OCDaf: Ordered Causal Discovery with Autoregressive Flows
CoRR(2023)
Abstract
We propose OCDaf, a novel order-based method for learning causal graphs from observational data. We establish the identifiability of causal graphs within multivariate heteroscedastic noise models, a generalization of additive noise models that allow for non-constant noise variances. Drawing upon the structural similarities between these models and affine autoregressive normalizing flows, we introduce a continuous search algorithm to find causal structures. Our experiments demonstrate state-of-the-art performance across the Sachs and SynTReN benchmarks in Structural Hamming Distance (SHD) and Structural Intervention Distance (SID). Furthermore, we validate our identifiability theory across various parametric and nonparametric synthetic datasets and showcase superior performance compared to existing baselines.
MoreTranslated text
Key words
causal discovery,autoregressive flows
AI Read Science
Must-Reading Tree
Example
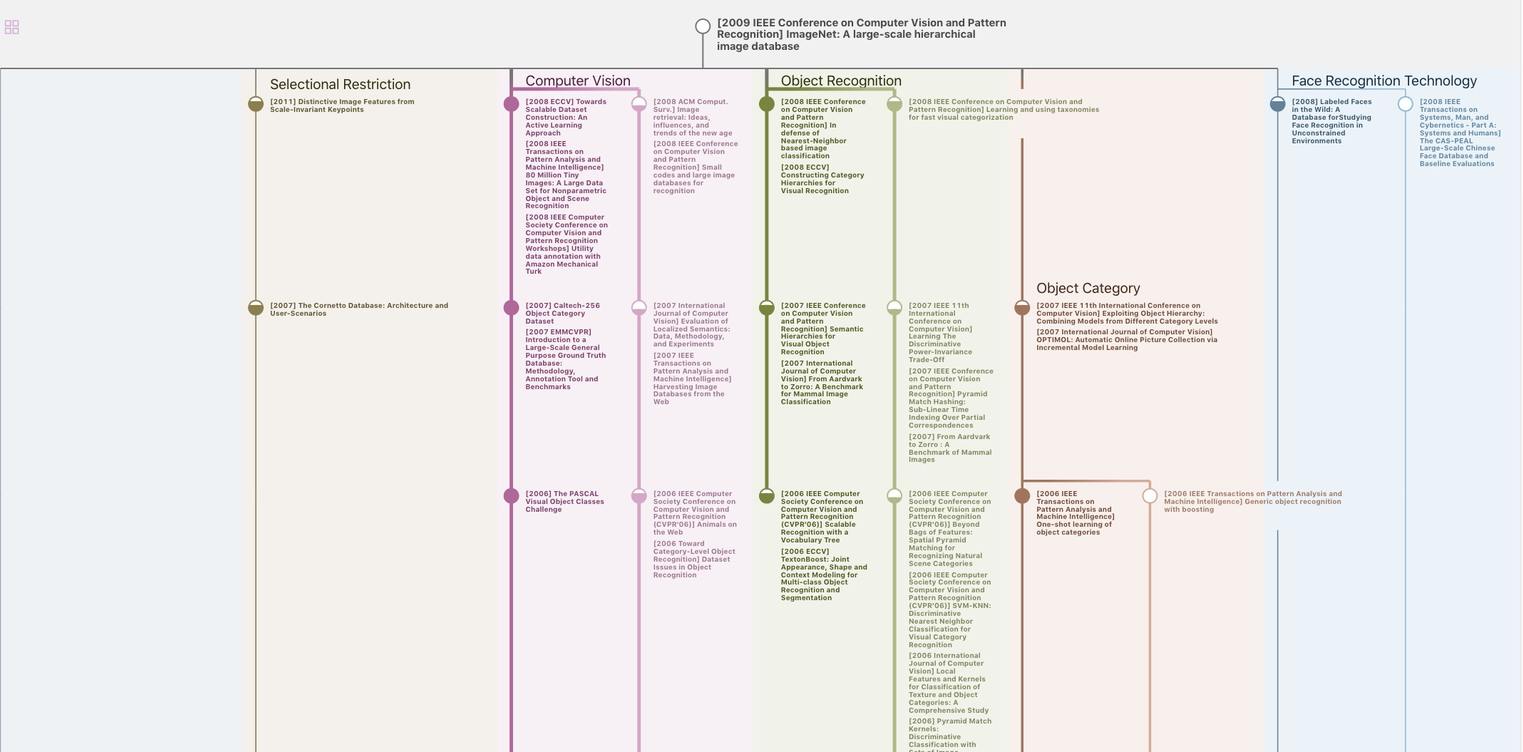
Generate MRT to find the research sequence of this paper
Chat Paper
Summary is being generated by the instructions you defined