Computer-Aided Cytology Diagnosis in Animals: CNN-Based Image Quality Assessment for Accurate Disease Classification
CoRR(2023)
摘要
This paper presents a computer-aided cytology diagnosis system designed for animals, focusing on image quality assessment (IQA) using Convolutional Neural Networks (CNNs). The system's building blocks are tailored to seamlessly integrate IQA, ensuring reliable performance in disease classification. We extensively investigate the CNN's ability to handle various image variations and scenarios, analyzing the impact on detecting low-quality input data. Additionally, the network's capacity to differentiate valid cellular samples from those with artifacts is evaluated. Our study employs a ResNet18 network architecture and explores the effects of input sizes and cropping strategies on model performance. The research sheds light on the significance of CNN-based IQA in computer-aided cytology diagnosis for animals, enhancing the accuracy of disease classification.
更多查看译文
关键词
image quality
AI 理解论文
溯源树
样例
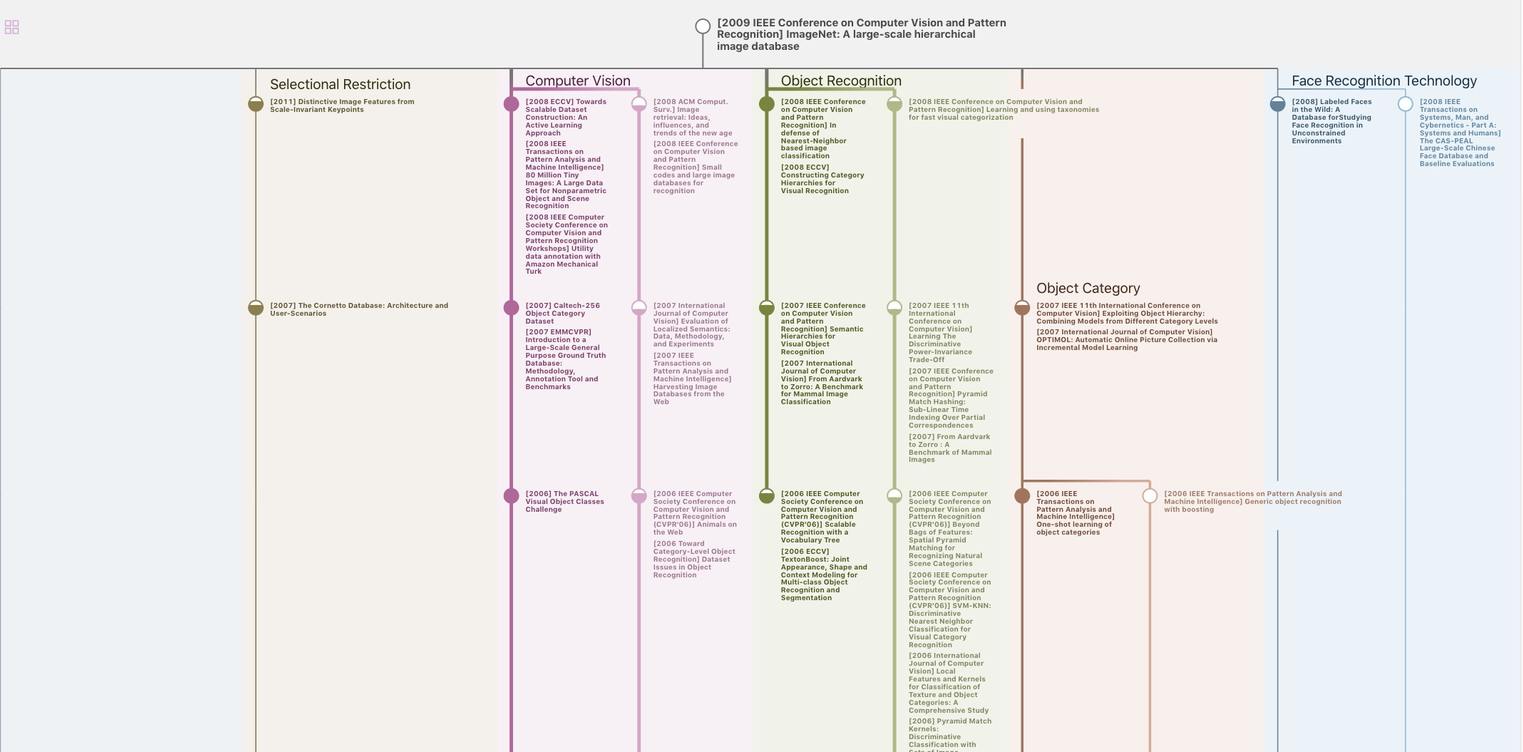
生成溯源树,研究论文发展脉络
Chat Paper
正在生成论文摘要