Locally Adaptive and Differentiable Regression
CoRR(2023)
摘要
Over-parameterized models like deep nets and random forests have become very popular in machine learning. However, the natural goals of continuity and differentiability, common in regression models, are now often ignored in modern overparametrized, locally-adaptive models. We propose a general framework to construct a global continuous and differentiable model based on a weighted average of locally learned models in corresponding local regions. This model is competitive in dealing with data with different densities or scales of function values in different local regions. We demonstrate that when we mix kernel ridge and polynomial regression terms in the local models, and stitch them together continuously, we achieve faster statistical convergence in theory and improved performance in various practical settings.
更多查看译文
关键词
adaptive
AI 理解论文
溯源树
样例
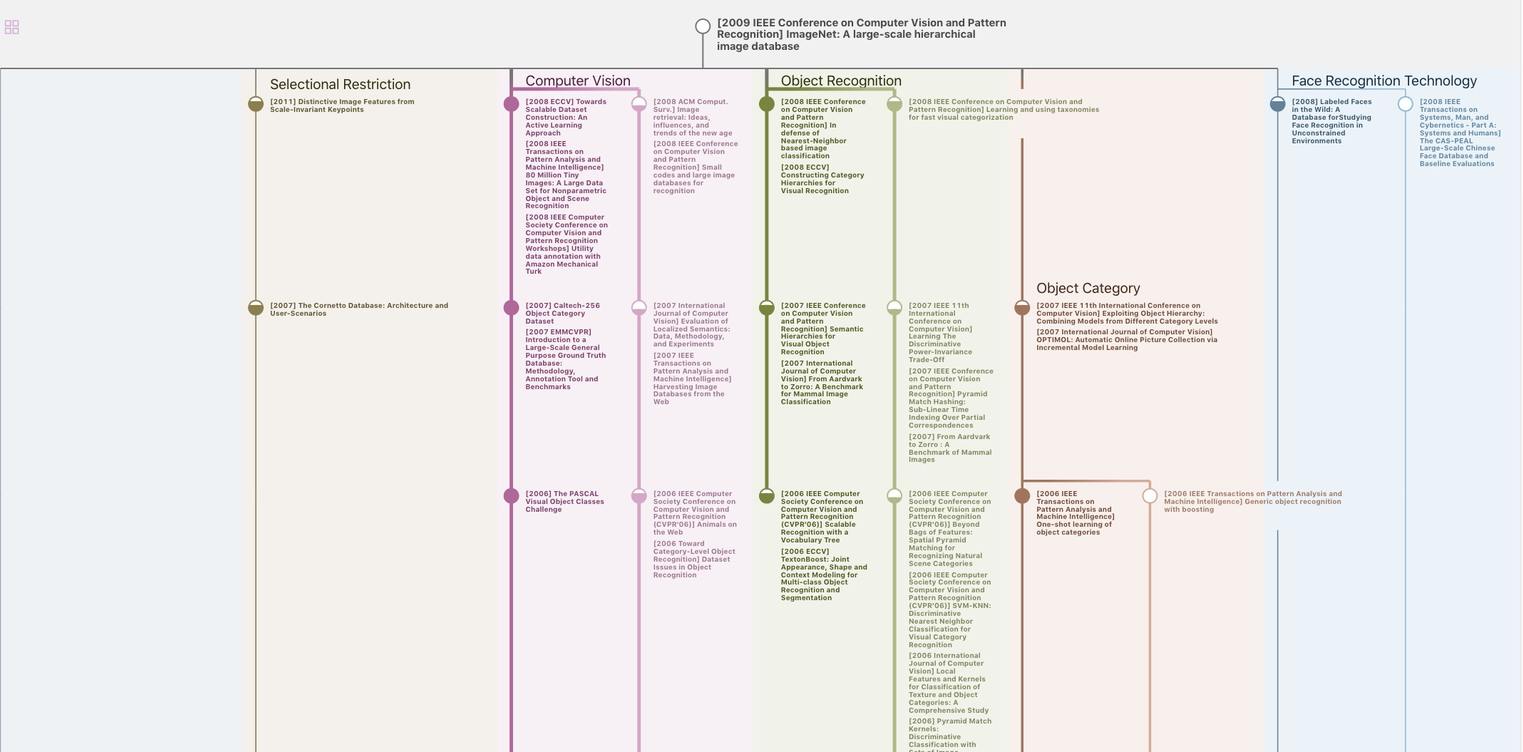
生成溯源树,研究论文发展脉络
Chat Paper
正在生成论文摘要