Multi-View Diffusion Process for Spectral Clustering and Image Retrieval
IEEE transactions on image processing : a publication of the IEEE Signal Processing Society(2023)
摘要
This paper presents a novel approach to multi-view graph learning that combines weight learning and graph learning in an alternating optimization framework. Multi-view graph learning refers to the problem of constructing a unified affinity graph using heterogeneous sources of data representation, which is a popular technique in many learning systems where no prior knowledge of data distribution is available. Our approach is based on a fusion-and-diffusion strategy, in which multiple affinity graphs are fused together via a weight learning scheme based on the unsupervised graph smoothness and utilised as a consensus prior to the diffusion. We propose a novel multi-view diffusion process that learns a manifold-aware affinity graph by propagating affinities on tensor product graphs, leveraging high-order contextual information to enhance pairwise affinities. In contrast to existing multi-view graph learning approaches, our approach is not limited by the quality of initial graphs or the assumption of a latent common subspace among multiple views. Instead, our approach is able to identify the consistency among views and fuse multiple graphs adaptively. We formulate both weight learning and diffusion-based affinity learning in a unified framework and propose an alternating optimization solver that is guaranteed to converge. The proposed approach is applied to image retrieval and clustering tasks on 16 real-world datasets. Extensive experimental results demonstrate that our approach outperforms state-of-the-art methods for both retrieval and clustering on 13 out of 16 datasets.
更多查看译文
关键词
Multi-view graph learning,diffusion process,affinity learning,retrieval,spectral clustering
AI 理解论文
溯源树
样例
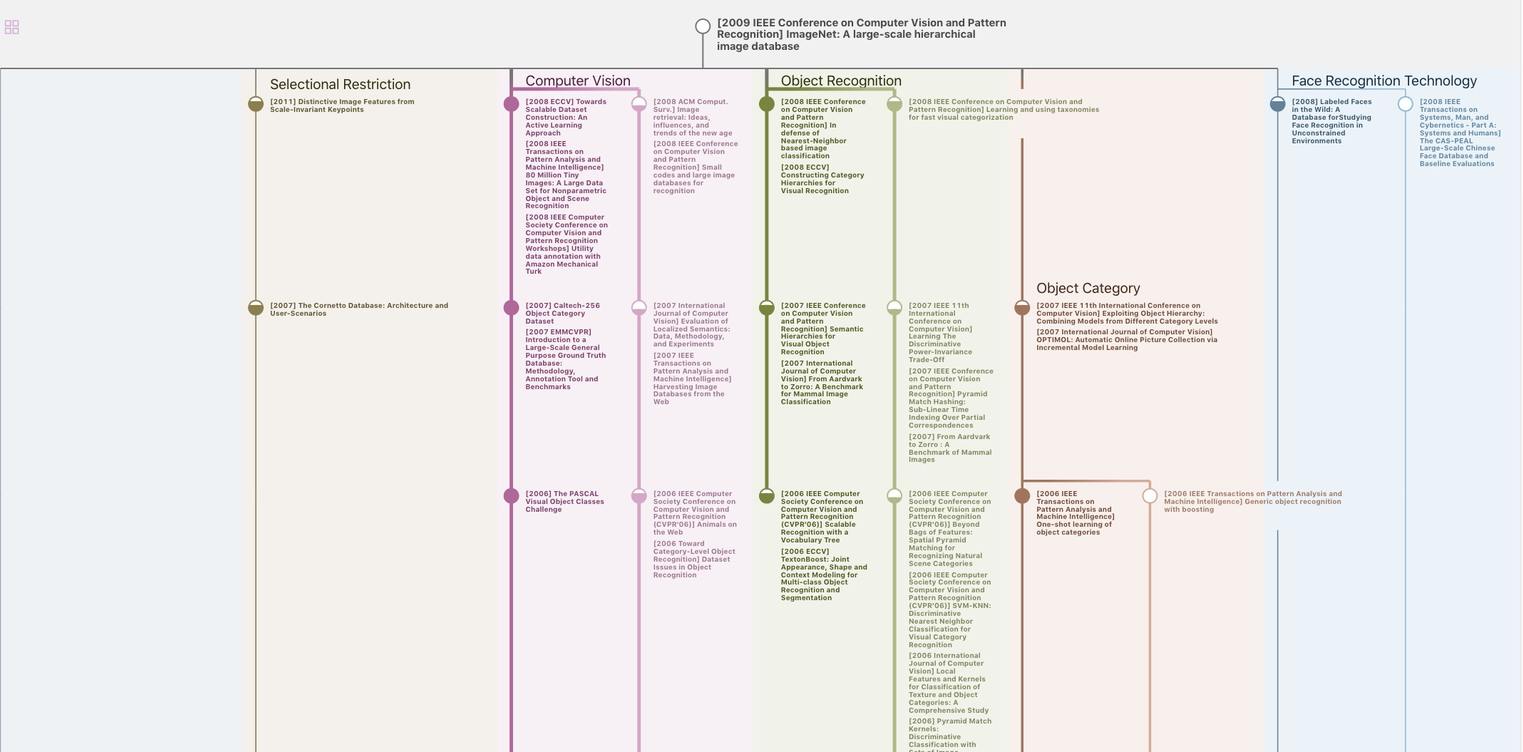
生成溯源树,研究论文发展脉络
Chat Paper
正在生成论文摘要