Domain Adaptation for Learning from Label Proportions Using Domain-Adversarial Neural Network
SN Comput. Sci.(2023)
摘要
Learning from Label Proportions (LLP) is a machine learning problem where the training data are composed of bags of instances, and only the class label proportions for each bag are given. In some domains, we can directly obtain label distributions; for example, one can use census statistics and social media user information grouped by location to build a classifier for user demographics. However, label proportions are unavailable in many domains, such as product review sites. The solution is to modify the model fit on data from where label proportion are available domains (the source domain) to apply to a domain where the label distributions are not available (target domain). Such problems can be regarded as the unsupervised domain adaptation problems in an LLP setting. The goal of this paper is to introduce domain adaptation methods to the original LLP solutions such that the proposed model can classify instances from a new domain. We propose a model combining domain-adversarial neural network (DANN) and label regularization, which can be fit on the source-domain bags and predict labels for target-domain instances. This approach requires only label proportions in the source domain. Our experiments on both synthetic tasks and sentiment classification tasks indicate a noticeable improvement in accuracy as compared to using LLP without domain adaptation.
更多查看译文
关键词
Domain adaptation,Learning from label proportion,Domain-adversarial neural network
AI 理解论文
溯源树
样例
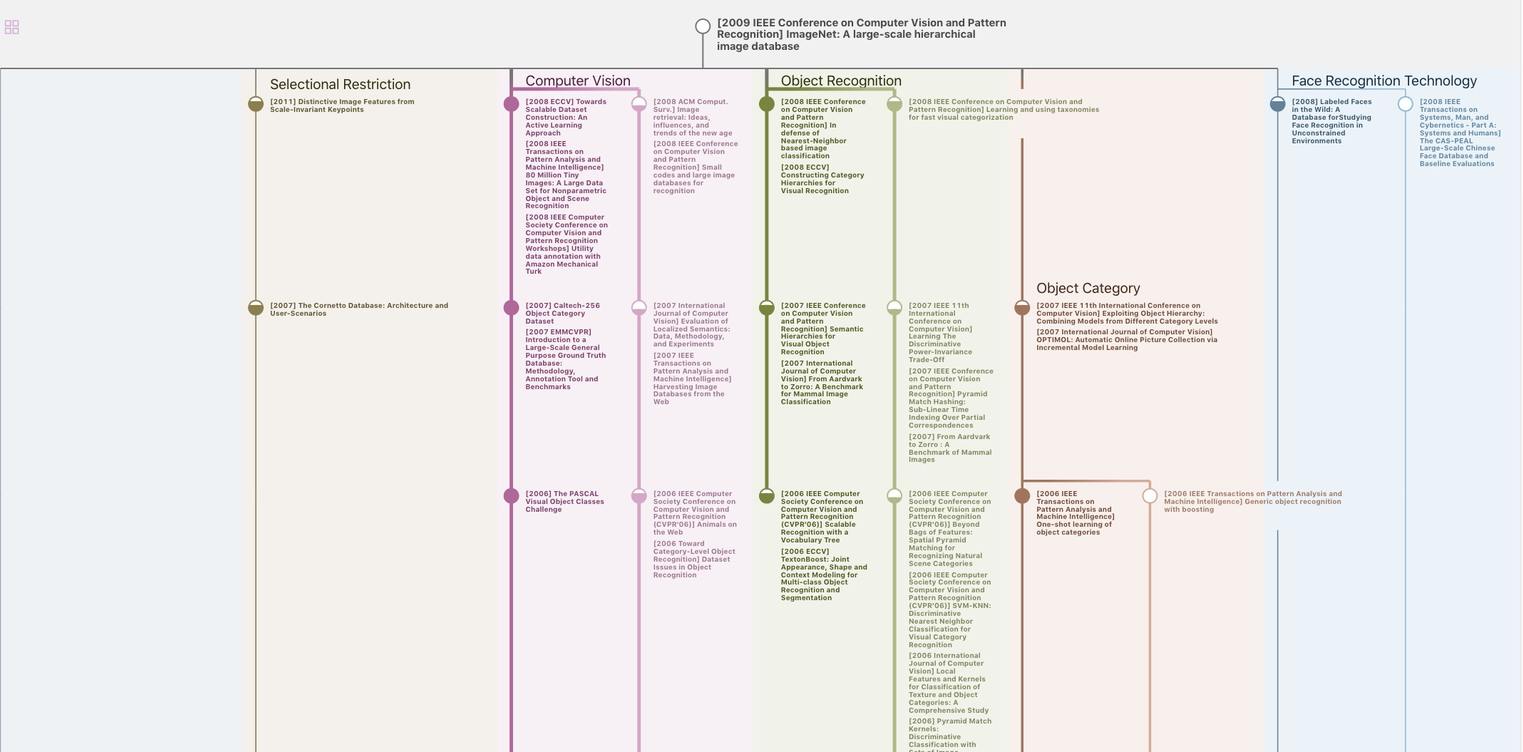
生成溯源树,研究论文发展脉络
Chat Paper
正在生成论文摘要