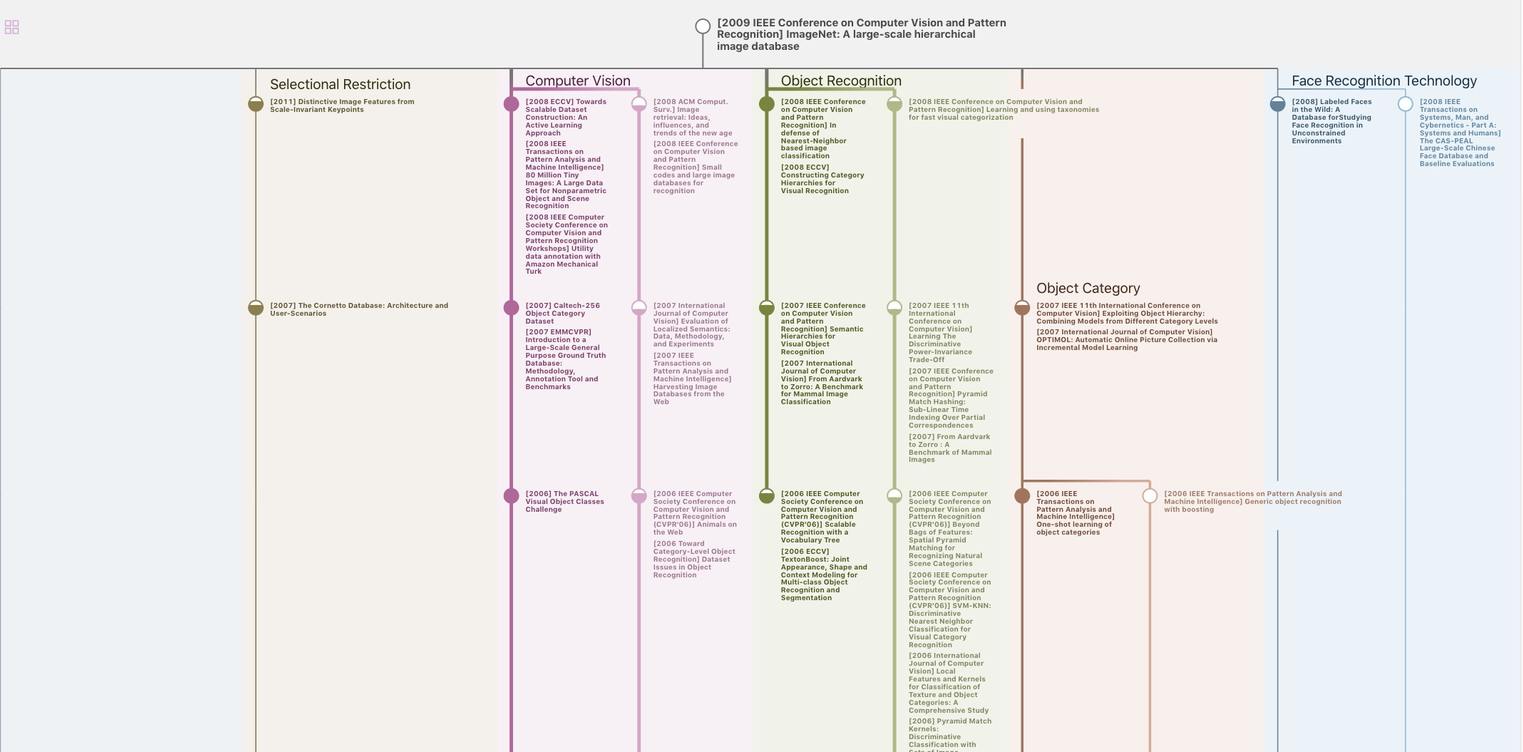
生成溯源树,研究论文发展脉络
Chat Paper
正在生成论文摘要