Post -Stroke Virtual Assessment Using Deep Learning
2023 IEEE Conference on Artificial Intelligence (CAI)(2023)
Abstract
Various machine learning (ML) models, including Linear SVM, SVM with RBF, and KNN, have been adopted to classify affected vs. non -affected body parts post-stroke. However, the quality and accuracy of each model depend on the data shape, class distribution, and configurations. Deep learning (DL) models such as CNN and LSTIVI have shown promising classification results compared to traditional machine learning. However, obtaining a robust training process is prominent. In this paper, we propose robust post -stroke assessment models adopting the methodology of ensemble and hybrid learning. Using a dataset derived from wearable sensors called Xsens sensors collected from twenty stroke survivors, we compared the performance of DL based and the proposed models to detect the affected hand of the stroke survivors from non -affected hands. Experimental results show the ensemble hybrid DL -based model achieved the highest accuracy compared to the individual DL models.
MoreTranslated text
Key words
Keywords Stroke,Wearable Sensors,Machine Learning,Deep Learning,Hybrid Modeling,Ensemble Learning
AI Read Science
Must-Reading Tree
Example
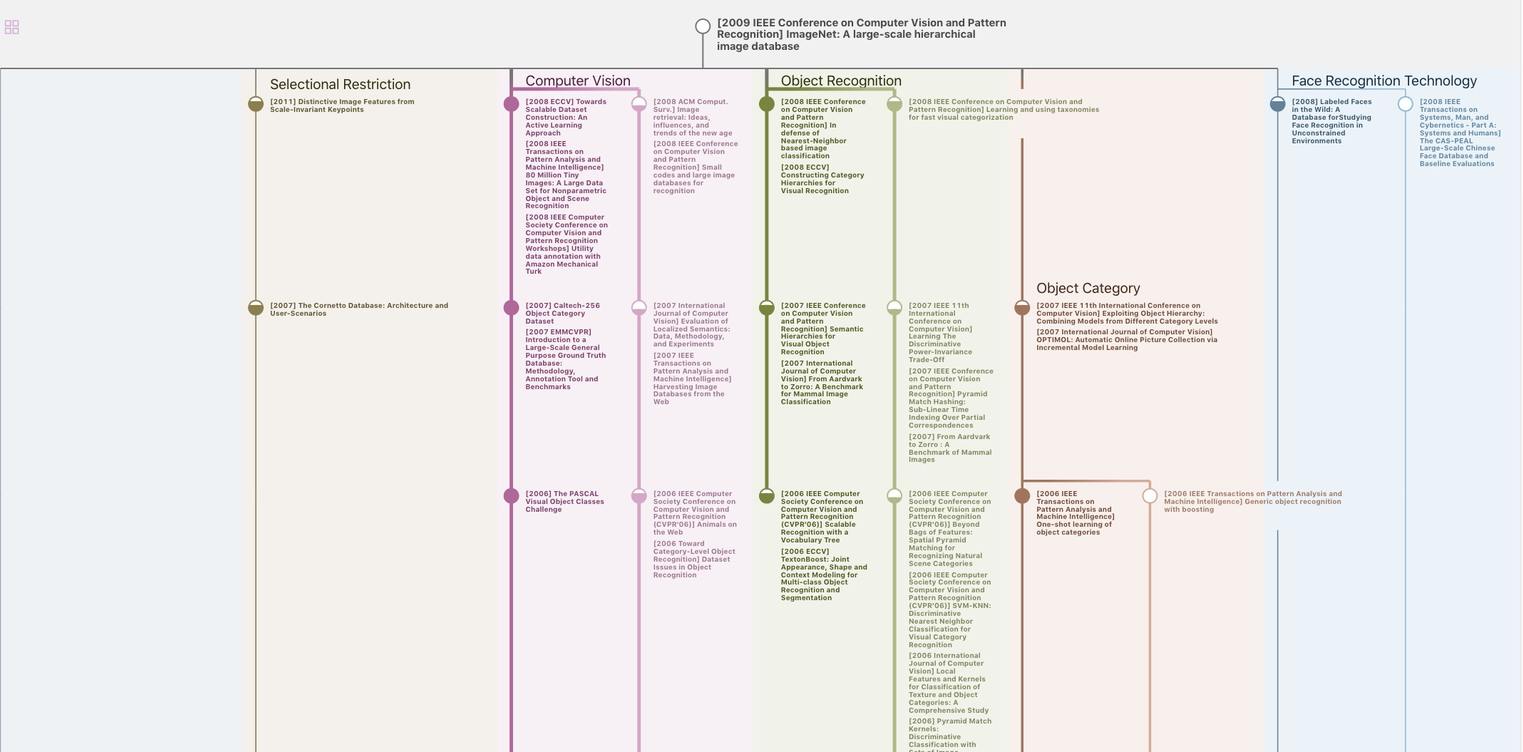
Generate MRT to find the research sequence of this paper
Chat Paper
Summary is being generated by the instructions you defined