Natural language processing to predict isocitrate dehydrogenase genotype in diffuse glioma using MR radiology reports
European Radiology(2023)
摘要
Objectives To evaluate the performance of natural language processing (NLP) models to predict isocitrate dehydrogenase (IDH) mutation status in diffuse glioma using routine MR radiology reports. Materials and methods This retrospective, multi-center study included consecutive patients with diffuse glioma with known IDH mutation status from May 2009 to November 2021 whose initial MR radiology report was available prior to pathologic diagnosis. Five NLP models (long short-term memory [LSTM], bidirectional LSTM, bidirectional encoder representations from transformers [BERT], BERT graph convolutional network [GCN], BioBERT) were trained, and area under the receiver operating characteristic curve (AUC) was assessed to validate prediction of IDH mutation status in the internal and external validation sets. The performance of the best performing NLP model was compared with that of the human readers. Results A total of 1427 patients (mean age ± standard deviation, 54 ± 15; 779 men, 54.6%) with 720 patients in the training set, 180 patients in the internal validation set, and 527 patients in the external validation set were included. In the external validation set, BERT GCN showed the highest performance (AUC 0.85, 95% CI 0.81−0.89) in predicting IDH mutation status, which was higher than LSTM (AUC 0.77, 95% CI 0.72−0.81; p = .003) and BioBERT (AUC 0.81, 95% CI 0.76−0.85; p = .03). This was higher than that of a neuroradiologist (AUC 0.80, 95% CI 0.76−0.84; p = .005) and a neurosurgeon (AUC 0.79, 95% CI 0.76−0.84; p = .04). Conclusion BERT GCN was externally validated to predict IDH mutation status in patients with diffuse glioma using routine MR radiology reports with superior or at least comparable performance to human reader. Clinical relevance statement Natural language processing may be used to extract relevant information from routine radiology reports to predict cancer genotype and provide prognostic information that may aid in guiding treatment strategy and enabling personalized medicine. Key Points • A transformer-based natural language processing (NLP) model predicted isocitrate dehydrogenase mutation status in diffuse glioma with an AUC of 0.85 in the external validation set. • The best NLP models were superior or at least comparable to human readers in both internal and external validation sets. • Transformer-based models showed higher performance than conventional NLP model such as long short-term memory.
更多查看译文
关键词
Natural language processing,Isocitrate dehydrogenase,Glioma
AI 理解论文
溯源树
样例
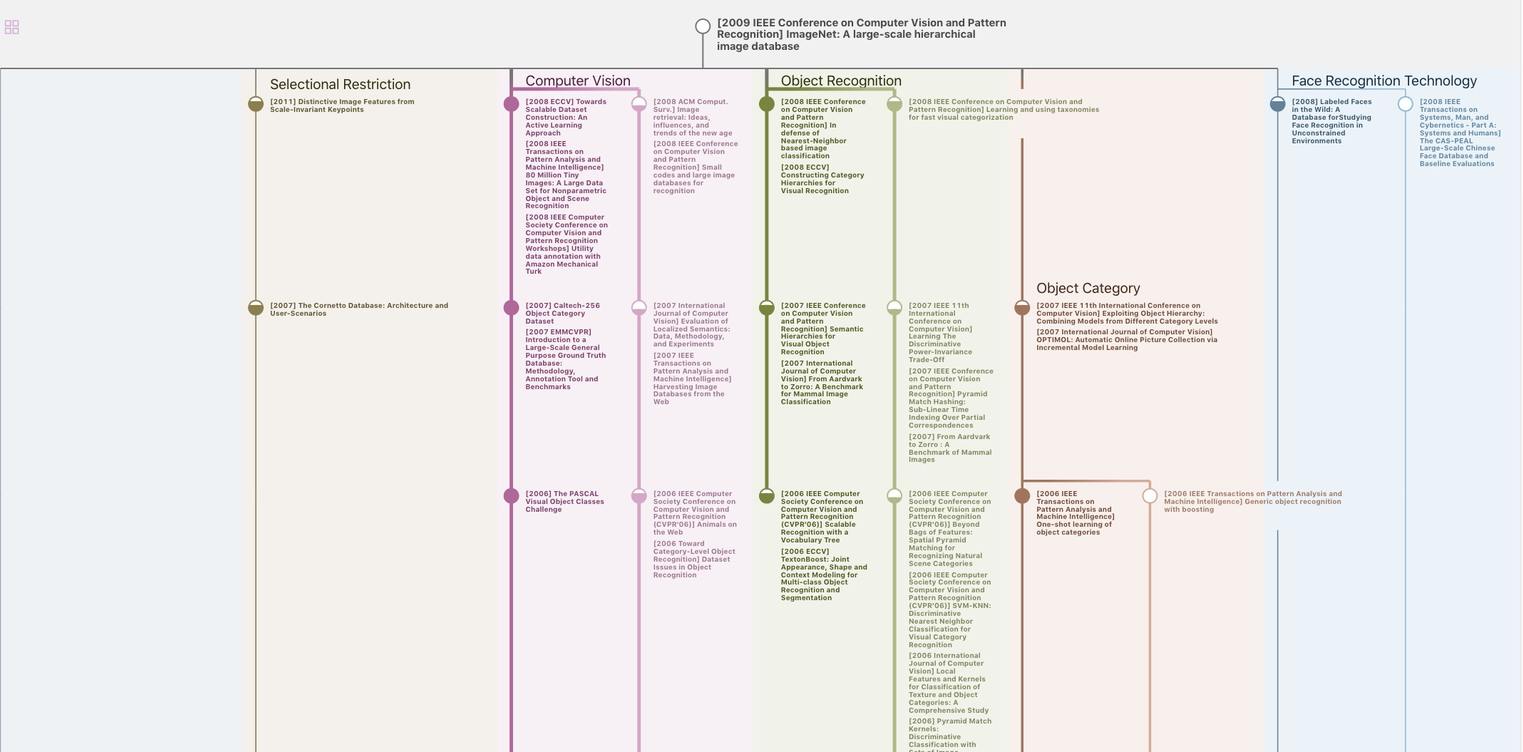
生成溯源树,研究论文发展脉络
Chat Paper
正在生成论文摘要