Distilling Knowledge from Resource Management Algorithms to Neural Networks: A Unified Training Assistance Approach
2023 IEEE 98TH VEHICULAR TECHNOLOGY CONFERENCE, VTC2023-FALL(2023)
摘要
As a fundamental problem, many studies are dedicated to the optimization of signal-to-interference-plus-noise ratio (SINR), in a multi-user setting. Although traditional model-based optimization methods achieve strong performance, it has high complexity. To fully leverage the high performance of traditional methods and the low complexity of the neural network (NN) based method, a knowledge distillation (KD) based algorithm distillation (AD) method is proposed in this paper, where traditional optimization methods serve as "teachers" for NN "students", improving unsupervised and reinforcement learning. This approach tackles common issues: unattainable optimal labels, overfitting, and inefficient training. Simulations confirm the advantages of AD, paving the way for traditional optimization integration with NNs in wireless communication.
更多查看译文
关键词
signal-to-interference-plus-noise ratio,neural network,algorithm distillation,convergence speed
AI 理解论文
溯源树
样例
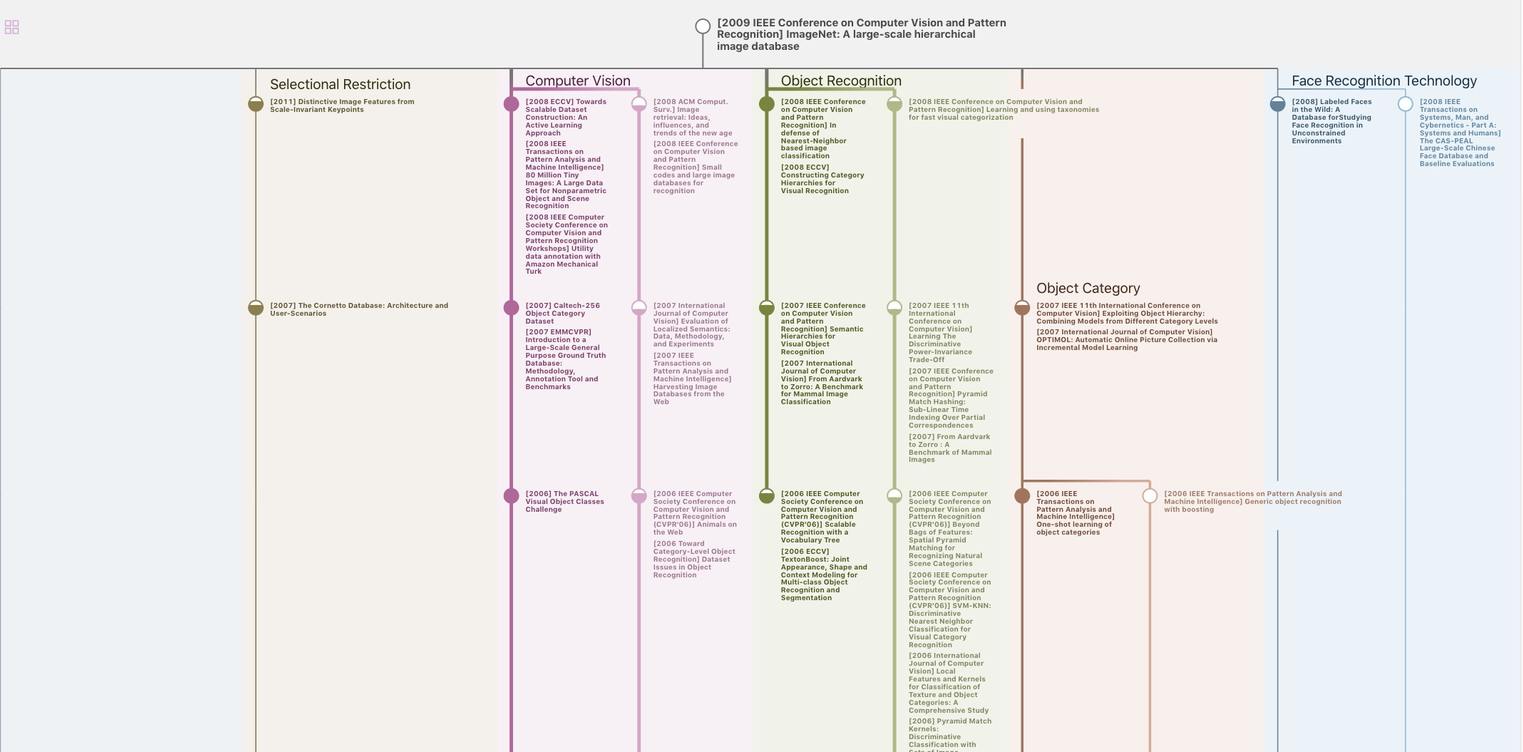
生成溯源树,研究论文发展脉络
Chat Paper
正在生成论文摘要