Shedding Light on Darkness: Enhancing Object Detection Robustness with Synthetic Perturbations for Real-world Challenges
2023 IEEE Conference on Artificial Intelligence (CAI)(2023)
摘要
Robustness against distribution shifts is crucial for object detection models in real-world applications. In this study, we evaluate the performance of four state-of-the-art models against natural perturbations, retrain them with synthetic perturbations using the AugLy augmentation package, and assess their improved performance against natural perturbations. Our empirical ablation study focuses on the brightness perturbation modality using the COCO 2017 and ExDARK datasets. Our findings suggest that synthetic perturbations can effectively improve model robustness against real-world distribution shifts, providing valuable insights for deploying robust object detection models in real-world scenarios.
更多查看译文
关键词
object detection, synthetic perturbation, natural perturbation, deep neural network model, AugLy augmentation, robustness, distribution shifts, data augmentation, transfer learning, ablation study, real-world scenarios
AI 理解论文
溯源树
样例
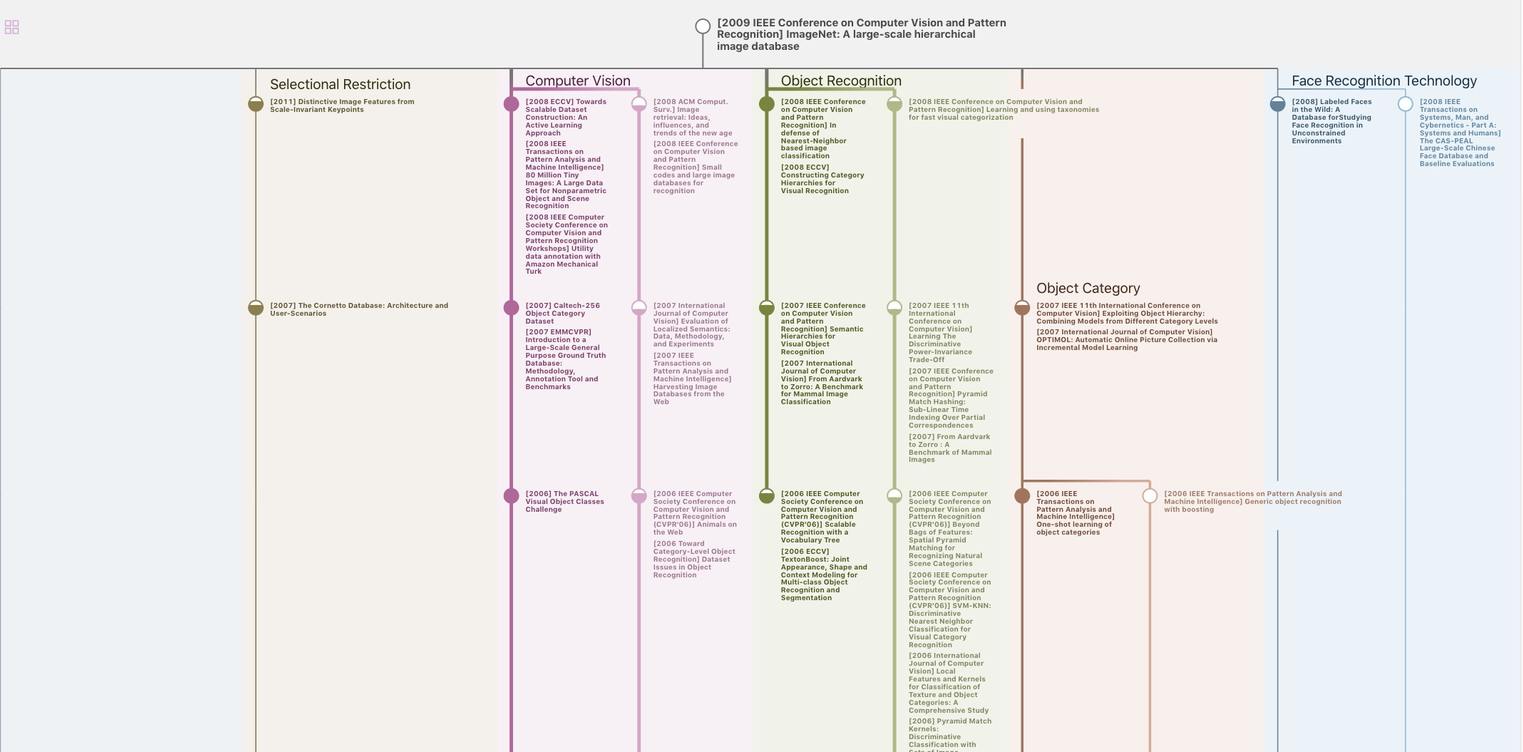
生成溯源树,研究论文发展脉络
Chat Paper
正在生成论文摘要