Unleashing the Power of Extra-Tree Feature Selection and Random Forest Classifier for Improved Survival Prediction in Heart Failure Patients
CoRR(2023)
摘要
Heart failure is a life-threatening condition that affects millions of people worldwide. The ability to accurately predict patient survival can aid in early intervention and improve patient outcomes. In this study, we explore the potential of utilizing data pre-processing techniques and the Extra-Tree (ET) feature selection method in conjunction with the Random Forest (RF) classifier to improve survival prediction in heart failure patients. By leveraging the strengths of ET feature selection, we aim to identify the most significant predictors associated with heart failure survival. Using the public UCL Heart failure (HF) survival dataset, we employ the ET feature selection algorithm to identify the most informative features. These features are then used as input for grid search of RF. Finally, the tuned RF Model was trained and evaluated using different matrices. The approach was achieved 98.33% accuracy that is the highest over the exiting work.
更多查看译文
关键词
improved survival prediction,random forest
AI 理解论文
溯源树
样例
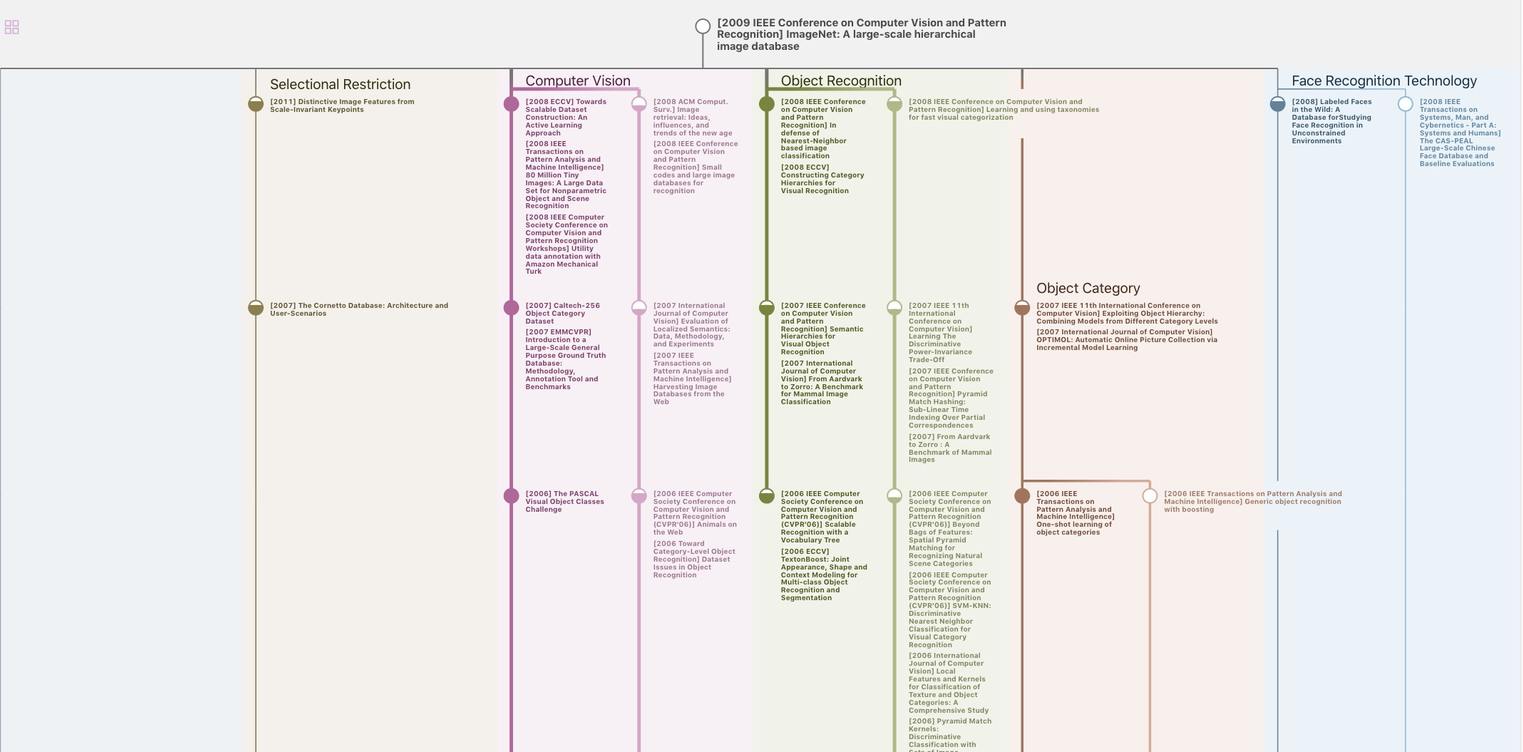
生成溯源树,研究论文发展脉络
Chat Paper
正在生成论文摘要