The Stochastic Steepest Descent Method for Robust Optimization in Banach Spaces
CoRR(2023)
摘要
Stochastic gradient methods have been a popular and powerful choice of optimization methods, aimed at minimizing functions. Their advantage lies in the fact that that one approximates the gradient as opposed to using the full Jacobian matrix. One research direction, related to this, has been on the application to infinite-dimensional problems, where one may naturally have a Hilbert space framework. However, there has been limited work done on considering this in a more general setup, such as where the natural framework is that of a Banach space. This article aims to address this by the introduction of a novel stochastic method, the stochastic steepest descent method (SSD). The SSD will follow the spirit of stochastic gradient descent, which utilizes Riesz representation to identify gradients and derivatives. Our choice for using such a method is that it naturally allows one to adopt a Banach space setting, for which recent applications have exploited the benefit of this, such as in PDE-constrained shape optimization. We provide a convergence theory related to this under mild assumptions. Furthermore, we demonstrate the performance of this method on a couple of numerical applications, namely a $p$-Laplacian and an optimal control problem. Our assumptions are verified in these applications.
更多查看译文
关键词
stochastic steepest descent method,robust optimization,banach
AI 理解论文
溯源树
样例
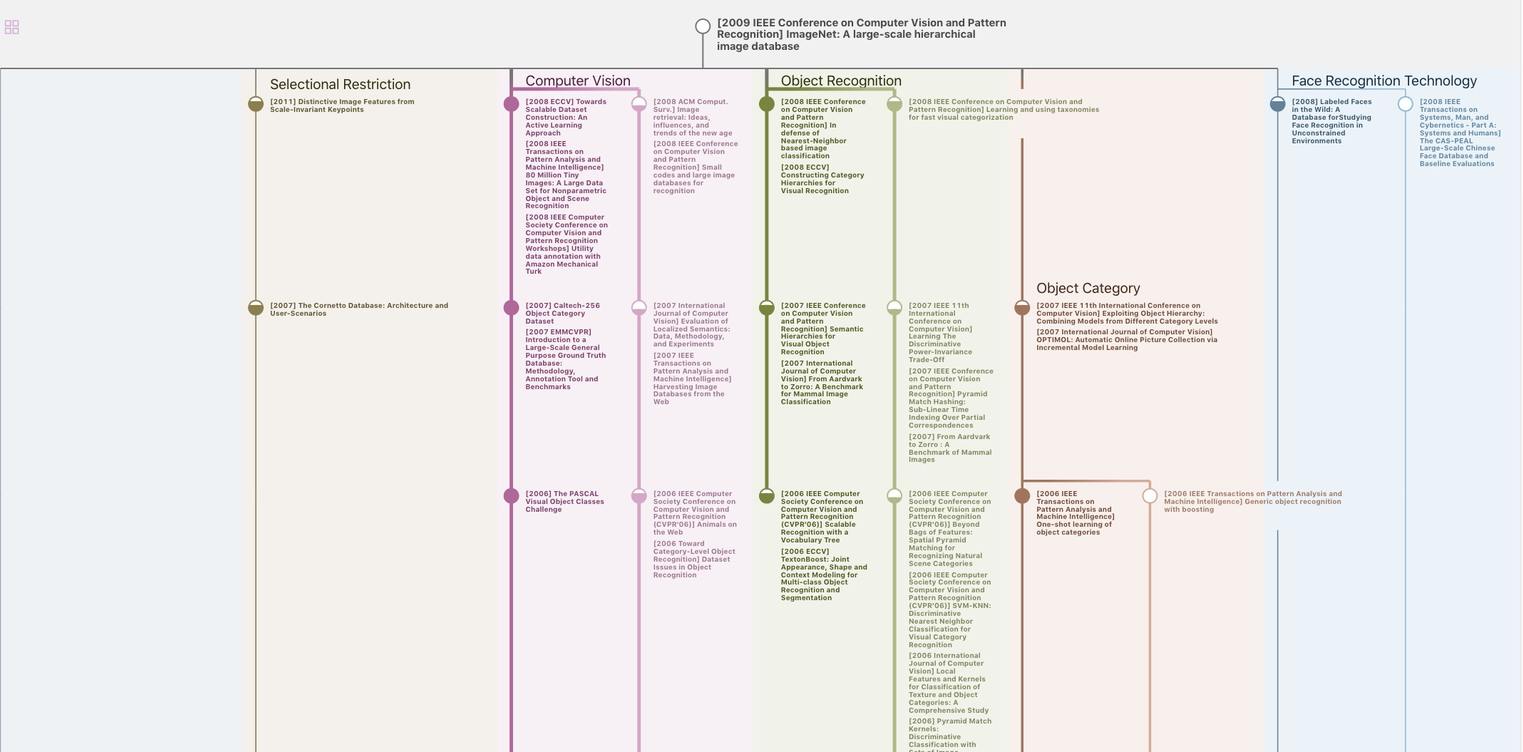
生成溯源树,研究论文发展脉络
Chat Paper
正在生成论文摘要