Federated Learning With Over-the-Air Aggregation Over Time-Varying Channels
IEEE Transactions on Wireless Communications(2023)
摘要
We study federated learning (FL) with over-the-air aggregation over time-varying wireless channels. Independent workers compute local gradients based on their local datasets and send them to a parameter server (PS) through a time-varying multipath fading multiple access channel via orthogonal frequency-division multiplexing (OFDM). We assume that the workers do not have channel state information, hence the PS employs multiple antennas to alleviate the fading effects. Wireless channel variations result in inter-carrier interference, which has a detrimental effect on the performance of OFDM systems, especially when the channel is rapidly varying. We examine the effects of the channel time variations on the convergence of the FL with over-the-air aggregation, and show that the resulting undesired interference terms have only limited destructive effects, which do not prevent the convergence of the learning algorithm. We also validate our results via extensive simulations, which corroborate the theoretical expectations.
更多查看译文
关键词
Federated learning, stochastic gradient descent, time-varying multipath fading MAC, OFDM, doppler spread
AI 理解论文
溯源树
样例
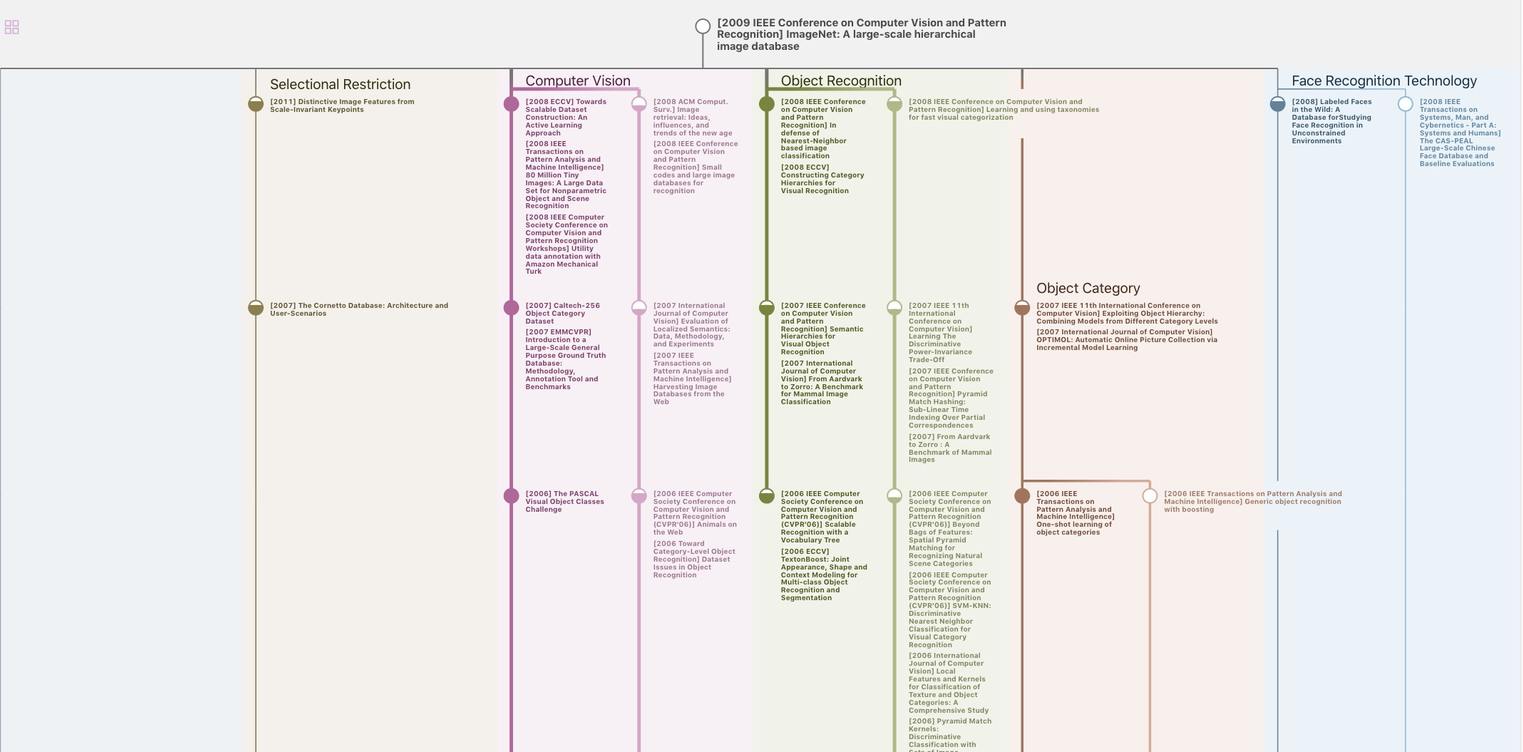
生成溯源树,研究论文发展脉络
Chat Paper
正在生成论文摘要