Machine-Learning Algorithms for Process Condition Data-Based Inclusion Prediction in Continuous-Casting Process: A Case Study.
Sensors (Basel, Switzerland)(2023)
摘要
Quality-related prediction in the continuous-casting process is important for the quality and process control of casting slabs. As intelligent manufacturing technologies continue to evolve, numerous data-driven techniques have been available for industrial applications. This case study was aimed at developing a machine-learning algorithm, capable of predicting slag inclusion defects in continuous-casting slabs, based on process condition sensor data. A large dataset consisting of sensor data from nearly 7300 casting samples has been analyzed, with the empirical mode decomposition (EMD) algorithm utilized to process the multi-modal time series. The following machine-learning algorithms have been examined: K-Nearest neighbors, support vector classifier (linear and nonlinear kernels), decision trees, random forests, AdaBoost, and Artificial Neural Networks. Four over-sampling or under-sampling algorithms have been adopted to solve imbalanced data distribution. In the experiment, the optimized random forest outperformed other machine-learning algorithms in terms of recall and ROC AUC, which could provide valuable insights for quality control.
更多查看译文
关键词
machine learning, continuous casting, inclusions, imbalanced dataset, case study, quality prediction
AI 理解论文
溯源树
样例
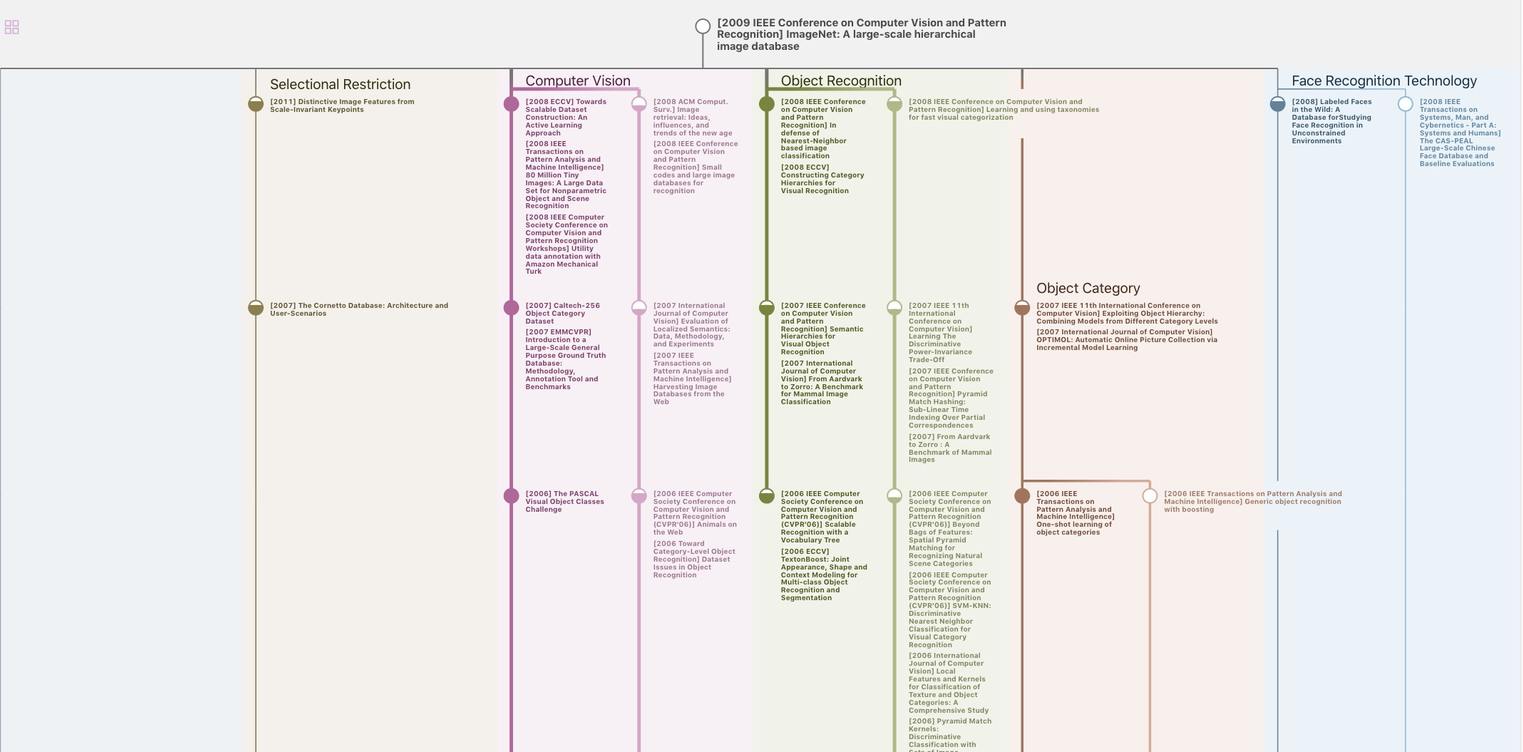
生成溯源树,研究论文发展脉络
Chat Paper
正在生成论文摘要